Im2Pano3D: Extrapolating 360 Structure and Semantics Beyond the Field of View
2018 IEEE/CVF Conference on Computer Vision and Pattern Recognition(2017)
摘要
We present Im2Pano3D, a convolutional neural network that generates a dense prediction of 3D structure and a probability distribution of semantic labels for a full 360 panoramic view of an indoor scene when given only a partial observation (<= 50%) in the form of an RGB-D image. To make this possible, Im2Pano3D leverages strong contextual priors learned from large-scale synthetic and real-world indoor scenes. To ease the prediction of 3D structure, we propose to parameterize 3D surfaces with their plane equations and train the model to predict these parameters directly. To provide meaningful training supervision, we use multiple loss functions that consider both pixel level accuracy and global context consistency. Experiments demon- strate that Im2Pano3D is able to predict the semantics and 3D structure of the unobserved scene with more than 56% pixel accuracy and less than 0.52m average distance error, which is significantly better than alternative approaches.
更多查看译文
关键词
real-world indoor scenes,Im2Pano3D,probability distribution,convolutional neural network,3D structure,semantic labels,RGB-D image
AI 理解论文
溯源树
样例
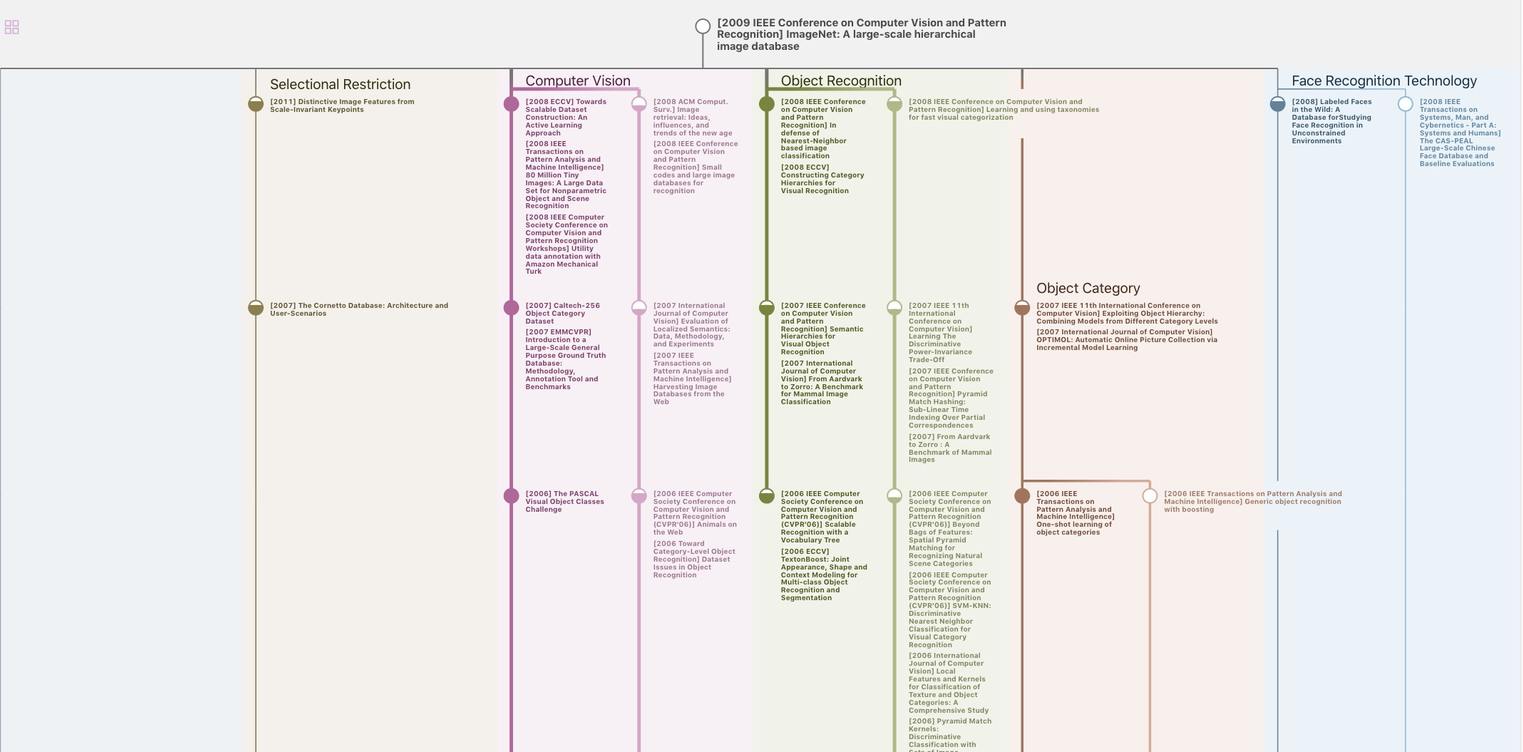
生成溯源树,研究论文发展脉络
Chat Paper
正在生成论文摘要