Convolutional neural networks on surfaces via seamless toric covers
ACM Trans. Graph.(2017)
摘要
The recent success of convolutional neural networks (CNNs) for image processing tasks is inspiring research efforts attempting to achieve similar success for geometric tasks. One of the main challenges in applying CNNs to surfaces is defining a natural convolution operator on surfaces. In this paper we present a method for applying deep learning to sphere-type shapes using a global seamless parameterization to a planar flat-torus, for which the convolution operator is well defined. As a result, the standard deep learning framework can be readily applied for learning semantic, high-level properties of the shape. An indication of our success in bridging the gap between images and surfaces is the fact that our algorithm succeeds in learning semantic information from an input of raw low-dimensional feature vectors. We demonstrate the usefulness of our approach by presenting two applications: human body segmentation, and automatic landmark detection on anatomical surfaces. We show that our algorithm compares favorably with competing geometric deep-learning algorithms for segmentation tasks, and is able to produce meaningful correspondences on anatomical surfaces where hand-crafted features are bound to fail.
更多查看译文
关键词
Geometric deep learning,Convolutional neural network,Shape analysis,shape segmentation
AI 理解论文
溯源树
样例
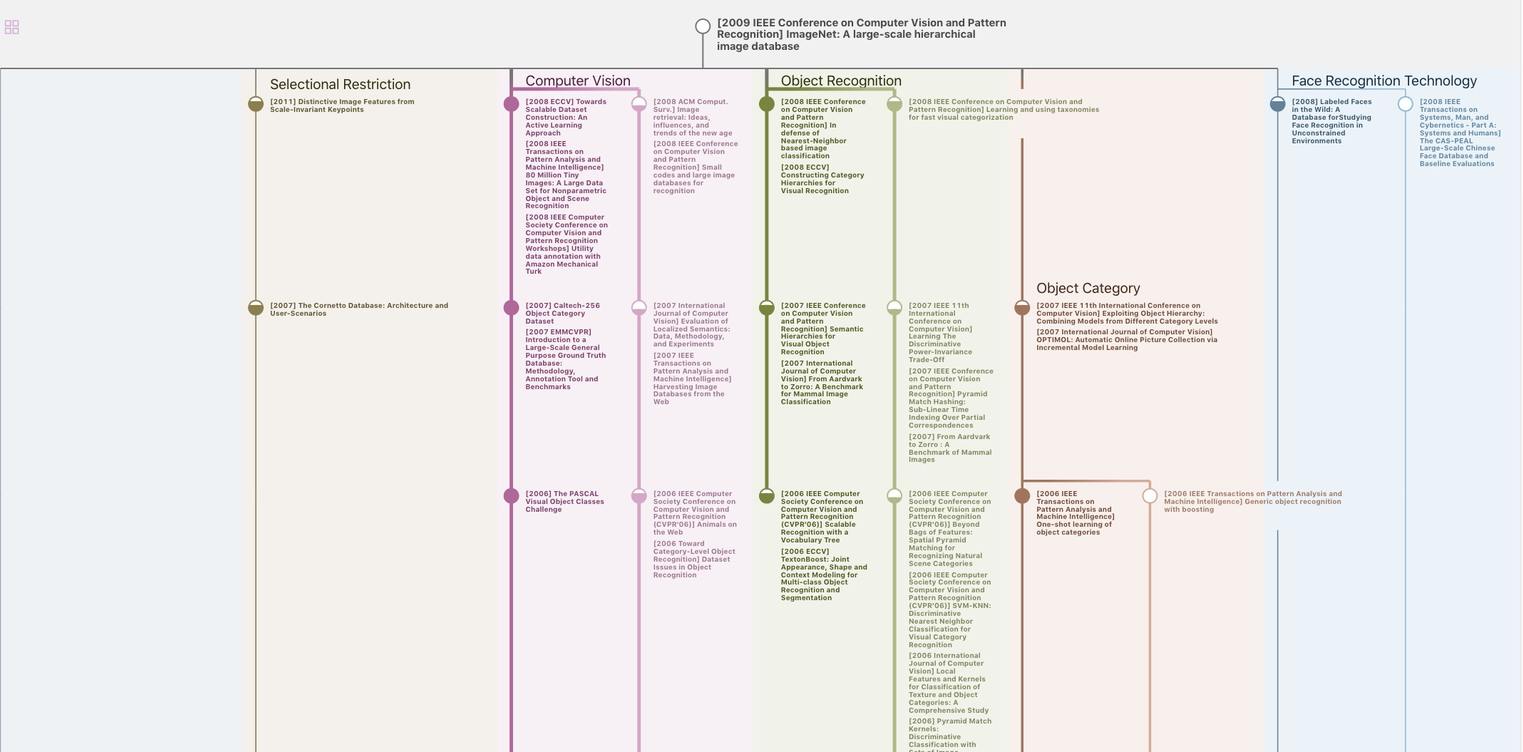
生成溯源树,研究论文发展脉络
Chat Paper
正在生成论文摘要