A Scalable Near-Memory Architecture for Training Deep Neural Networks on Large In-Memory Datasets.
IEEE Transactions on Computers(2019)
摘要
Most investigations into near-memory hardware accelerators for deep neural networks have primarily focused on inference, while the potential of accelerating training has received relatively little attention so far. Based on an in-depth analysis of the key computational patterns in state-of-the-art gradient-based training methods, we propose an efficient near-memory acceleration engine called NTX that can be used to train state-of-the-art deep convolutional neural networks at scale. Our main contributions are: (i) a loose coupling of RISC-V cores and NTX co-processors reducing offloading overhead by
$7\times$ 7 ×
over previously published results; (ii) an optimized IEEE 754 compliant data path for fast high-precision convolutions and gradient propagation; (iii) evaluation of near-memory computing with NTX embedded into residual area on the Logic Base die of a Hybrid Memory Cube; and (iv) a scaling analysis to meshes of HMCs in a data center scenario. We demonstrate a
$2.7\times$ 2 . 7 ×
energy efficiency improvement of NTX over contemporary GPUs at
$4.4\times$ 4 . 4 ×
less silicon area, and a compute performance of 1.2 Tflop/s for training large state-of-the-art networks with full floating-point precision. At the data center scale, a mesh of NTX achieves above 95 percent parallel and energy efficiency, while providing
$2.1\times$ 2 . 1 ×
energy savings or
$3.1\times$ 3 . 1 ×
performance improvement over a GPU-based system.
更多查看译文
关键词
Training,Hardware,Computer architecture,Neural networks,Registers,Data centers,Silicon
AI 理解论文
溯源树
样例
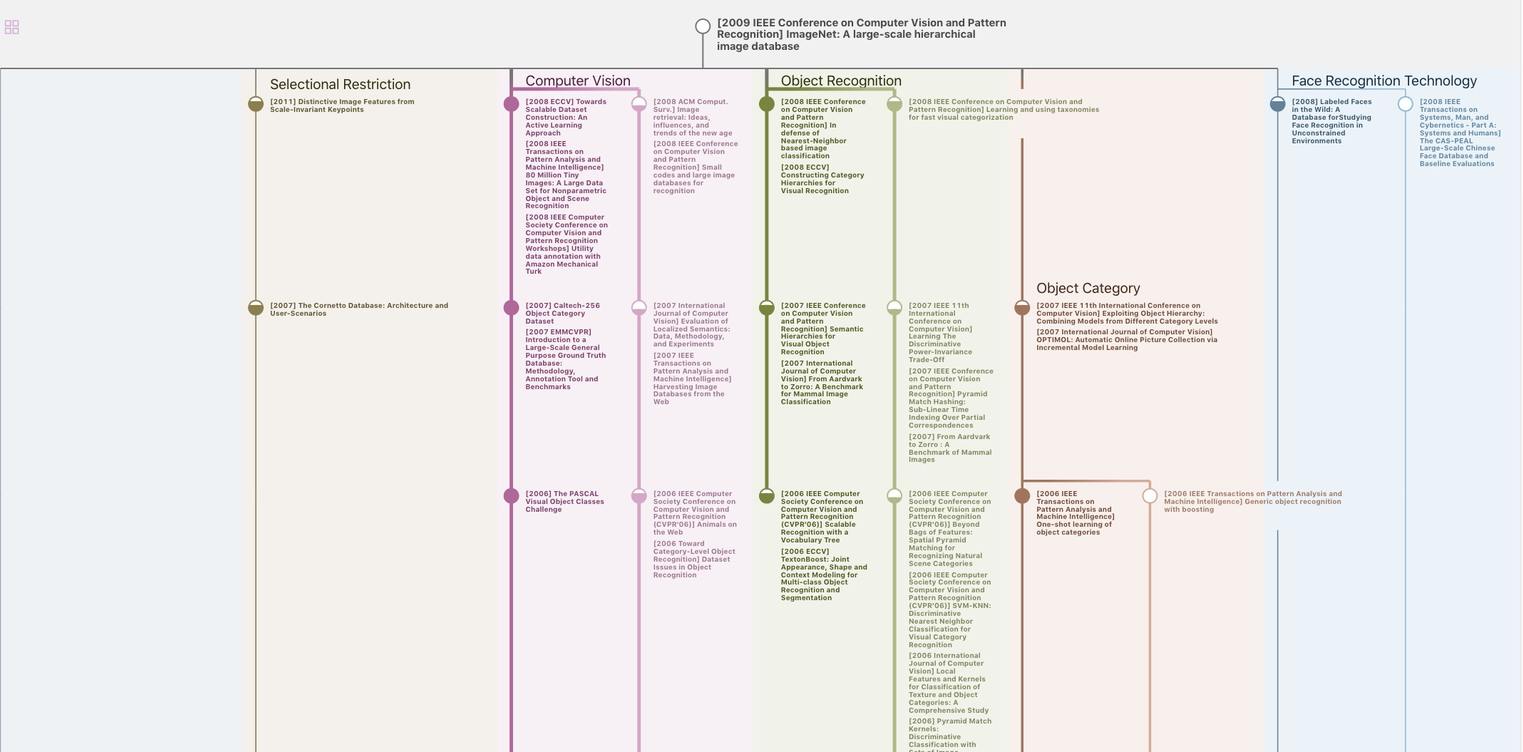
生成溯源树,研究论文发展脉络
Chat Paper
正在生成论文摘要