Machine learning for neural decoding.
arXiv: Neurons and Cognition(2017)
摘要
Despite rapid advances in machine learning tools, the majority of neural decoding approaches still use traditional methods. Improving the performance of neural decoding algorithms allows us to better understand the information contained in a neural population, and can help advance engineering applications such as brain machine interfaces. Here, we apply modern machine learning techniques, including neural networks and gradient boosting, to decode from spiking activity in 1) motor cortex, 2) somatosensory cortex, and 3) hippocampus. We compare the predictive ability of these modern methods with traditional decoding methods such as Wiener and Kalman filters. Modern methods, in particular neural networks and ensembles, significantly outperformed the traditional approaches. For instance, for all of the three brain areas, an LSTM decoder explained over 40% of the unexplained variance from a Wiener filter. These results suggest that modern machine learning techniques should become the standard methodology for neural decoding. We provide a tutorial and code to facilitate wider implementation of these methods.
更多查看译文
AI 理解论文
溯源树
样例
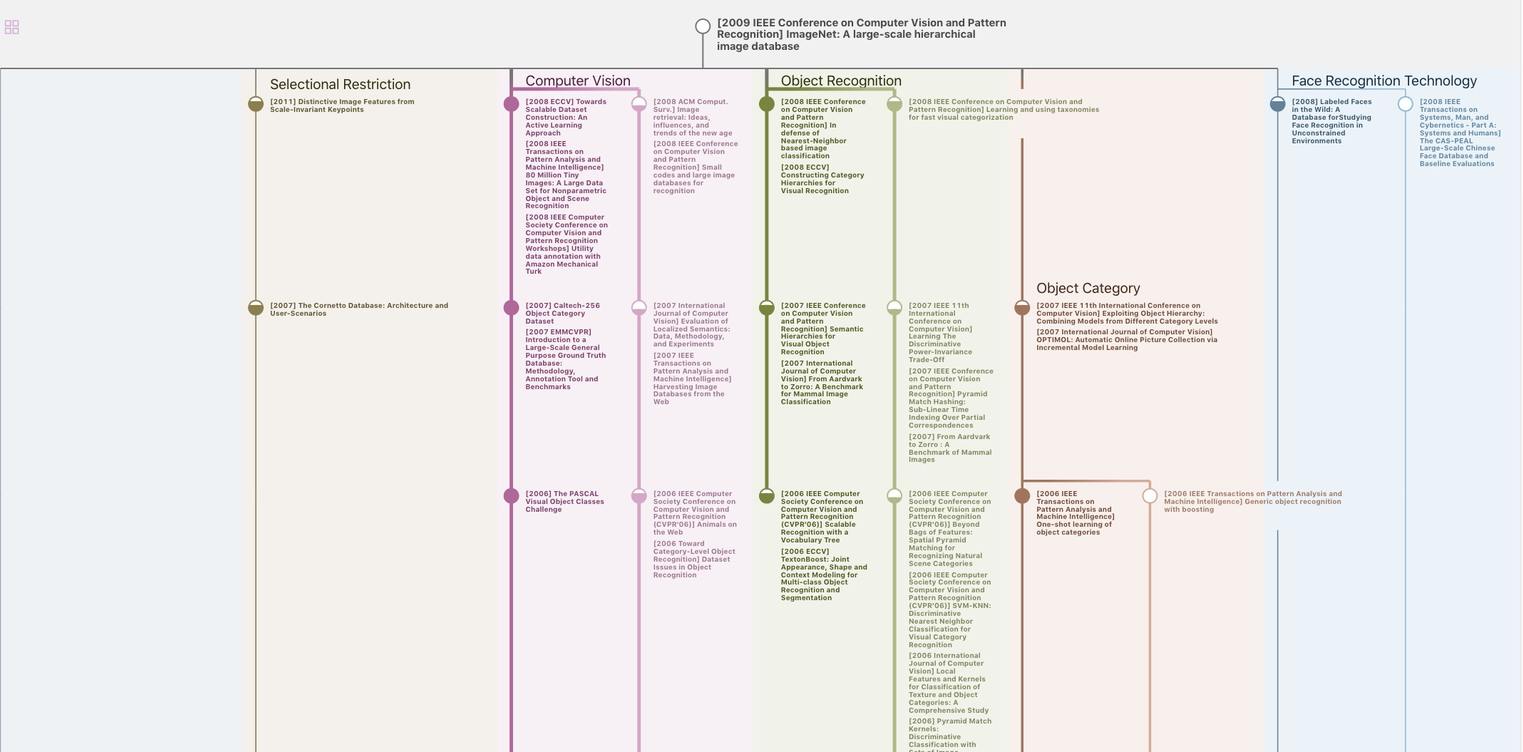
生成溯源树,研究论文发展脉络
Chat Paper
正在生成论文摘要