Multi-Label Classification Based on Low Rank Representation for Image Annotation.
REMOTE SENSING(2017)
摘要
Annotating remote sensing images is a challenging task for its labor demanding annotation process and requirement of expert knowledge, especially when images can be annotated with multiple semantic concepts (or labels). To automatically annotate these multi-label images, we introduce an approach called Multi-Label Classification based on Low Rank Representation (MLC-LRR). MLC-LRR firstly utilizes low rank representation in the feature space of images to compute the low rank constrained coefficient matrix, then it adapts the coefficient matrix to define a feature-based graph and to capture the global relationships between images. Next, it utilizes low rank representation in the label space of labeled images to construct a semantic graph. Finally, these two graphs are exploited to train a graph-based multi-label classifier. To validate the performance of MLC-LRR against other related graph-based multi-label methods in annotating images, we conduct experiments on a public available multi-label remote sensing images (Land Cover). We perform additional experiments on five real-world multi-label image datasets to further investigate the performance of MLC-LRR. Empirical study demonstrates that MLC-LRR achieves better performance on annotating images than these comparing methods across various evaluation criteria; it also can effectively exploit global structure and label correlations of multi-label images.
更多查看译文
关键词
remote sensing images,image annotation,multi label classification,low rank representation,graph construction,semantic graph
AI 理解论文
溯源树
样例
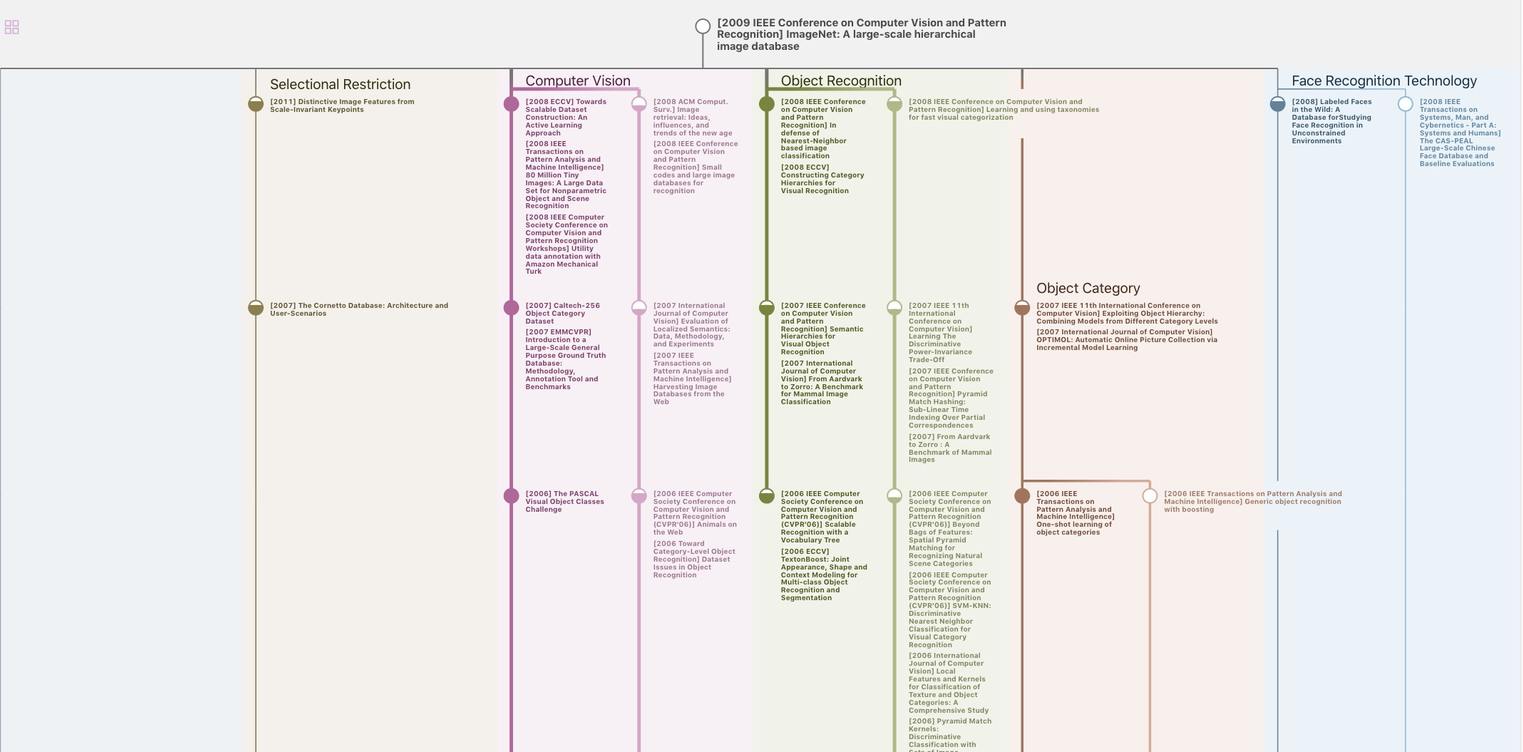
生成溯源树,研究论文发展脉络
Chat Paper
正在生成论文摘要