A step towards quantifying when an algorithm can and cannot predict an individual's wellbeing.
UbiComp '17: The 2017 ACM International Joint Conference on Pervasive and Ubiquitous Computing Maui Hawaii September, 2017(2017)
摘要
Researchers are exploring the ability to infer complex signals, such a mental wellbeing, from easily collected smartphone behavioral data. Rather than focusing on improving overall accuracy of such an approach, we seek to understand when we are and are not capable of predicting an individual's wellbeing. In particular, we consider the ability to predict daily wellbeing from smartphone GPS location data as a case study. We hypothesize that user characteristics, such as behavioral variability, level of depression symptoms, and amount of labeled data, are related to improvements in prediction accuracy. Our preliminary results indicate that there may be a relationship between an algorithm's ability to successfully predict an individual's wellbeing reports and the individual's location behavior variability. While further work is needed to improve model accuracy and confirm this relationship in a larger study, our work is a step in the necessary direction of understanding which individuals can be monitored with smartphone data.
更多查看译文
关键词
Mental health, Depression, Mobile health (mHealth), Mobile sensing, Supervised learning
AI 理解论文
溯源树
样例
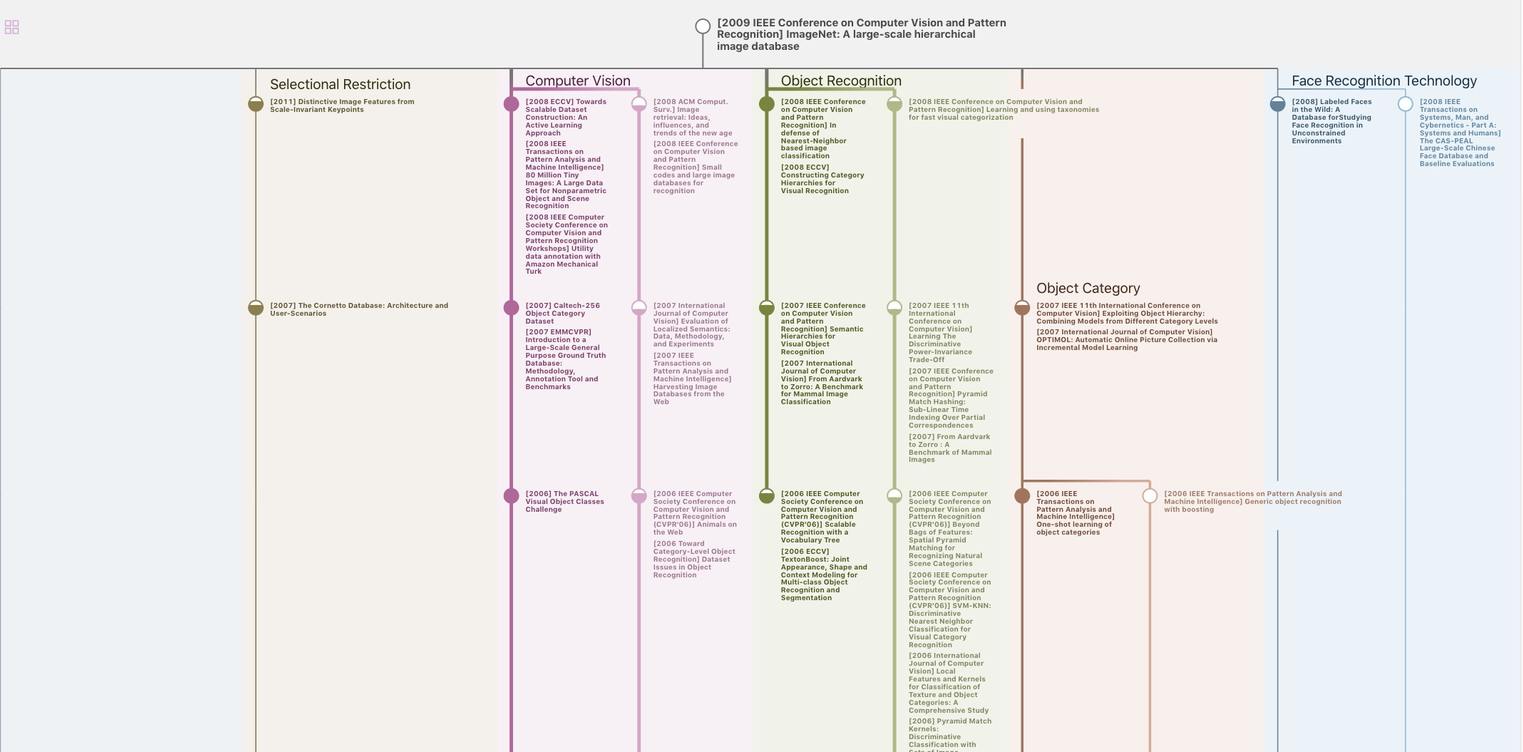
生成溯源树,研究论文发展脉络
Chat Paper
正在生成论文摘要