Stacked Hourglass Network for Robust Facial Landmark Localisation.
IEEE Computer Society Conference on Computer Vision and Pattern Recognition Workshops(2017)
摘要
With the increasing number of public available training data for face alignment, the regression-based methods attracted much attention and have become the dominant methods to solve this problem. There are two main factors, the variance of the regression target and the capacity of regression model, affecting the performance of the regression task. In this paper, we present a Stacked Hourglass Network for robust facial landmark localisation. We first adopt a supervised face transformation to remove the translation, scale and rotation variation of each face, in order to reduce the variance of the regression target. Then we employ a deep convolutional neural network named Stacked Hourglass Network to increase the capacity of the regression model. To better evaluate the proposed method, we reimplement two popular cascade shape regression models, SDM and LBF, for comparison. Extensive experiments on four challenging datasets, COFW, IBUG, 300W and the Menpo Benchmark, prove the effectiveness of the proposed method.
更多查看译文
关键词
stacked hourglass network,robust facial landmark localisation,face alignment,public available training data,regression task,supervised face transformation,deep convolutional neural network,cascade shape regression models,SDM,LBF,COFW,IBUG,Menpo benchmark
AI 理解论文
溯源树
样例
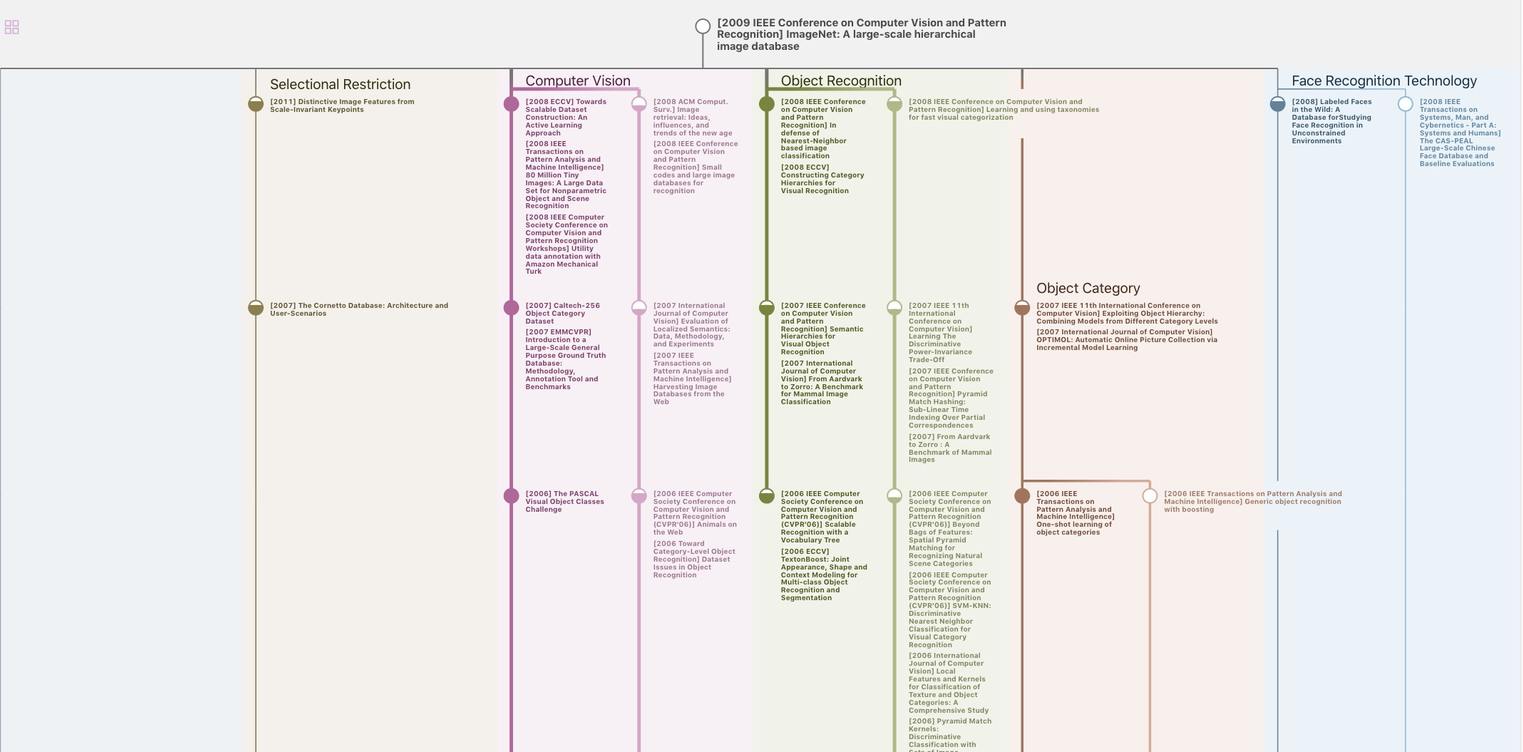
生成溯源树,研究论文发展脉络
Chat Paper
正在生成论文摘要