The Algorithmic LLL and the Witness Tree Lemma.
arXiv: Discrete Mathematics(2017)
摘要
We consider the recent works of cite{AIJACM,HV,Harmonic} that provide tools for analyzing focused stochastic local search algorithms that arise from algorithmizations of the Lovasz Local Lemma cite{LLL} (LLL) in general probability spaces. These are algorithms which search a state space probabilistically by repeatedly selecting a flaw that is currently present and moving to a random nearby state in an effort to address it and, eventually, reach a flawless state. While the original Moser-Tardos cite{MT} (MT) algorithm is amenable to the analysis of these abstract frameworks, many follow-up results cite{Haeupler_jacm,EnuHarris,szege_meet,determ,distributed,ParallelHarris} that further enhance, or exploit, our understanding of the MT process are not transferable to these general settings. Mainly, this is because a key ingredient of the original analysis of Moser and Tardos, the emph{witness tree lemma}, does not longer hold. In this work, we show that we can recover the witness tree lemma in the setting. The latter was recently introduced by Kolmogorov cite{Kolmofocs} and captures the vast majority of the LLL applications. Armed with it, we focus on studying properties of commutative algorithms and give several applications. Among other things, we are able to generalize and extend to the commutative setting the main result of cite{Haeupler_jacm} which states that the output of the MT algorithm well-approximates the conditional LLL-distribution, i.e., the distribution obtained by conditioning on all bad events being avoided.
更多查看译文
AI 理解论文
溯源树
样例
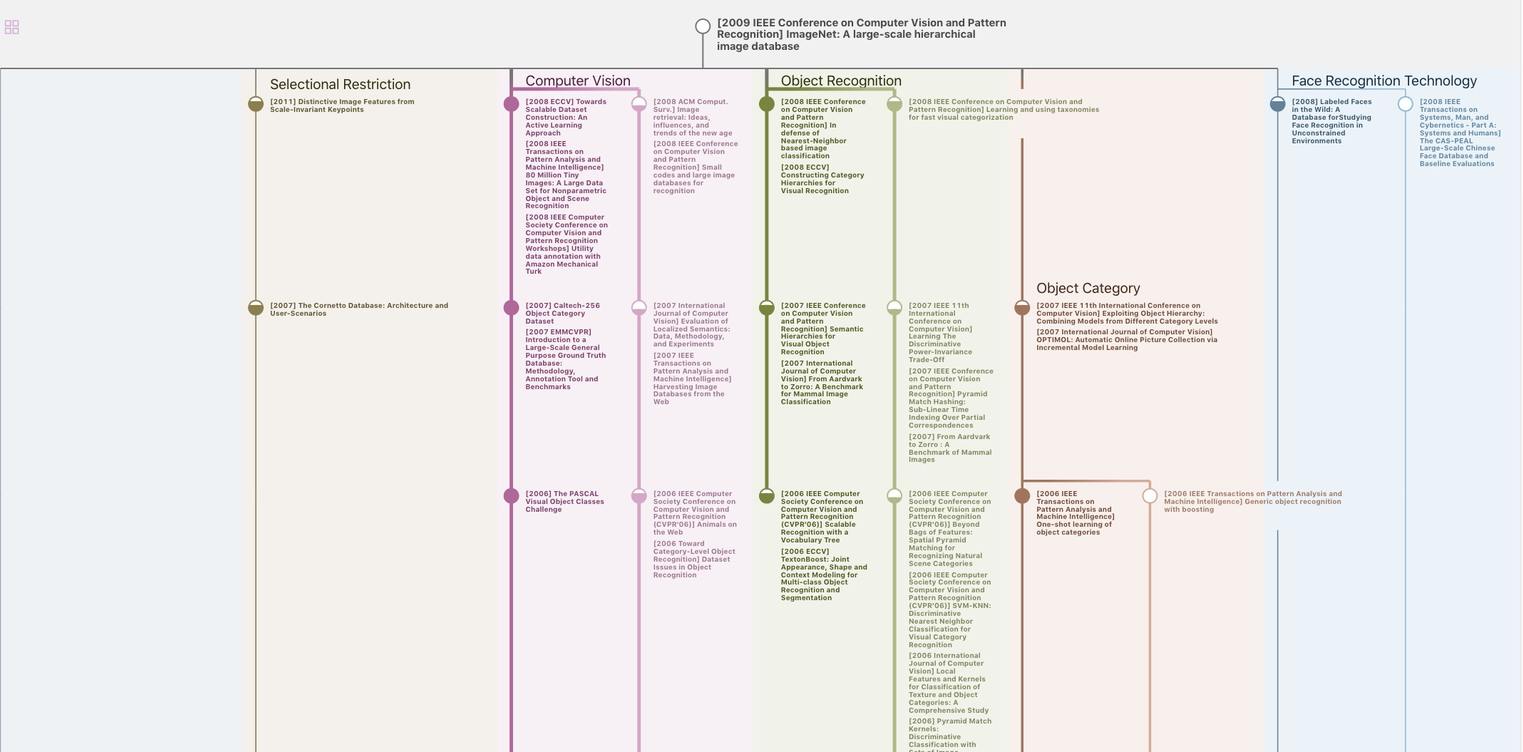
生成溯源树,研究论文发展脉络
Chat Paper
正在生成论文摘要