Content Embedding Regularized Matrix Factorization for Recommender Systems
2017 IEEE International Congress on Big Data (BigData Congress)(2017)
摘要
In recommender systems, numerous efforts have been made on utilizing textual information in matrix factorization to alleviate the problem of data sparsity. Recently, some of the works have explored neural networks to go for an in-depth understanding of textual item content, and further generate more accurate item latent models. These works achieve impressive effectiveness on performing recommendations. Nevertheless, there remains an open issue as how to effectively exploit description documents of both users and items in matrix factorization. In this paper, we proposed content embedding regularized matrix factorization (CERMF) to address this issue. CERMF adopts convolution neural networks to simultaneously generate the independent embedding representations for the users and the items. Then, dual embeddings are used to regularize the generation of latent models for users and items. Experimental results are proved that exploiting content information of both users and items dramatically improves the accuracy of recommendations as compared with the state-of-the-art recommendation models.
更多查看译文
关键词
Recommender Systems,Matrix Factorization,Convolutional Neural Network,Regularization
AI 理解论文
溯源树
样例
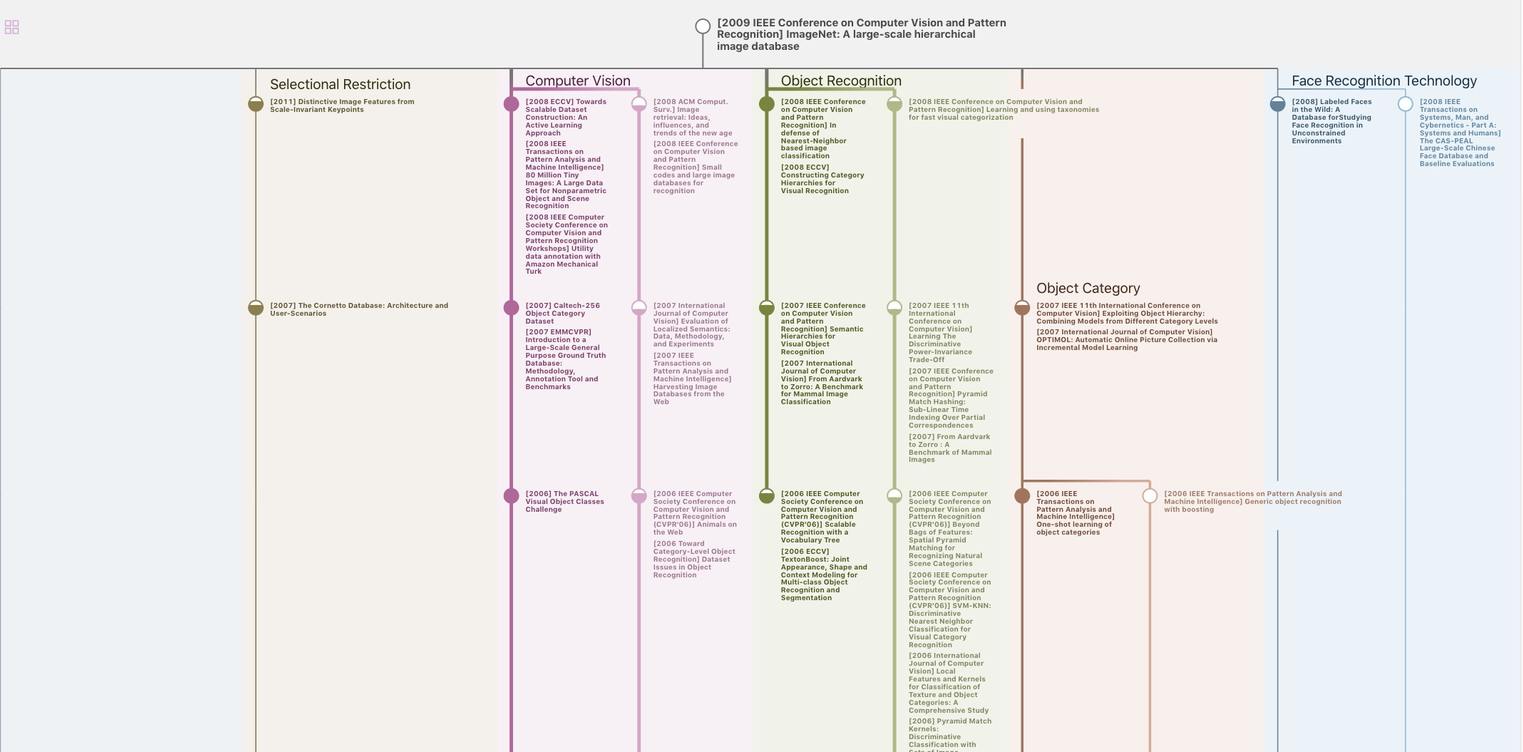
生成溯源树,研究论文发展脉络
Chat Paper
正在生成论文摘要