ADaPTION: Toolbox and Benchmark for Training Convolutional Neural Networks with Reduced Numerical Precision Weights and Activation
arXiv: Learning(2017)
摘要
Deep Neural Networks (DNNs) and Convolutional Neural Networks (CNNs) are useful for many practical tasks in machine learning. Synaptic weights, as well as neuron activation functions within the deep network are typically stored with high-precision formats, e.g. 32 bit floating point. However, since storage capacity is limited and each memory access consumes power, both storage capacity and memory access are two crucial factors in these networks. Here we present a method and present the ADaPTION toolbox to extend the popular deep learning library Caffe to support training of deep CNNs with reduced numerical precision of weights and activations using fixed point notation. ADaPTION includes tools to measure the dynamic range of weights and activations. Using the ADaPTION tools, we quantized several CNNs including VGG16 down to 16-bit weights and activations with only 0.8 quantization, especially of the activations, leads to increase of up to 50 sparsity especially in early and intermediate layers, which we exploit to skip multiplications with zero, thus performing faster and computationally cheaper inference.
更多查看译文
AI 理解论文
溯源树
样例
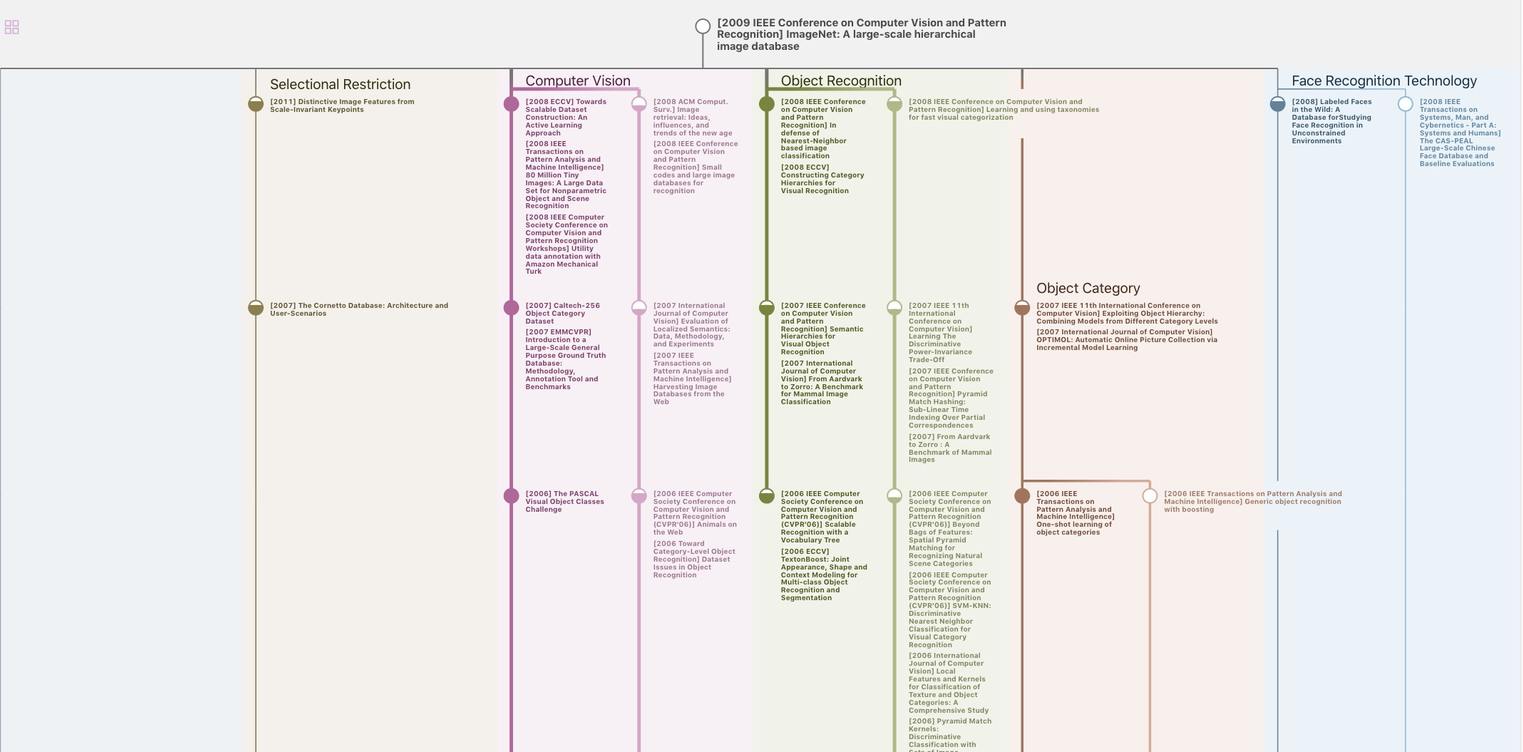
生成溯源树,研究论文发展脉络
Chat Paper
正在生成论文摘要