Data Analytics for Managing Power in Commercial Buildings.
TCPS(2017)
摘要
Commercial buildings are significant consumers of electricity. We propose a number of methods for managing power in commercial buildings. The first step toward better energy management in commercial buildings is monitoring consumption. However, instrumenting every electrical panel in a large commercial building is an expensive proposition. In this article, we demonstrate that it is also unnecessary. Specifically, we propose a greedy meter (sensor) placement algorithm based on maximization of information gain subject to a cost constraint. The algorithm provides a near-optimal solution guarantee, and our empirical results demonstrate a 15% improvement in prediction power over conventional methods. Next, to identify power-saving opportunities, we use an unsupervised anomaly detection technique based on a low-dimensional embedding. Furthermore, to enable a building manager to effectively plan for demand response programs, we evaluate several solutions for fine-grained, short-term load forecasting. Our investigation reveals that support vector regression and an ensemble model work best overall. Finally, to better manage resources such as lighting and HVAC, we propose a semisupervised approach combining hidden Markov models (HMMs) and a standard classifier to model occupancy based on readily available port-level network statistics. We show that the proposed two-step approach simplifies the occupancy model while achieving good accuracy. The experimental results demonstrate an average occupancy estimation error of 9.3% with a potential reduction of 9.5% in lighting load using our occupancy models.
更多查看译文
关键词
Commercial buildings, anomaly detection, meter placement, occupancy modeling, power management, short-term load forecast
AI 理解论文
溯源树
样例
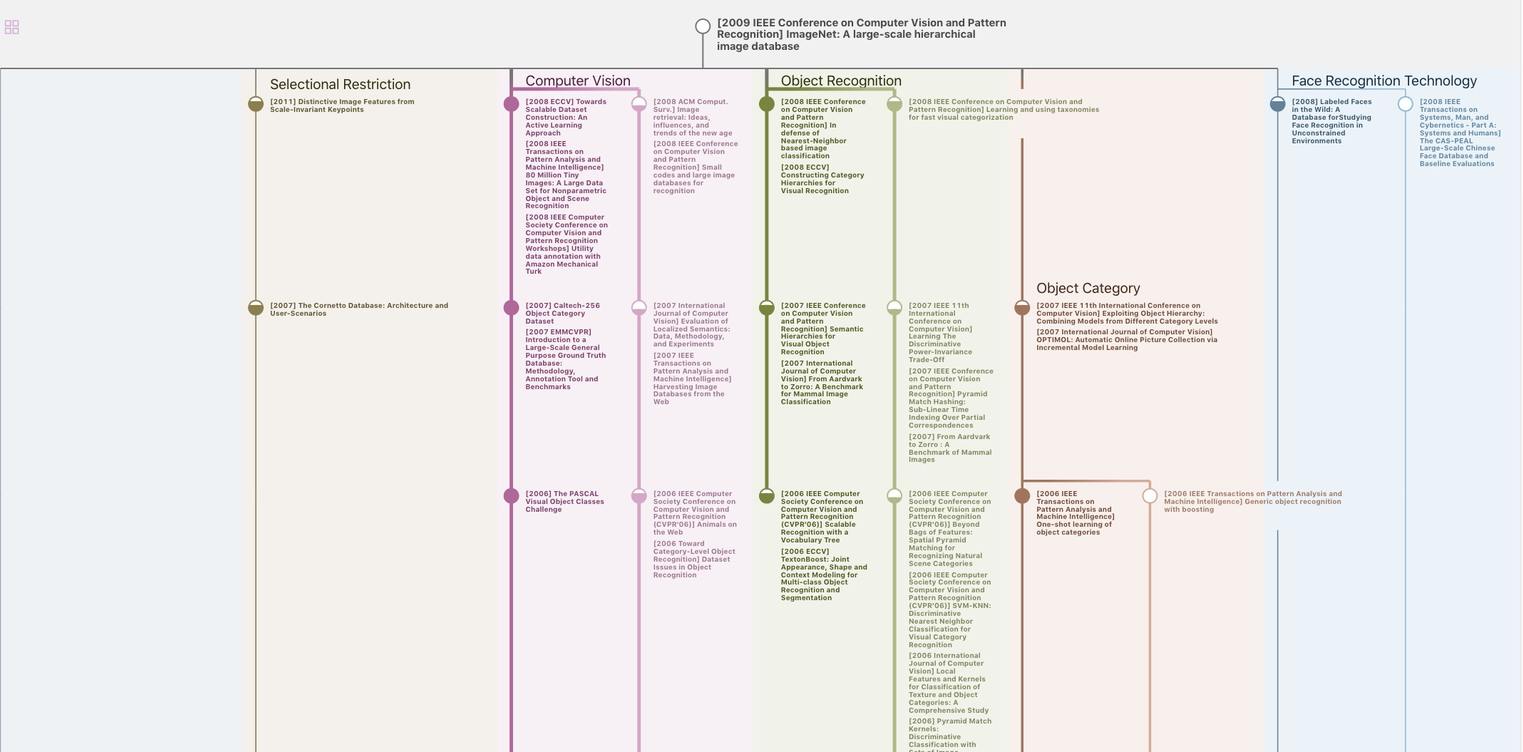
生成溯源树,研究论文发展脉络
Chat Paper
正在生成论文摘要