Opening the Black Box: Analysing MLP Functionality Using Walsh Functions
Studies in Computational Intelligence(2016)
摘要
The Multilayer Perceptron (MLP) is a neural network architecture that is widely used for regression, classification and time series forecasting. One often cited disadvantage of the MLP, however, is the difficulty associated with human understanding of a particular MLP's function. This so called black box limitation is due to the fact that the weights of the network reveal little about structure of the function they implement. This paper proposes a method for understanding the structure of the function learned by MLPs that model functions of the class f : {-1, 1}(n) -> R-m. This includes regression and classification models. A Walsh decomposition of the function implemented by a trained MLP is performed and the coefficients analysed. The advantage of a Walsh decomposition is that it explicitly separates the contribution to the function made by each subset of input neurons. It also allows networks to be compared in terms of their structure and complexity. The method is demonstrated on some small toy functions and on the larger problem of the MNIST handwritten digit classification data set.
更多查看译文
关键词
Multilayer perceptrons,Walsh functions,Network function analysis
AI 理解论文
溯源树
样例
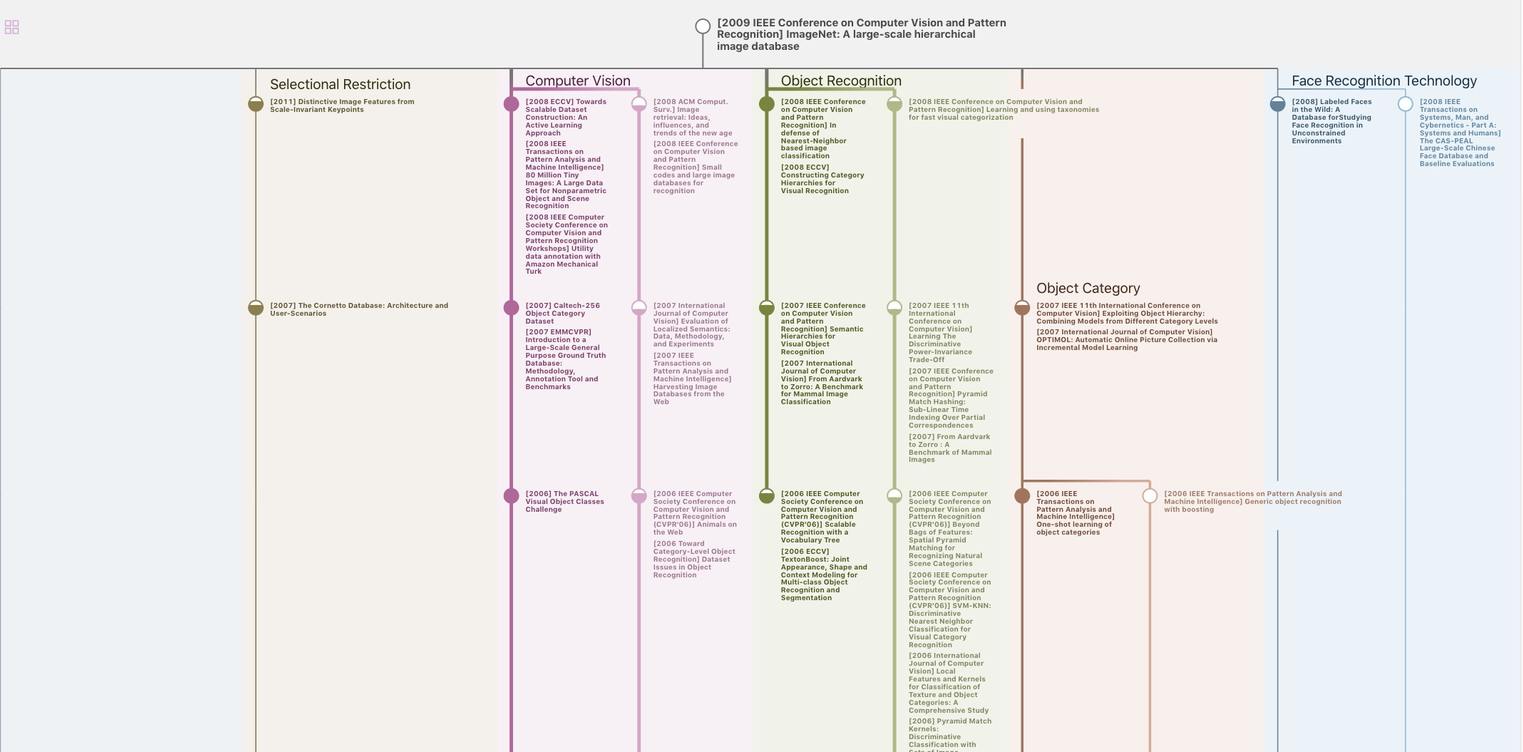
生成溯源树,研究论文发展脉络
Chat Paper
正在生成论文摘要