A machine learning based demand charge management solution
2017 IEEE PES Innovative Smart Grid Technologies Conference Europe (ISGT-Europe)(2017)
摘要
In contrast with battery storages installed by distribution network operators (DNOs) which are responsible to shave the daily peaks, behind the meter energy managements systems (BTM-EMSs) are mostly installed by the commercial/industrial units to minimize the maximum demand peak over a billing period. Therefore, the daily optimization frameworks used in DNO side battery storages could not be applied to control BTM battery storages due to unnecessary degradation of the battery storages. As an alternative, two-layer control frameworks could be applied in BTM applications in which the minimum achievable of the maximum peak value in the next month (called demand charge threshold (DCT)) is determined in the monthly layer, and the daily layer schedules battery charge and discharge to realize the predetermined DCT. However, the unpredicted behavior of individual electricity loads in coming month casts a shadow over the functionality of such two layer demand charge management solutions(DCMS). In this paper, a novel two-layer DCMS is proposed in which, the monthly layer forecasts the near optimal DCT value for the next month based on the behavior of the load DCT values in the last few months. In the absence of the long history of the load profile, the similar DCT profiles of the other loads available in the data set are used to construct the DCT forecast model. The efficiency of the proposed approach is validated through the simulation studies on the real value load data.
更多查看译文
关键词
Energy management systems,battery storage,demand charge,forecasting,time series
AI 理解论文
溯源树
样例
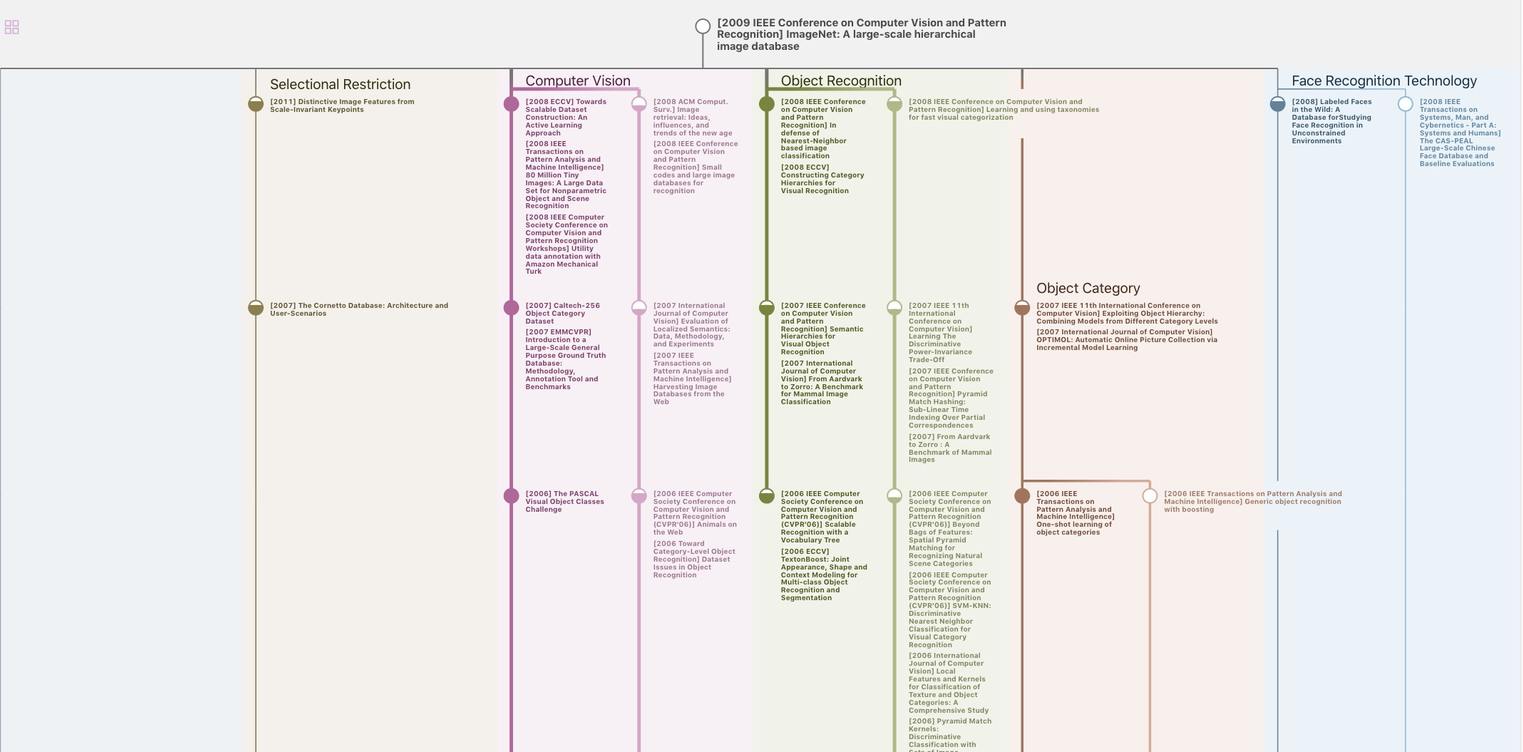
生成溯源树,研究论文发展脉络
Chat Paper
正在生成论文摘要