Long Short-Term Memory Network for Remaining Useful Life estimation
2017 IEEE International Conference on Prognostics and Health Management (ICPHM)(2017)
摘要
Remaining Useful Life (RUL) of a component or a system is defined as the length from the current time to the end of the useful life. Accurate RUL estimation plays a critical role in Prognostics and Health Management(PHM). Data driven approaches for RUL estimation use sensor data and operational data to estimate RUL. Traditional regression based approaches and recent Convolutional Neural Network (CNN) approach use features created from sliding windows to build models. However, sequence information is not fully considered in these approaches. Sequence learning models such as Hidden Markov Models (HMMs) and Recurrent Neural Networks (RNNs) have flaws when modeling sequence information. HMMs are limited to discrete hidden states and are known to have issues when modeling long-term dependencies in the data. RNNs also have issues with long-term dependencies. In this work, we propose a Long Short-Term Memory (LSTM) approach for RUL estimation, which can make full use of the sensor sequence information and expose hidden patterns within sensor data with multiple operating conditions, fault and degradation models. Extensive experiments using three widely adopted Prognostics and Health Management data sets show that LSTM for RUL estimation significantly outperforms traditional approaches for RUL estimation as well as Convolutional Neural Network (CNN).
更多查看译文
关键词
long-short-term memory network,remaining useful life estimation,RUL estimation,prognostics-and-health management,PHM,convolutional neural network approach,CNN,sequence learning models,HMM,hidden Markov models,RNN,recurrent neural networks,LSTM estimation
AI 理解论文
溯源树
样例
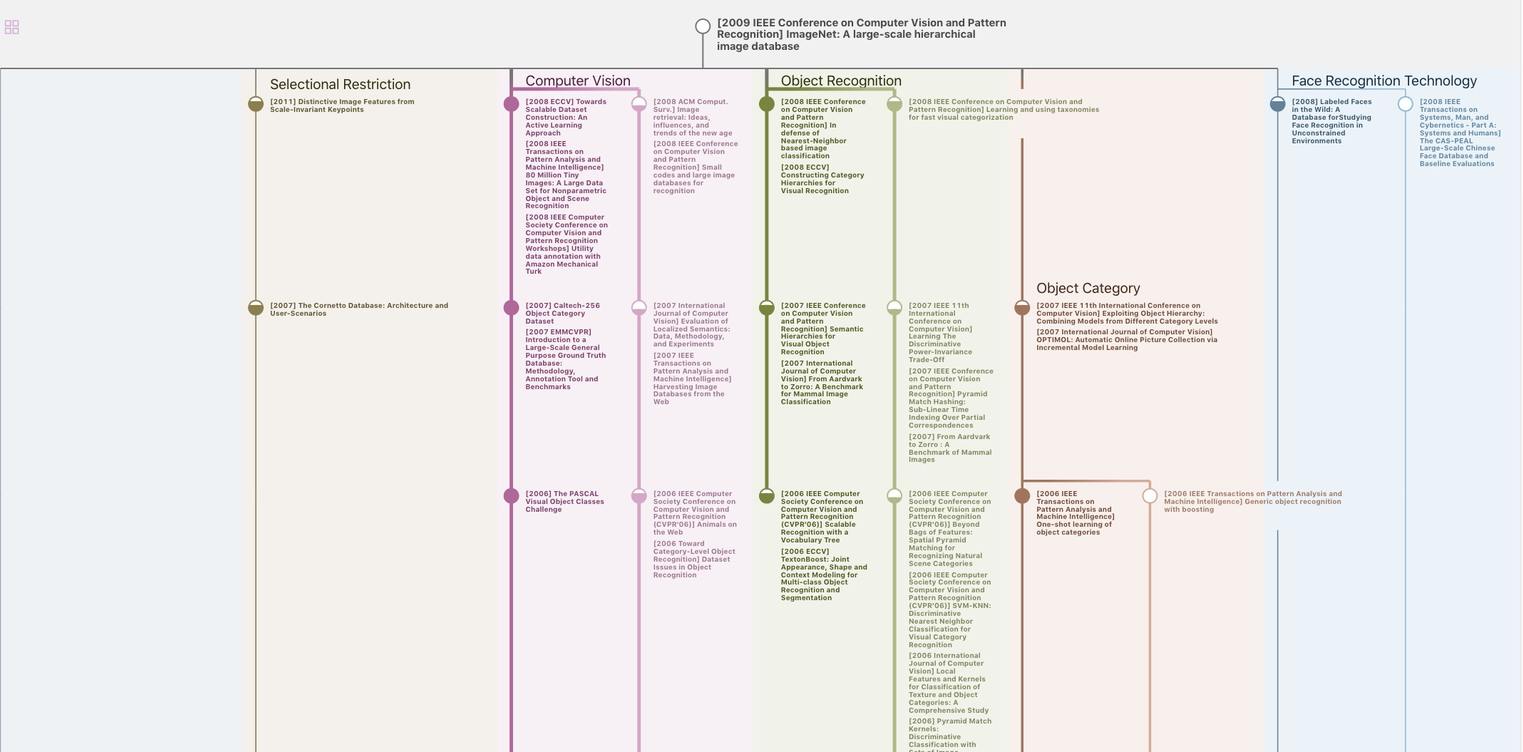
生成溯源树,研究论文发展脉络
Chat Paper
正在生成论文摘要