Lifted Auto-Context Forests for Brain Tumour Segmentation.
Lecture Notes in Computer Science(2016)
摘要
We revisit Auto-Context Forests for brain tumour segmentation in multi-channel magnetic resonance images, where semantic context is progressively built and refined via successive layers of Decision Forests (DFs). Specifically, we make the following contributions: (1) improved generalization via an efficient node-splitting criterion based on hold-out estimates, (2) increased compactness at a tree-level, thereby yielding shallow discriminative ensembles trained orders of magnitude faster, and (3) guided semantic bagging that exposes latent data-space semantics captured by forest pathways. The proposed framework is practical: the per-layer training is fast, modular and robust. It was a top performer in the MICCAI 2016 BRATS (Brain Tumour Segmentation) challenge, and this paper aims to discuss and provide details about the challenge entry.
更多查看译文
关键词
Brain Tumor Segmentation (BRATS), Multi-channel Magnetic Resonance Imaging, Decision Forests (DFs), BRATS Challenge, Enhancing Tumor Parts (ET)
AI 理解论文
溯源树
样例
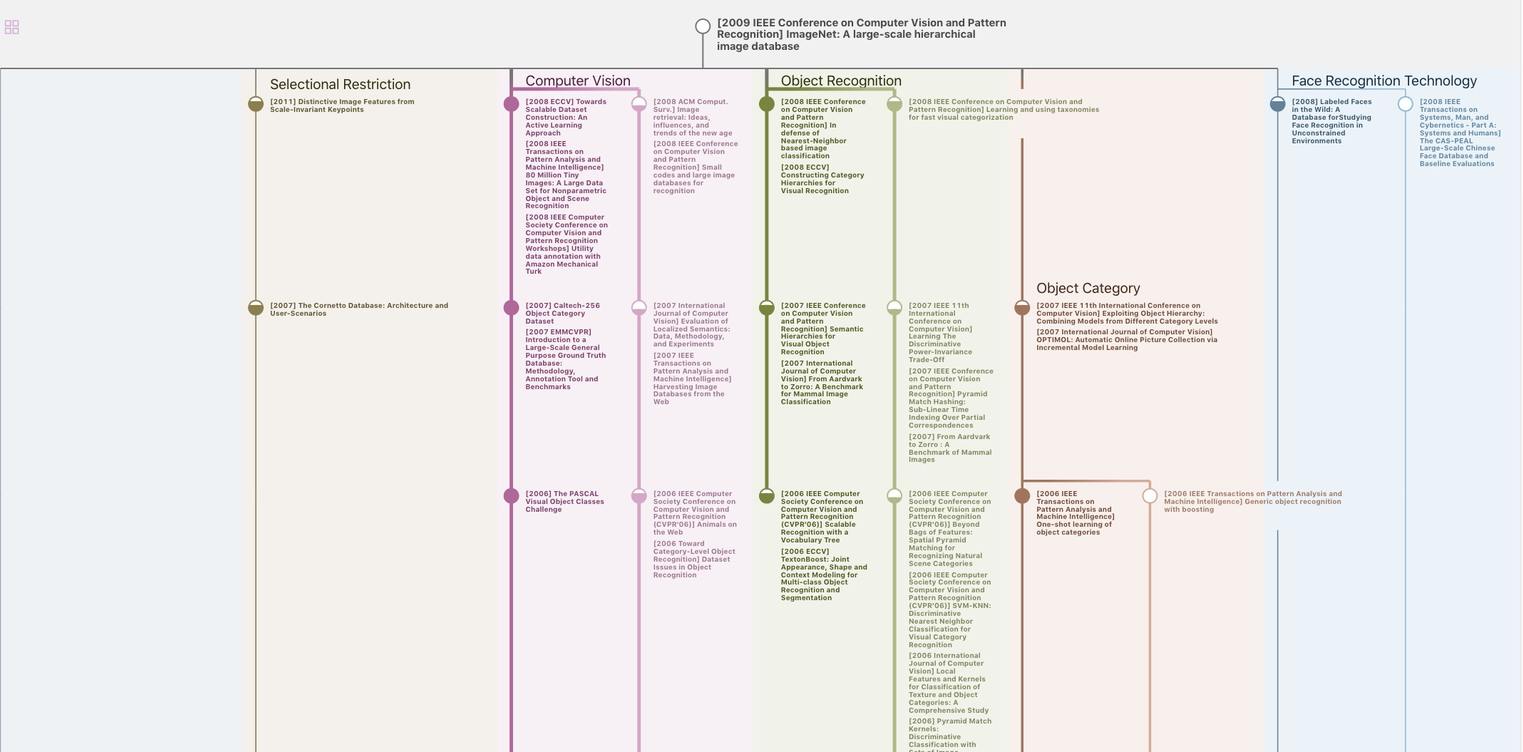
生成溯源树,研究论文发展脉络
Chat Paper
正在生成论文摘要