TIME: A Training-in-memory Architecture for Memristor-based Deep Neural Networks.
DAC(2017)
摘要
The training of neural network (NN) is usually time-consuming and resource intensive. Memristor has shown its potential in computation of NN. Especially for the metal-oxide resistive random access memory (RRAM), its crossbar structure and multi-bit characteristic can perform the matrix-vector product in high precision, which is the most common operation of NN. However, there exist two challenges on realizing the training of NN. Firstly, the current architecture can only support the inference phase of training and cannot perform the backpropagation (BP), the weights update of NN. Secondly, the training of NN requires enormous iterations and constantly updates the weights to reach the convergence, which leads to large energy consumption because of lots of write and read operations. In this work, we propose a novel architecture, TIME, and peripheral circuit designs to enable the training of NN in RRAM. TIME supports the BP and the weights update while maximizing the reuse of peripheral circuits for the inference operation on RRAM. Meanwhile, a variability-free tuning scheme and gradually-write circuits are designed to reduce the cost of tuning RRAM. We explore the performance of both SL (supervised learning) and DRL (deep reinforcement learning) in TIME, and a specific mapping method of DRL is also introduced to further improve the energy efficiency. Experimental results show that, in SL, TIME can achieve 5.3x higher energy efficiency on average compared with the most powerful application-specific integrated circuits (ASIC) in the literature. In DRL, TIME can perform averagely 126x higher than GPU in energy efficiency. If the cost of tuning RRAM can be further reduced, TIME have the potential of boosting the energy efficiency by 2 orders of magnitude compared with ASIC.
更多查看译文
关键词
energy efficiency,training-in-memory architecture,memristor,deep neural networks,metal-oxide resistive random access memory,RRAM
AI 理解论文
溯源树
样例
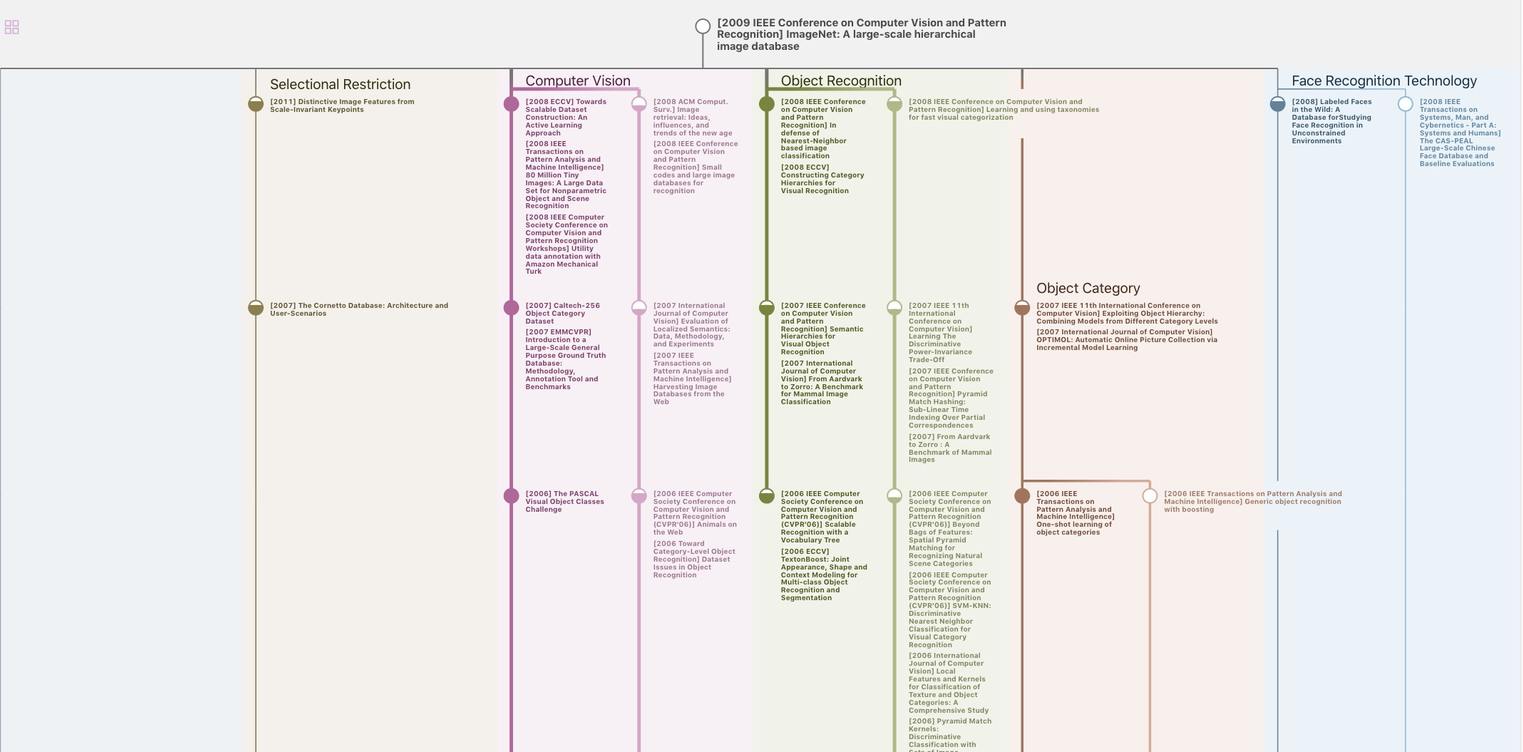
生成溯源树,研究论文发展脉络
Chat Paper
正在生成论文摘要