Leveraging Long and Short-Term Information in Content-Aware Movie Recommendation via Adversarial Training
arXiv: Information Retrieval(2020)
摘要
Movie recommendation systems provide users with ranked lists of movies based on individual's preferences and constraints. Two types of models are commonly used to generate ranking results: 1) long-term models and 2) session-based models. The long-term-based models represent the interactions between users and movies that are supposed to change slowly across time, while the session-based models encode the information of users' interests and changing dynamics of movies' attributes in short terms. In this paper, we propose the LSIC model, leveraging long and short-term information for content-aware movie recommendation using adversarial training. In the adversarial process, we train a generator as an agent of reinforcement learning which recommends the next movie to a user sequentially. We also train a discriminator which attempts to distinguish the generated list of movies from the real records. The poster information of movies is integrated to further improve the performance of movie recommendation, which is specifically essential when few ratings are available. The experiments demonstrate that the proposed model has robust superiority over competitors and achieves the state-of-the-art results.
更多查看译文
关键词
Adversarial learning,content-aware recommendation,top-N movie recommendation
AI 理解论文
溯源树
样例
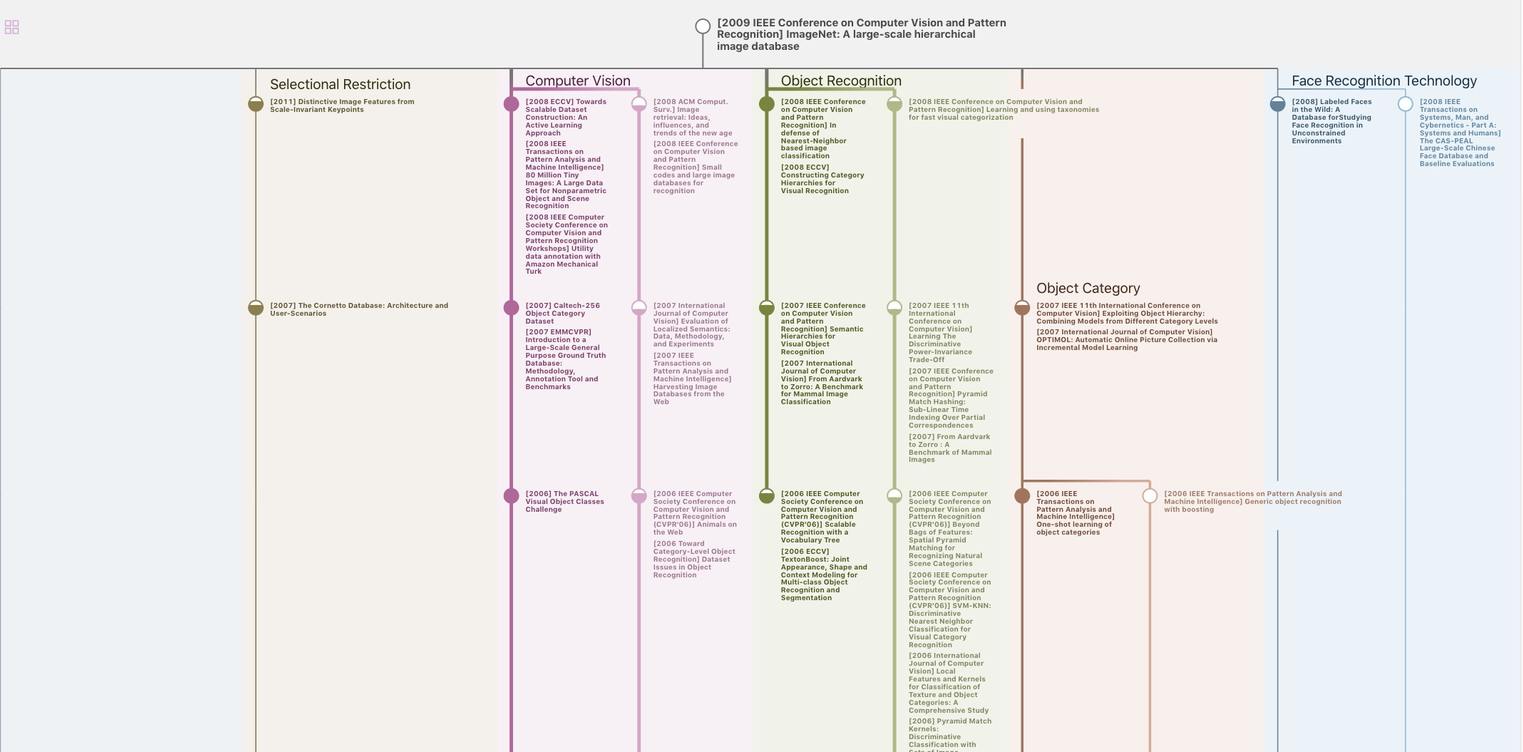
生成溯源树,研究论文发展脉络
Chat Paper
正在生成论文摘要