Sliced voxel representations with LSTM and CNN for 3D shape recognition.
Asia-Pacific Signal and Information Processing Association Annual Summit and Conference(2017)
摘要
We propose a sliced voxel representation, which we call Sliced Square Voxels (SSV), based on LSTM (Long Short-Term Memory) and CNN (Convolutional Neural Network) for three-dimensional shape recognition. Given an arbitrary 3D model, we first convert it into binary voxel of size 32x32x32. Then, after a view position is fixed, we slice the binary voxel data vertically in the depth direction. To utilize the 2D projected shape information of the sliced voxels, CNN has been applied. The output of CNN is fed into LSTM, which is our main idea, where the spatial topology is supposed to be favored with LSTM. From our experiments, our proposed method turns out to be superior to the baseline method which we prepared using 3DCNN. We further compared with related previous methods, using large-scale 3D model dataset (ModelNet10 and ModelNet40), and our proposed methods outperformed them.
更多查看译文
关键词
LSTM,CNN,3D shape recognition,sliced voxel representation,Sliced Square Voxels,Long Short-Term Memory,Convolutional Neural Network,three-dimensional shape recognition,binary voxel data,2D projected shape information,3D model
AI 理解论文
溯源树
样例
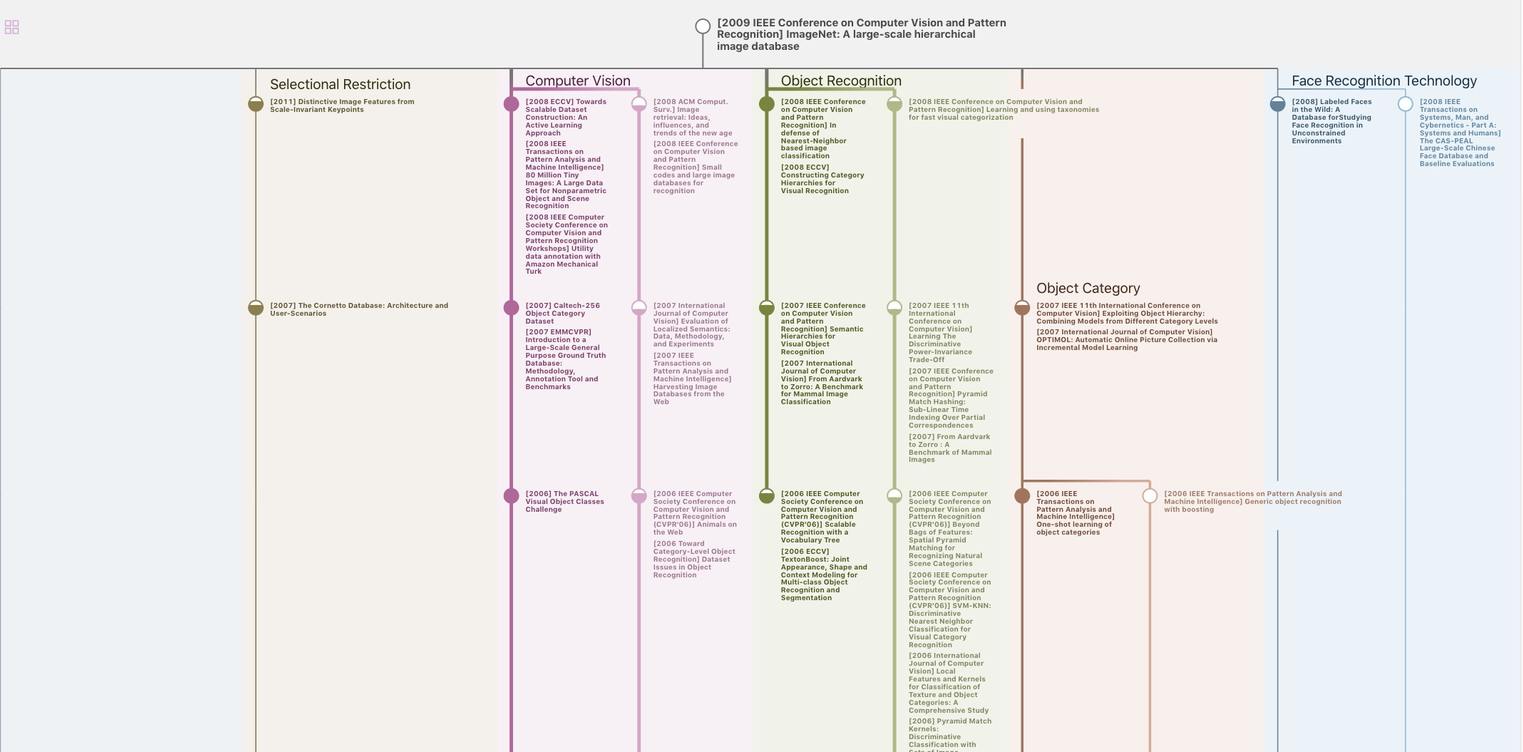
生成溯源树,研究论文发展脉络
Chat Paper
正在生成论文摘要