Multimodal Soft Nonnegative Matrix Co-Factorization for Convolutive Source Separation.
IEEE Trans. Signal Processing(2017)
摘要
In this paper, the problem of convolutive source separation via multimodal soft Nonnegative Matrix Co-Factorization (NMCF) is addressed. Different aspects of a phenomenon may be recorded by sensors of different types (e.g., audio and video of human speech), and each of these recorded signals is called a modality. Since the underlying phenomenon of the modalities is the same, they have some similarities. Especially, they usually have similar time changes. It means that changes in one of them usually correspond to changes in the other one. So their active or inactive periods are usually similar. Assuming this similarity, it is expected that the activation coefficient matrices of their Nonnegative Matrix Factorization (NMF) have a similar form. In this paper, the similarity of the activation coefficient matrices between the modalities is considered for co-factorization. This similarity is used for separation procedure in a soft manner by using penalty terms. This results in more flexibility in the separation procedure. Simulation results and comparison with state-of-the-art algorithms show the effectiveness of the proposed algorithm.
更多查看译文
关键词
Source separation,Lips,Radio frequency,Speech,Matrix decomposition,Spectrogram,Noise measurement
AI 理解论文
溯源树
样例
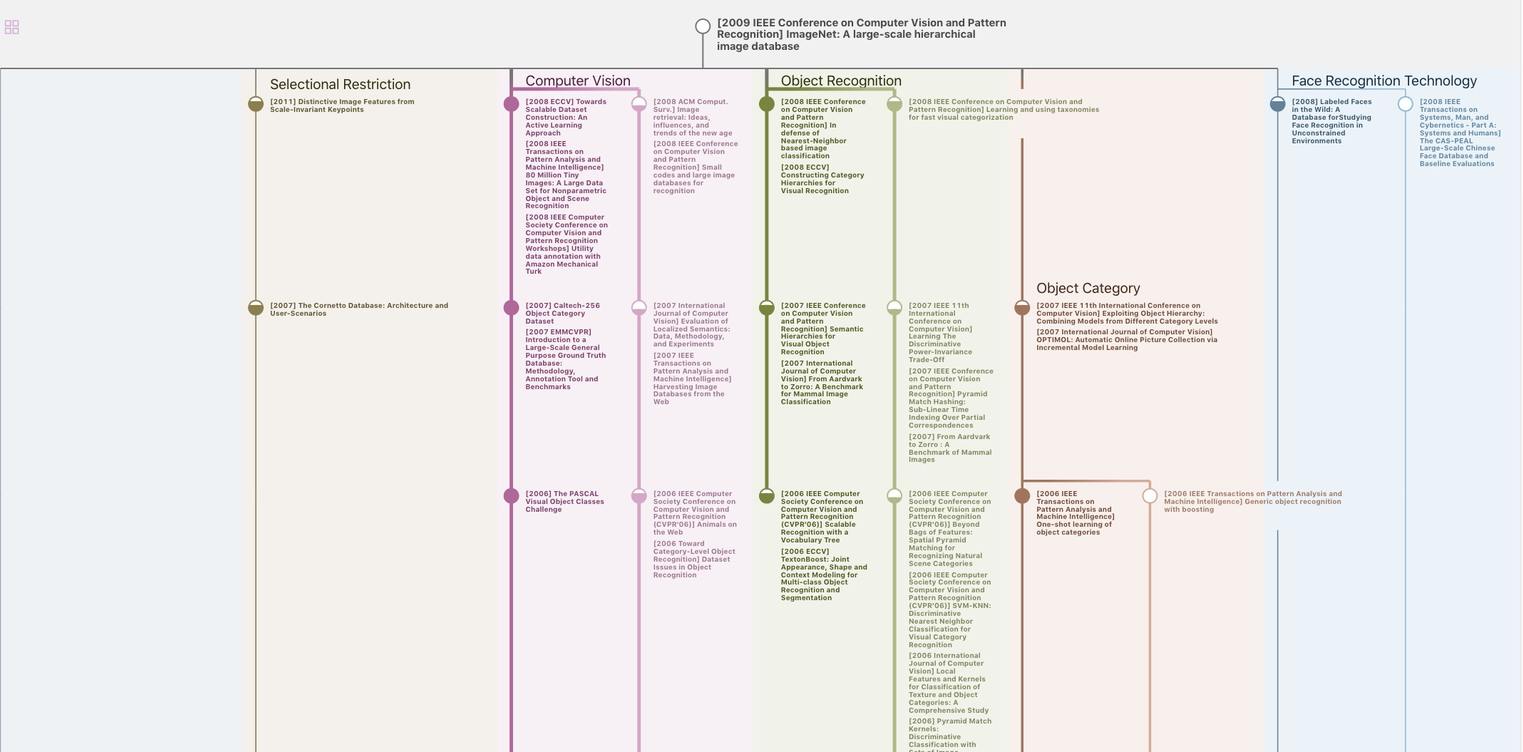
生成溯源树,研究论文发展脉络
Chat Paper
正在生成论文摘要