Deep Mixture of Diverse Experts for Large-Scale Visual Recognition.
IEEE transactions on pattern analysis and machine intelligence(2019)
摘要
A deep mixture of diverse experts algorithm is developed by seamlessly combining a set of base deep CNNs (convolutional neural networks) with diverse but overlapped task spaces (outputs) to generate a mixture network with larger outputs, e.g., these base deep CNNs are trained to recognize different subsets of tens of thousands of atomic object classes. One particular base deep CNNs with outputs is learned for each task group to recognize M atomic object classes and identify one special class of "not-in-group", where the network structure of the well-designed deep CNNs is directly used to configure such base deep CNNs. For M semantically-related atomic object classes in the same task group, a deep multi-task learning algorithm is developed to leverage their inter-class visual similarities to learn more discriminative base deep CNNs and multi-task softmax for enhancing their separability. All these base deep CNNs with diverse but overlapped task spaces are seamlessly combined to build a mixture network with larger outputs for recognizing tens of thousands of atomic object classes. Our experimental results have demonstrated that our deep mixture of diverse experts algorithm can achieve very competitive results on large-scale visual recognition.
更多查看译文
关键词
Task analysis,Visualization,Training,Complexity theory,Image recognition,Prediction algorithms,Diversity reception
AI 理解论文
溯源树
样例
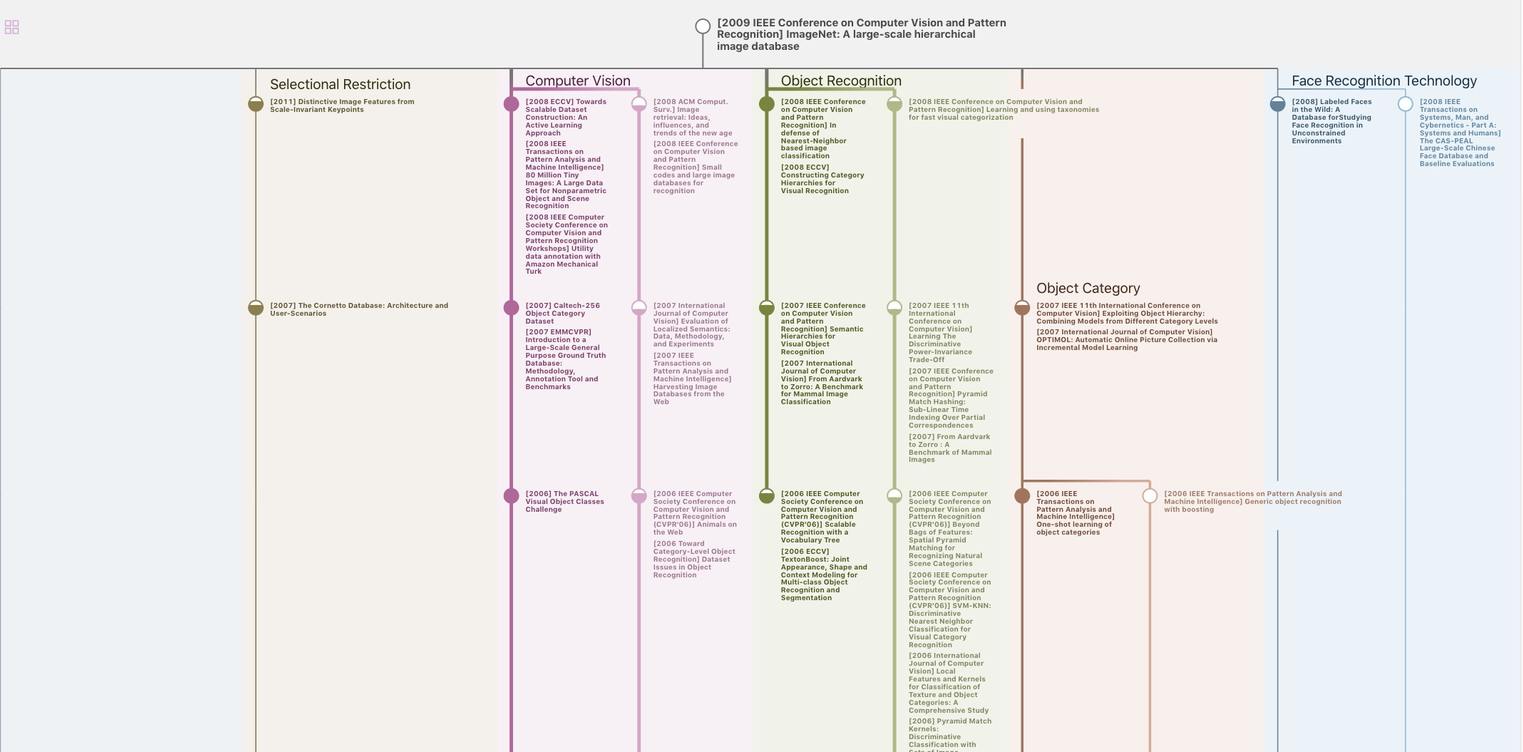
生成溯源树,研究论文发展脉络
Chat Paper
正在生成论文摘要