Multi-modal deep feature learning for RGB-D object detection.
Pattern Recognition(2017)
摘要
We present an approach for RGB-D object detection, which can exploit both modality-correlated and modality-specific relationships between RGB and depth images.The shared weights strategy and a parameter-free-correlation layer are introduced to extract the modality-correlated representations.The proposed approach can simultaneously generate RGB-D region proposals and perform region-wise RGB-D object recognition. We present a novel multi-modal deep feature learning architecture for RGB-D object detection. The current paradigm for object detection typically consists of two stages: objectness estimation and region-wise object recognition. Most existing RGB-D object detection approaches treat the two stages separately by extracting RGB and depth features individually, thus ignore the correlated relationship between these two modalities. In contrast, our proposed method is designed to take full advantages of both depth and color cues by exploiting both modality-correlated and modality-specific features and jointly performing RGB-D objectness estimation and region-wise object recognition. Specifically, shared weights strategy and a parameter-free correlation layer are exploited to carry out RGB-D-correlated objectness estimation and region-wise recognition in conjunction with RGB-specific and depth-specific procedures. The parameters of these three networks are simultaneously optimized via end-to-end multi-task learning. The multi-modal RGB-D objectness estimation results and RGB-D object recognition results are both boosted by late-fusion ensemble. To validate the effectiveness of the proposed approach, we conduct extensive experiments on two challenging RGB-D benchmark datasets, NYU Depth v2 and SUN RGB-D. The experimental results show that by introducing the modality-correlated feature representation, the proposed multi-modal RGB-D object detection approach is substantially superior to the state-of-the-art competitors. Moreover, compared to the expensive deep architecture (VGG16) that the state-of-the-art methods preferred, our approach, which is built upon more lightweight deep architecture (AlexNet), performs slightly better.
更多查看译文
关键词
RGB-D objectness estimation,RGB-D object detection,Multi-modal learning,Convolutional neural networks
AI 理解论文
溯源树
样例
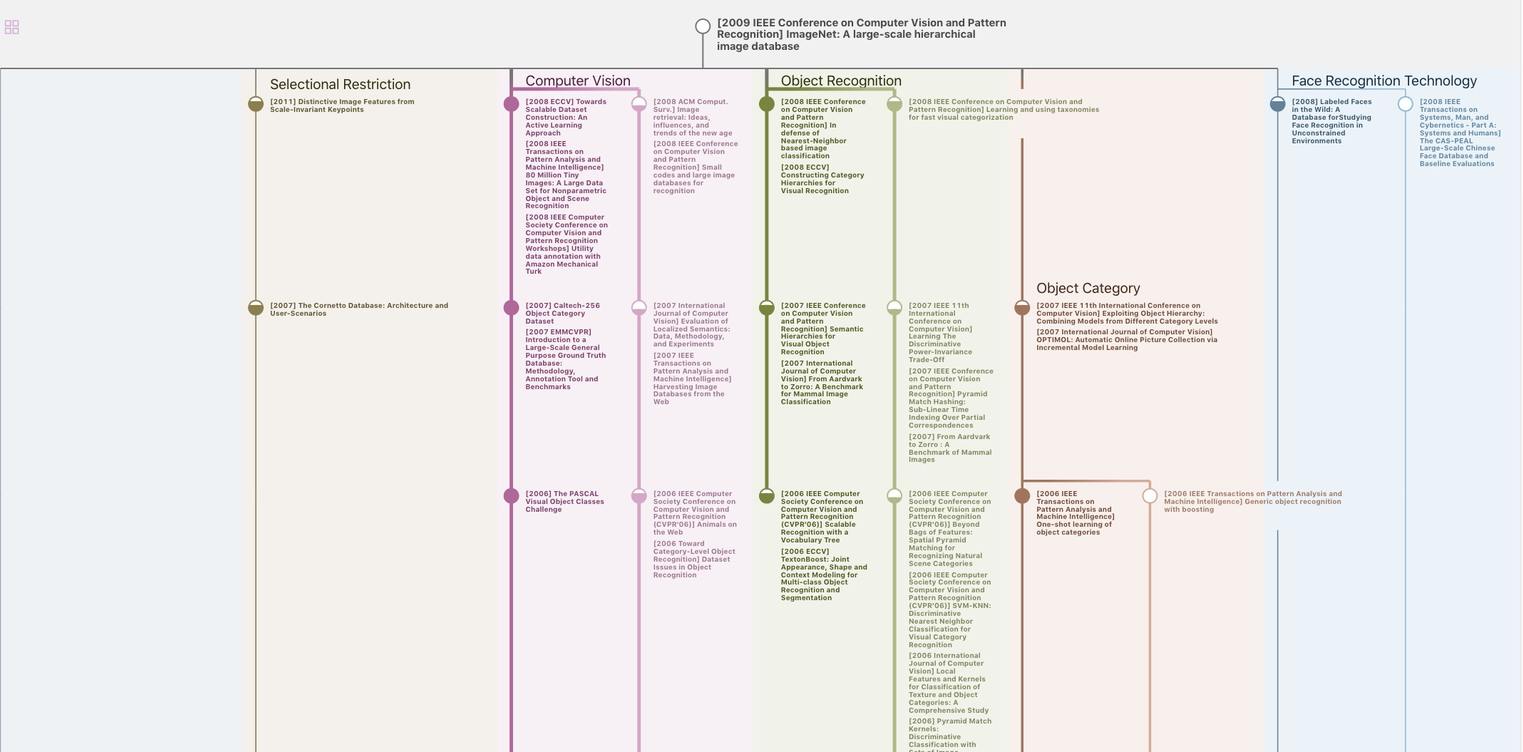
生成溯源树,研究论文发展脉络
Chat Paper
正在生成论文摘要