Learning Data-Efficient Hierarchical Features for Robotic Graspable Object Recognition
2017 IEEE International Conference on Advanced Intelligent Mechatronics (AIM)(2017)
摘要
Robotic graspable object recognition is a crucial ingredient in many exciting autonomous manipulation applications. However, identifying complex image features from limited data remains largely unsolved. In this paper, we leverage the advantages of two kinds of feature representation approaches, kernel descriptors and deep neural networks, to present a novel hierarchical feature learning framework for robotic graspable object recognition. This work enables the recovery of sparse and compressible features from limited data examples. Firstly, we design multiple kernel descriptors from the raw RGB-D images to adequately capture the discriminative structure of the object. Then, the extracted abstract representations are transferred to a four-layer deep neural network to generate more representative features for final graspable discrimination. Our network obtains impressive generalization capability with limited training data. Extensive experiments are carried out to validate the proposed method and the results show the state-of- the-art performance in discriminating graspable object task under limited-data.
更多查看译文
关键词
data-efficient hierarchical features,robotic graspable object recognition,autonomous manipulation applications,complex image features,feature representation,kernel descriptors,deep neural networks,hierarchical feature learning framework,raw RGB-D images,abstract representations,four-layer deep neural network,graspable discrimination,limited training data
AI 理解论文
溯源树
样例
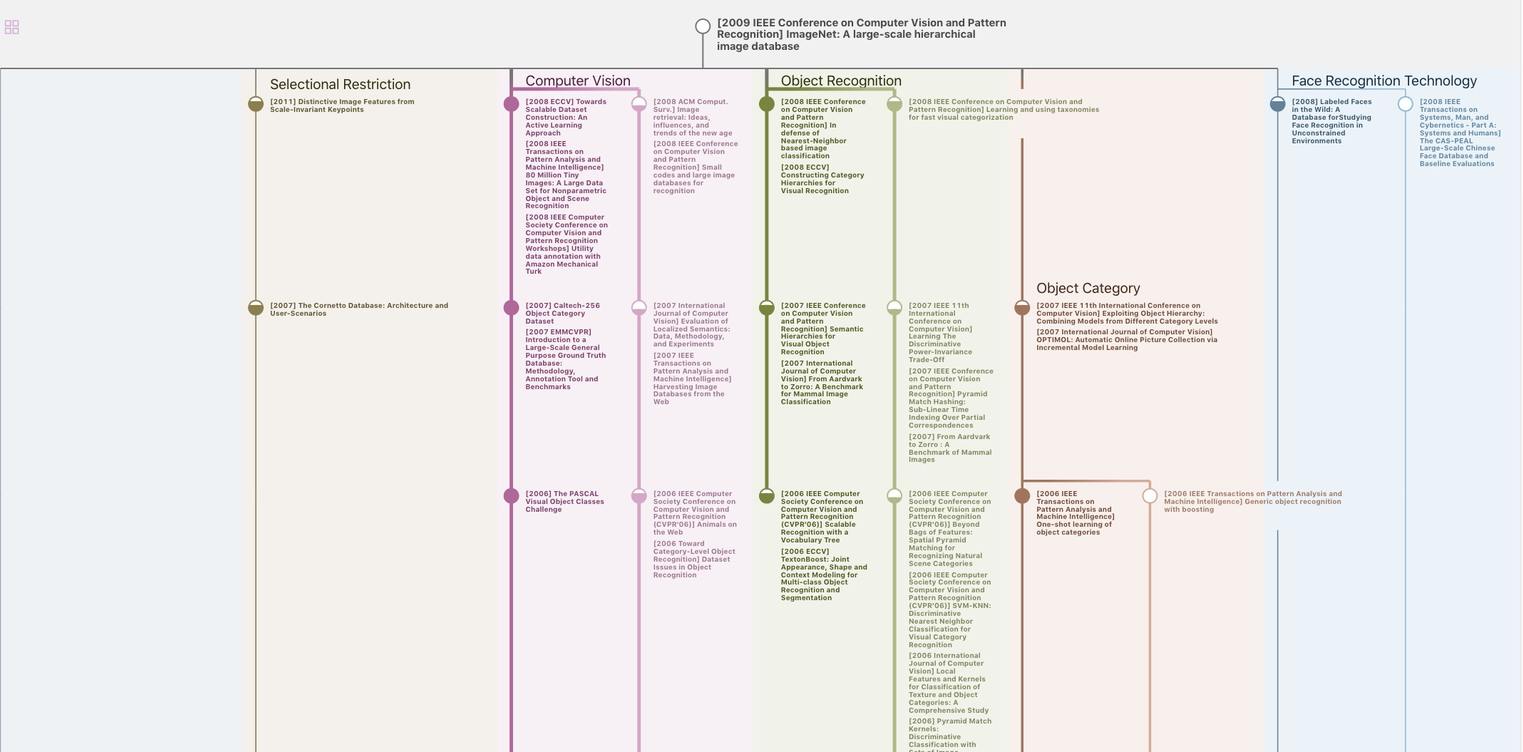
生成溯源树,研究论文发展脉络
Chat Paper
正在生成论文摘要