Corroboration via Provenance Patterns.
TaPP(2017)
摘要
In today's distributed and heterogeneous systems, provenance data is becoming increasingly important for understanding process flow, tracing how outputs came about, and enabling users to make more informed decisions based on such outputs. However, within such systems, the sources (computational or human) that generate provenance may belong to different stakeholders operating under different policies. Thus, being autonomous and self-interested, these stakeholders may claim untrue data to protect their interests (e.g. to justify bad performance). In response, this paper proposes a corroboration methodology for verifying a claim made by a source, via confirming it against the claims of other sources. In particular, given a claim in PROV, this claim is generalised to varying levels of abstraction, deriving two types of provenance templates, namely confirmation patterns , capturing the information to be confirmed, and witness patterns , capturing the relevant witnesses. These patterns are utilised to find relevant evidence, among the reports of others, that supports the claim, and to respectively estimate the reliability degree of the claim. The proposed corroboration methodology is illustrated via a case study in the service provision domain.
更多查看译文
AI 理解论文
溯源树
样例
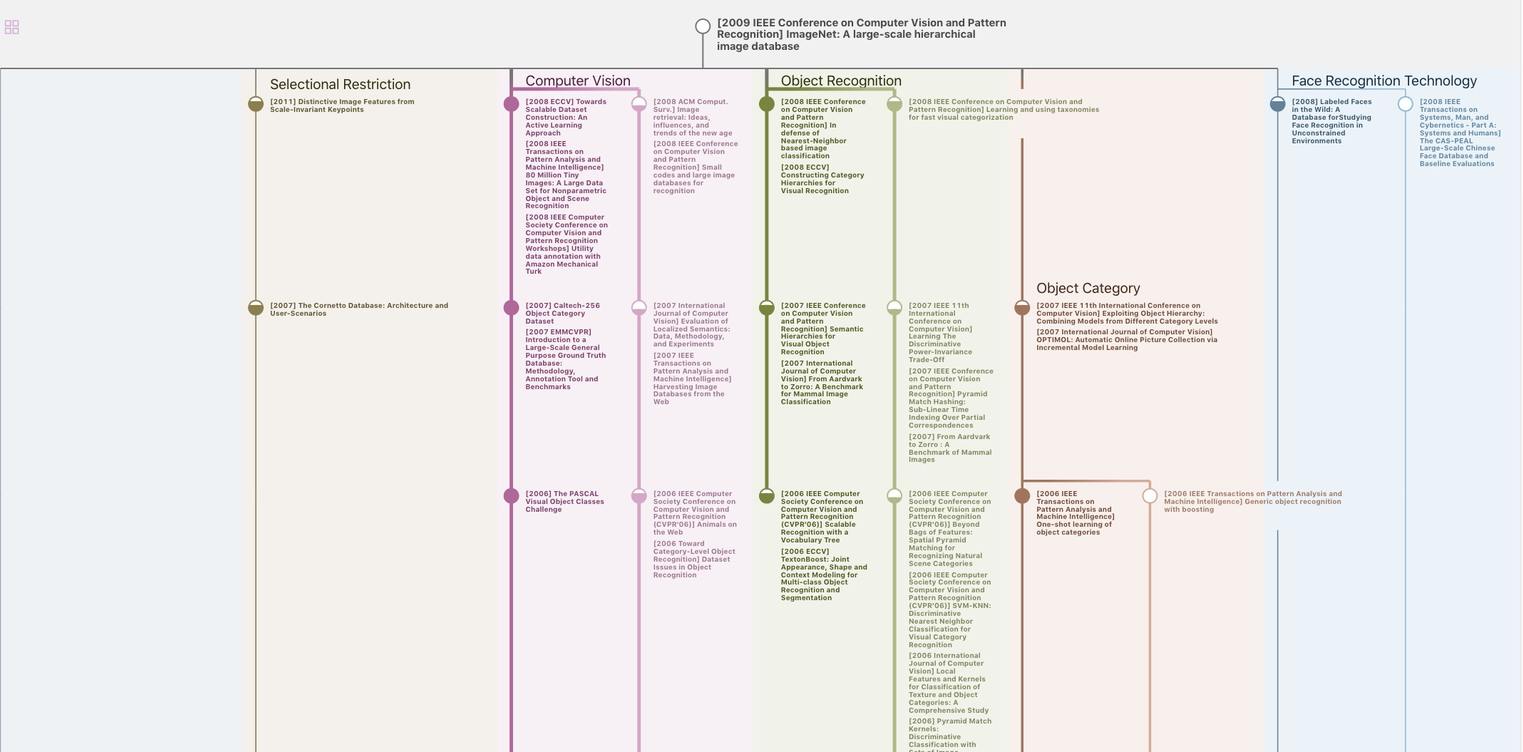
生成溯源树,研究论文发展脉络
Chat Paper
正在生成论文摘要