As you like it: Localization via paired comparisons.
arXiv: Machine Learning(2021)
摘要
Suppose that we wish to estimate a vector from a set of binary paired comparisons of the form $mathbf{x}$ is closer to $mathbf{p}$ than to $mathbf{q}$ for various choices of vectors $mathbf{p}$ and $mathbf{q}$. The problem of estimating from this type of observation arises in a variety of contexts, including nonmetric multidimensional scaling, unfolding, and ranking problems, often because it provides a powerful and flexible model of preference. We describe theoretical bounds for how well we can expect to estimate under a randomized model for $mathbf{p}$ and $mathbf{q}$. We also present results for the case where the comparisons are noisy and subject to some degree of error. Additionally, we show that under a randomized model for $mathbf{p}$ and $mathbf{q}$, a suitable number of binary paired comparisons yield a stable embedding of the space of target vectors. Finally, we also that we can achieve significant gains by adaptively changing the distribution for choosing $mathbf{p}$ and $mathbf{q}$.
更多查看译文
AI 理解论文
溯源树
样例
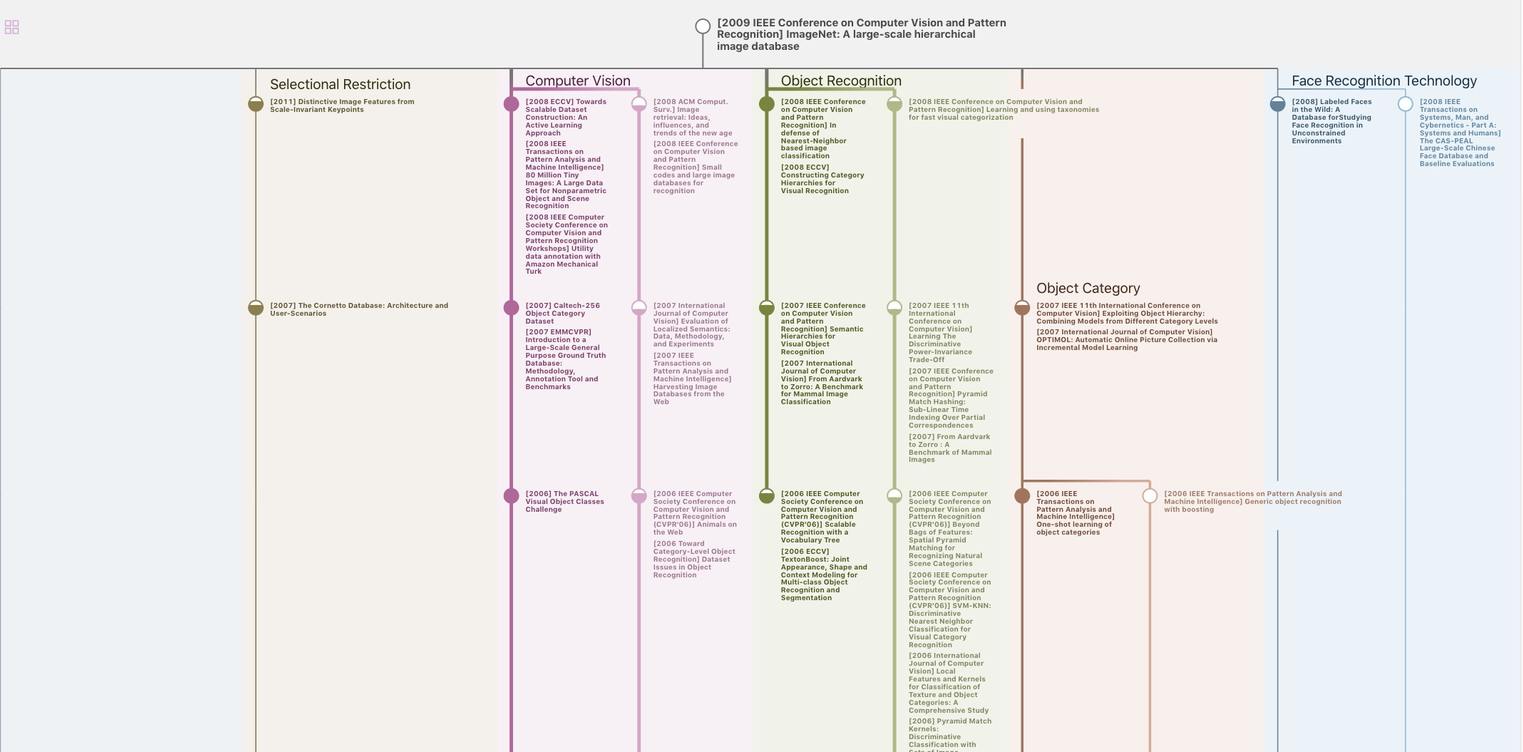
生成溯源树,研究论文发展脉络
Chat Paper
正在生成论文摘要