Knowledge Discovery of Complex Data Using Gaussian Mixture Models.
Lecture Notes in Computer Science(2017)
摘要
With the explosive growth of data quantity and variety, the representation and analysis of complex data becomes a more and more challenging task in many modern applications. As a general class of probabilistic distribution functions, Gaussian Mixture Models have the ability to approximate arbitrary distributions in a concise way, making them very suitable for the representation of complex data. To facilitate efficient queries and following analysis, we generalize Euclidean distance to Gaussian Mixture Models and derive the closed-form expression called Infinite Euclidean Distance. Our metric enables efficient and accurate similarity calculations. For the analysis of complex data, we model two real-world data sets, NBA player statistic and the weather data of airports, into Gaussian Mixture Models, and we compare the performance of Infinite Euclidean Distance to previous similarity measures on both classification and clustering tasks. Experimental evaluations demonstrate the efficiency and effectiveness of Infinite Euclidean Distance and Gaussian Mixture Models on the analysis of complex data.
更多查看译文
AI 理解论文
溯源树
样例
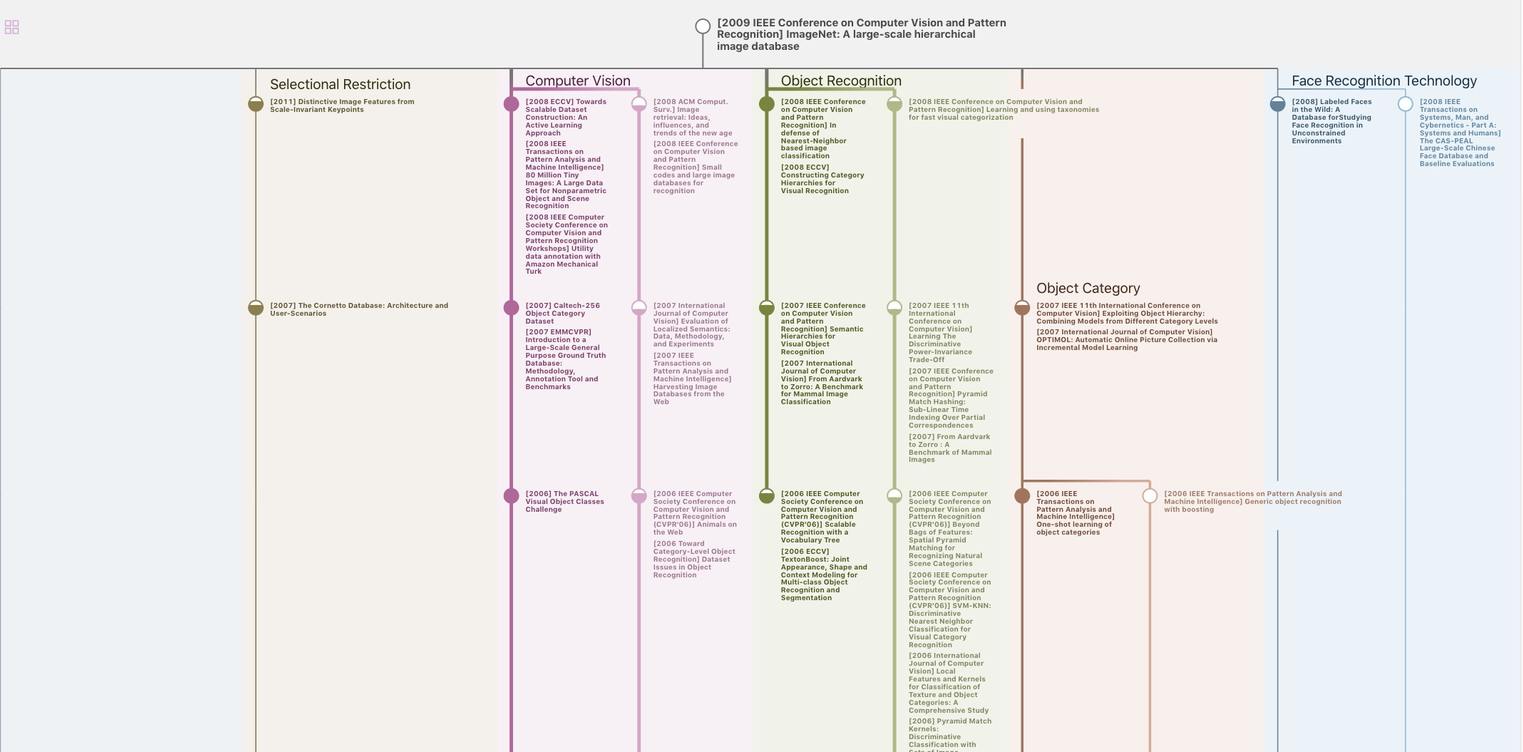
生成溯源树,研究论文发展脉络
Chat Paper
正在生成论文摘要