Long-term Visual Localization using Semantically Segmented Images
2018 IEEE International Conference on Robotics and Automation (ICRA)(2018)
摘要
Robust cross-seasonal localization is one of the major challenges in long-term visual navigation of autonomous vehicles. In this paper, we exploit recent advances in semantic segmentation of images, i.e., where each pixel is assigned a label related to the type of object it represents, to attack the problem of long-term visual localization. We show that semantically labeled 3-D point maps of the environment, together with semantically segmented images, can be efficiently used for vehicle localization without the need for detailed feature descriptors (SIFT, SURF, etc.). Thus, instead of depending on hand-crafted feature descriptors, we rely on the training of an image segmenter. The resulting map takes up much less storage space compared to a traditional descriptor based map. A particle filter based semantic localization solution is compared to one based on SIFT-features, and even with large seasonal variations over the year we perform on par with the larger and more descriptive SIFT-features, and are able to localize with an error below 1 m most of the time.
更多查看译文
关键词
particle filter based semantic localization solution,SIFT-features,vehicle localization,semantically labeled 3D point maps,autonomous vehicles,long-term visual navigation,robust cross-seasonal localization,semantically segmented images,long-term visual localization,image segmenter,hand-crafted feature descriptors
AI 理解论文
溯源树
样例
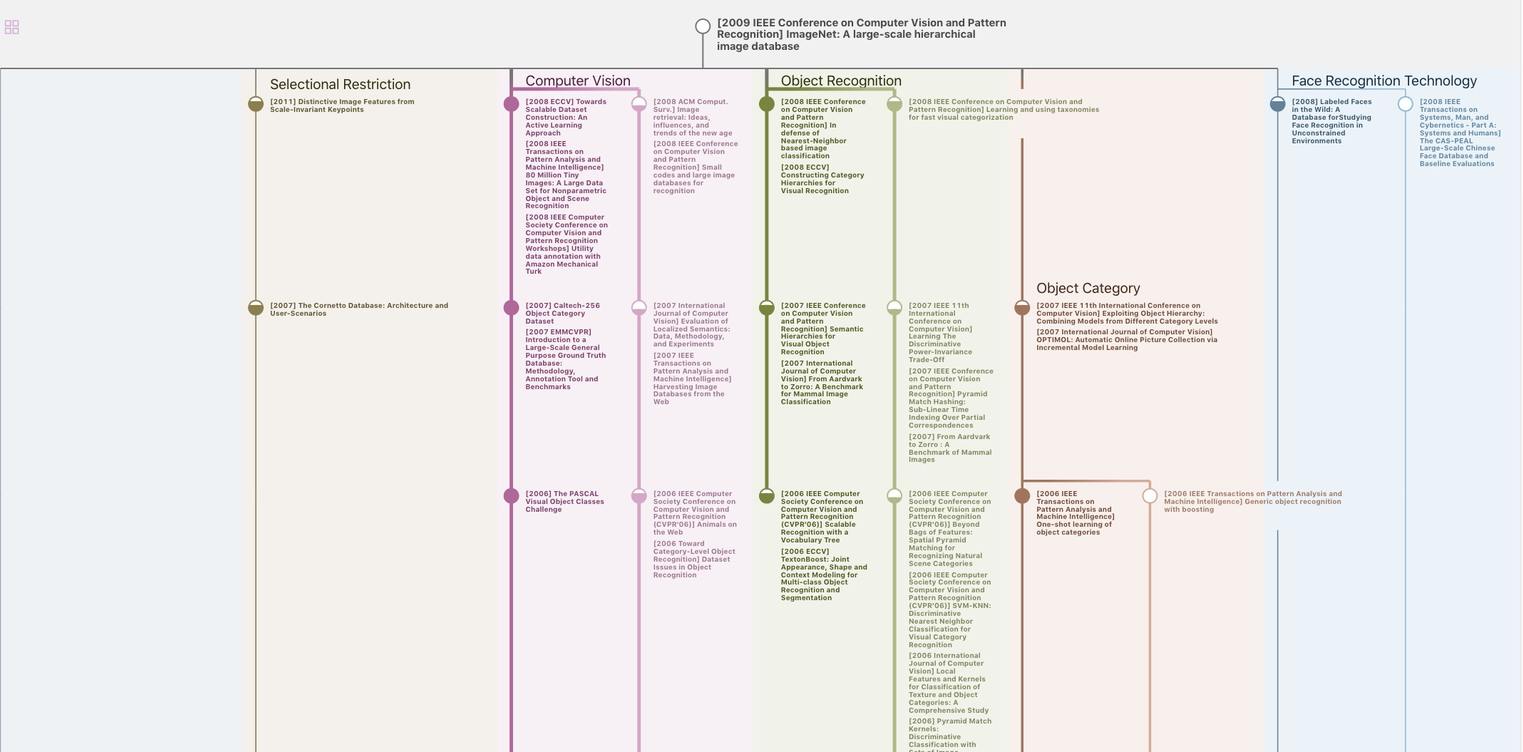
生成溯源树,研究论文发展脉络
Chat Paper
正在生成论文摘要