Dynamic Occupancy Grid Prediction for Urban Autonomous Driving: A Deep Learning Approach with Fully Automatic Labeling
2018 IEEE International Conference on Robotics and Automation (ICRA)(2017)
摘要
Long-term situation prediction plays a crucial role in the development of intelligent vehicles. A major challenge still to overcome is the prediction of complex downtown scenarios with multiple road users, e.g., pedestrians, bikes, and motor vehicles, interacting with each other. This contribution tackles this challenge by combining a Bayesian filtering technique for environment representation, and machine learning as long-term predictor. More specifically, a dynamic occupancy grid map is utilized as input to a deep convolutional neural network. This yields the advantage of using spatially distributed velocity estimates from a single time step for prediction, rather than a raw data sequence, alleviating common problems dealing with input time series of multiple sensors. Furthermore, convolutional neural networks have the inherent characteristic of using context information, enabling the implicit modeling of road user interaction. Pixel-wise balancing is applied in the loss function counteracting the extreme imbalance between static and dynamic cells. One of the major advantages is the unsupervised learning character due to fully automatic label generation. The presented algorithm is trained and evaluated on multiple hours of recorded sensor data and compared to Monte-Carlo simulation.
更多查看译文
关键词
complex interactions,dynamic occupancy grid prediction,urban autonomous driving,deep learning approach,long-term situation prediction,intelligent vehicles,complex downtown scenarios,multiple road users,motor vehicles,Bayesian filtering technique,environment representation,machine learning,deep convolutional neural network,spatially distributed velocity estimates,raw data sequence,input time series,multiple sensors,convolutional neural networks,road user interaction,pixel-wise balancing,static cells,dynamic cells,unsupervised learning character,pedestrians,bikes,distributed velocity estimation,Monte-Carlo simulation
AI 理解论文
溯源树
样例
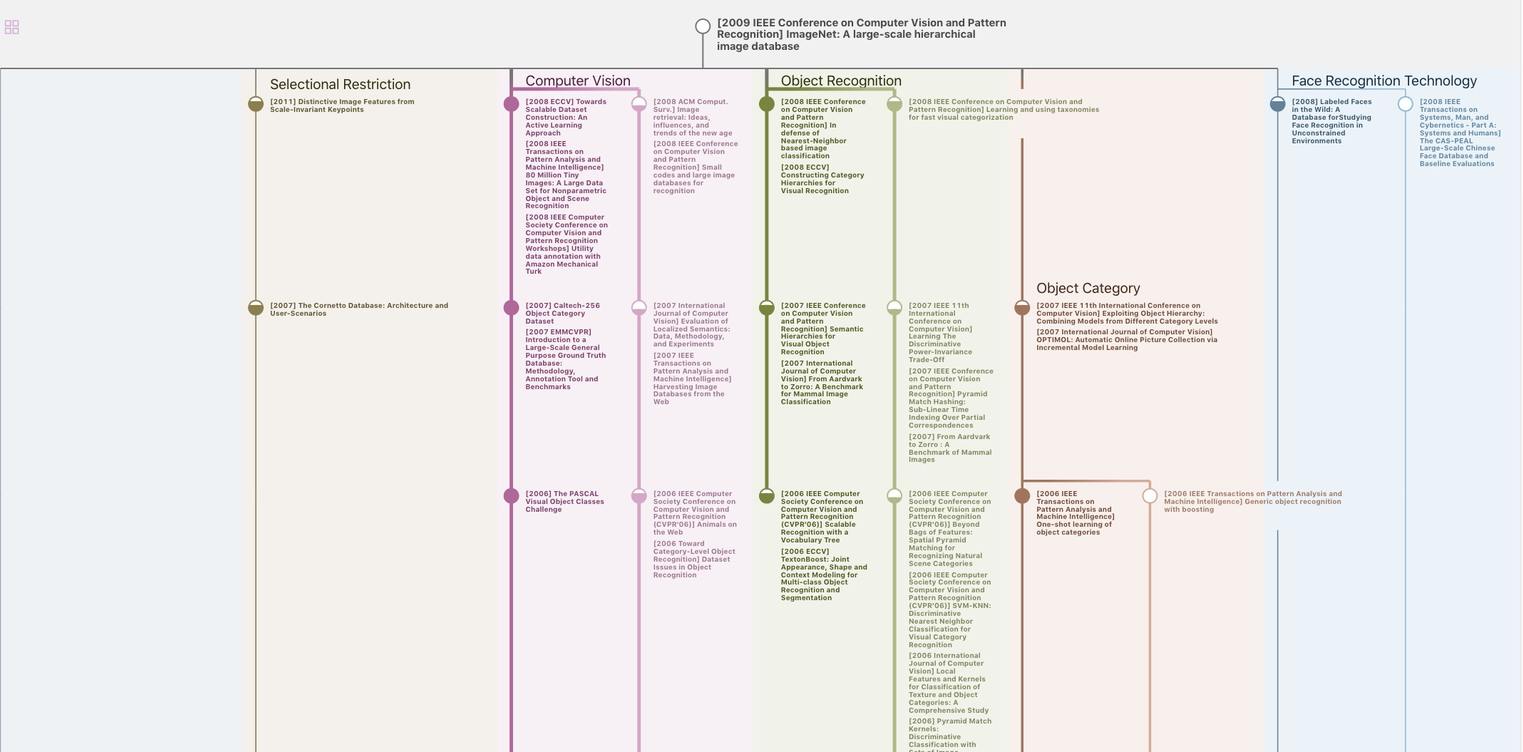
生成溯源树,研究论文发展脉络
Chat Paper
正在生成论文摘要