A Level-Wise Load Balanced Scientific Workflow Execution Optimization using NSGA-II.
CCGrid(2017)
摘要
Over the past decade, cloud computing has grown in popularity for the processing of scientific applications as a result of the scalability of the cloud and the ready availability of on-demand computing and storage resources. It is also a cost-effective alternative for scientific workflow executions with a pay-per-use paradigm. However, providing services with optimal performance at the lowest financial resource deployment cost is still challenging. Several fine-grained tasks are included in scientific workflow applications, and efficient execution of these tasks according to their processing dependency to minimize the overall makespan during workflow execution is an important research area. In this paper, a system for level-wise workflow makespan optimization and virtual machine deployment cost minimization for overall workflow optimization in cloud infrastructure is proposed. Further, balanced task clustering, to ensure load balancing in different virtual machine instances at each workflow level during workflow execution, is also considered. The system retrieves the necessary workflow information from a directed acyclic graph and uses the non-dominated sorting genetic algorithm II (NSGA-II) to carry out multiobjective optimization. Pareto front solutions obtained for makespan time and instance resource deployment cost for several scientific workflow applications verify the efficacy of our system.
更多查看译文
关键词
Scientific Workflow Applications, Cloud Computing, Multiobjective Optimization, NSGA-II
AI 理解论文
溯源树
样例
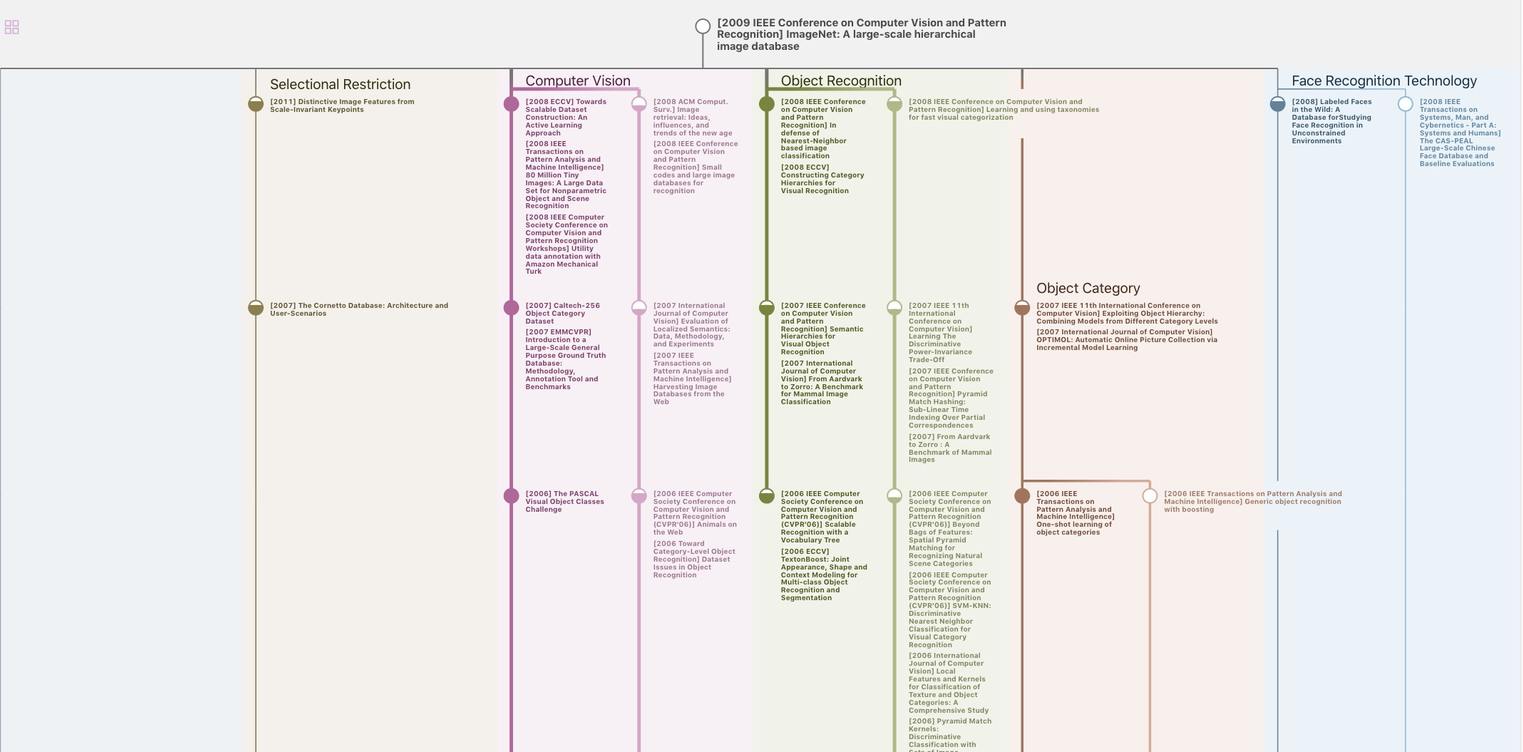
生成溯源树,研究论文发展脉络
Chat Paper
正在生成论文摘要