"Serial" versus "Parallel": A Comparison of Spatio-Temporal Clustering Approaches.
Lecture Notes in Artificial Intelligence(2017)
摘要
Spatio-temporal clustering, which is a process of grouping objects based on their spatial and temporal similarity, is increasingly gaining more scientific attention. Research in spatio-temporal clustering mainly focuses on approaches that use time and space in parallel. In this paper, we introduce a serial spatio-temporal clustering algorithm, called ST-DPOLY, which creates spatial clusters first and then creates spatio-temporal clusters by identifying continuing relationships between the spatial clusters in consecutive time frames. We compare this serial approach with a parallel approach named ST-SNN. Both ST-DPOLY and ST-SNN are density-based clustering approaches: while ST-DPOLY employs a density-contour based approach that operates on an actual density function, ST-SNN is based on well-established generic clustering algorithm Shared Nearest Neighbor (SNN). We demonstrate the effectiveness of these two approaches in a case study involving a New York city taxi trip dataset. The experimental results show that both ST-DPOLY and ST-SNN can find interesting spatio-temporal patterns in the dataset. Moreover, in terms of time and space complexity, ST-DPOLY has advantages over ST-SNN, while ST-SNN is more superior in terms of temporal flexibility; in terms of clustering results, results of ST-DPOLY are easier to interpret, while ST-SNN obtains more clusters which overlap with each other either spatially or temporally, which makes interpreting its clustering results more complicated.
更多查看译文
关键词
Spatio-temporal clustering,Cluster analysis,Batch processing,Shared nearest neighbor clustering,Density-based clustering
AI 理解论文
溯源树
样例
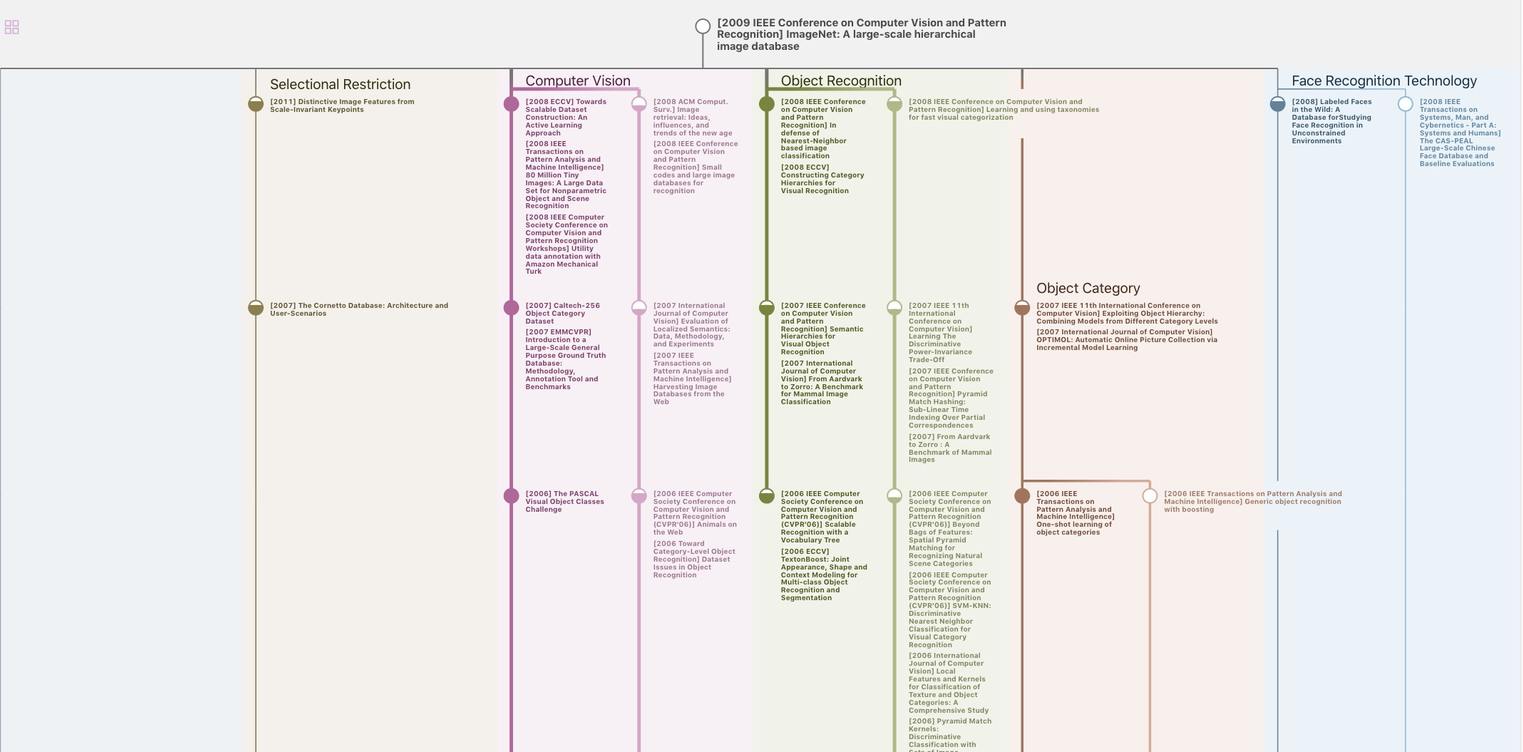
生成溯源树,研究论文发展脉络
Chat Paper
正在生成论文摘要