A3embed: Attribute Association Aware Network Embedding.
WWW '18: The Web Conference 2018 Lyon France April, 2018(2018)
摘要
Network embedding aims to learn low-dimensional vector representations for nodes in a network that preserve structural characteristics. It has been shown that such representations are helpful in several graph mining tasks such as node classification, link prediction, and community detection. Some recent works have attempted to extend the approach to attributed networks in which each node is associated with a set of attribute values. They have focused on homophily relationships by forcing nodes with similar attribute values to obtain similar vector representations. This is unnecessarily restrictive and misses the opportunity to harness other types of relationships revealed by patterns in attribute values of connected nodes for learning insightful relationships. In this paper, we propose a new network attributed embedding framework called A3embed that is aware of attribute associations. A3embed favors significant attribute associations, not merely homophily relationships, which contributes to its robustness to diverse attribute vectors and noisy links. The experimental results on real-world datasets demonstrate that the proposed framework achieves better performance on different graph mining tasks compared to existing models.
更多查看译文
AI 理解论文
溯源树
样例
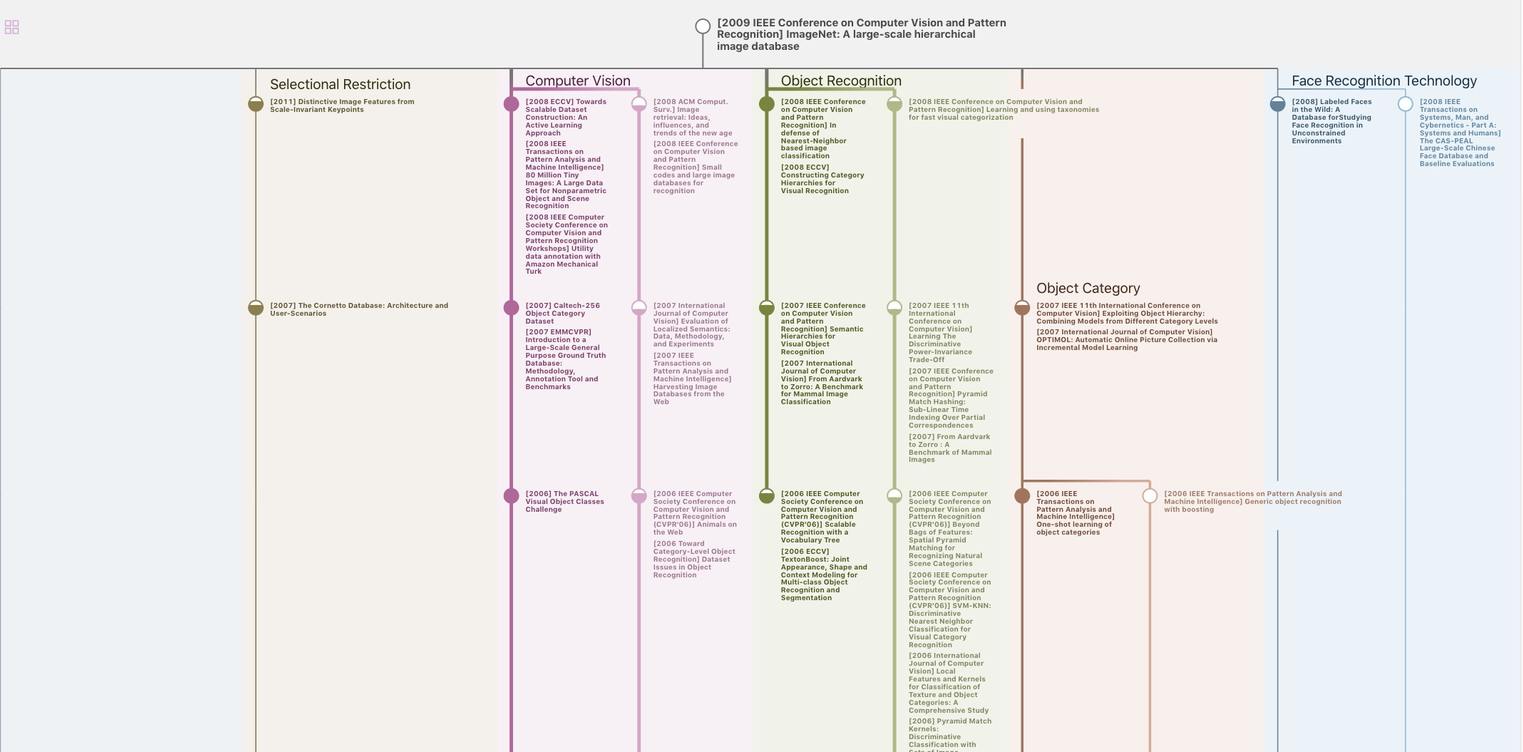
生成溯源树,研究论文发展脉络
Chat Paper
正在生成论文摘要