X-PACS: eXPlaining Anomalies by Characterizing Subspaces.
arXiv: Learning(2017)
摘要
Anomaly detection has numerous critical applications in finance, security, etc. and has been vastly studied. In this paper, we tap into a gap in the literature and consider a complementary problem: anomaly description. Interpretation of anomalies has important implications for decision makers, from being able to troubleshoot and prioritize their actions to making policy changes for prevention. We present a new method called X-PACS which reverse-engineers the known anomalies in a dataset by identifying a few anomalous patterns that they form along with the characterizing subspace of features that separates them from normal instances. From a descriptive data mining perspective, our solution has five key desired properties. It can unearth anomalous patterns (i) of multiple different types, (ii) hidden in arbitrary subspaces of a high dimensional space, (iii) interpretable by the end-users, (iv) succinct, providing the shortest data description, and finally (v) different from normal patterns of the data. There is no existing work on anomaly description that satisfy all of these desiderata simultaneously. While not our primary goal, anomalous patterns X-PACS finds can further be seen as multiple, interpretable signatures and can be used for detection. We show the effectiveness of X-PACS in explanation as well as detection tasks on 9 real-world datasets.
更多查看译文
AI 理解论文
溯源树
样例
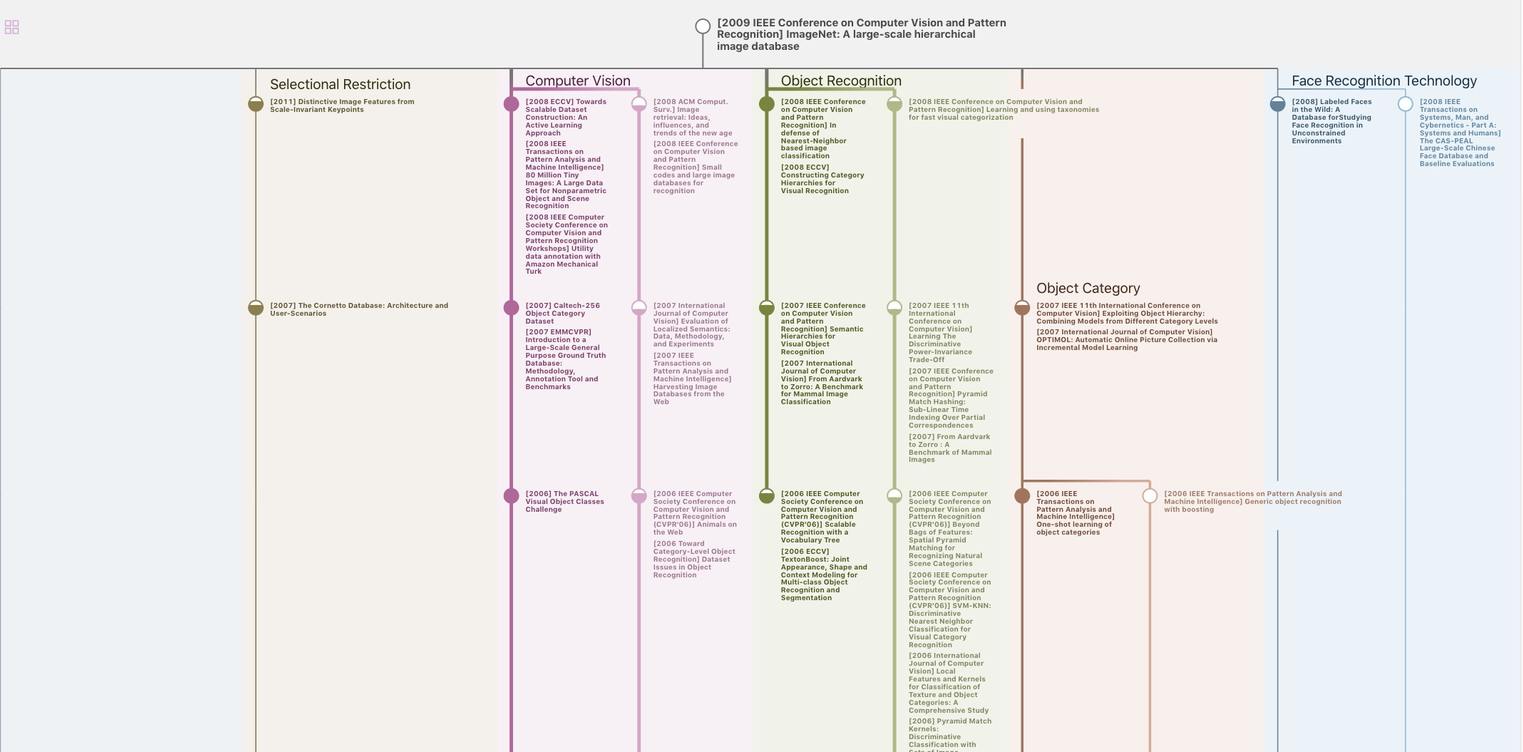
生成溯源树,研究论文发展脉络
Chat Paper
正在生成论文摘要