Concentration Bounds for High Sensitivity Functions Through Differential Privacy.
arXiv: Learning(2017)
摘要
A new line of work demonstrates how differential privacy can be used as a mathematical tool for guaranteeing generalization in adaptive data analysis. Specifically, if a differentially private analysis is applied on a sample S of i.i.d. examples to select a low-sensitivity function f, then w.h.p. f(S) is close to its expectation, even though f is being chosen adaptively, i.e., based on the data.Very recently, Steinke and Ullman observed that these generalization guarantees can be used for proving concentration bounds in the non-adaptive setting, where the low-sensitivity function is fixed beforehand. In particular, they obtain alternative proofs for classical concentration bounds for low-sensitivity functions, such as the Chernoff bound and McDiarmidu0027s Inequality. In this work, we extend this connection between differential privacy and concentration bounds, and show that differential privacy can be used to prove concentration of high-sensitivity functions.
更多查看译文
关键词
Differential privacy, concentration bounds, high sensitivity functions
AI 理解论文
溯源树
样例
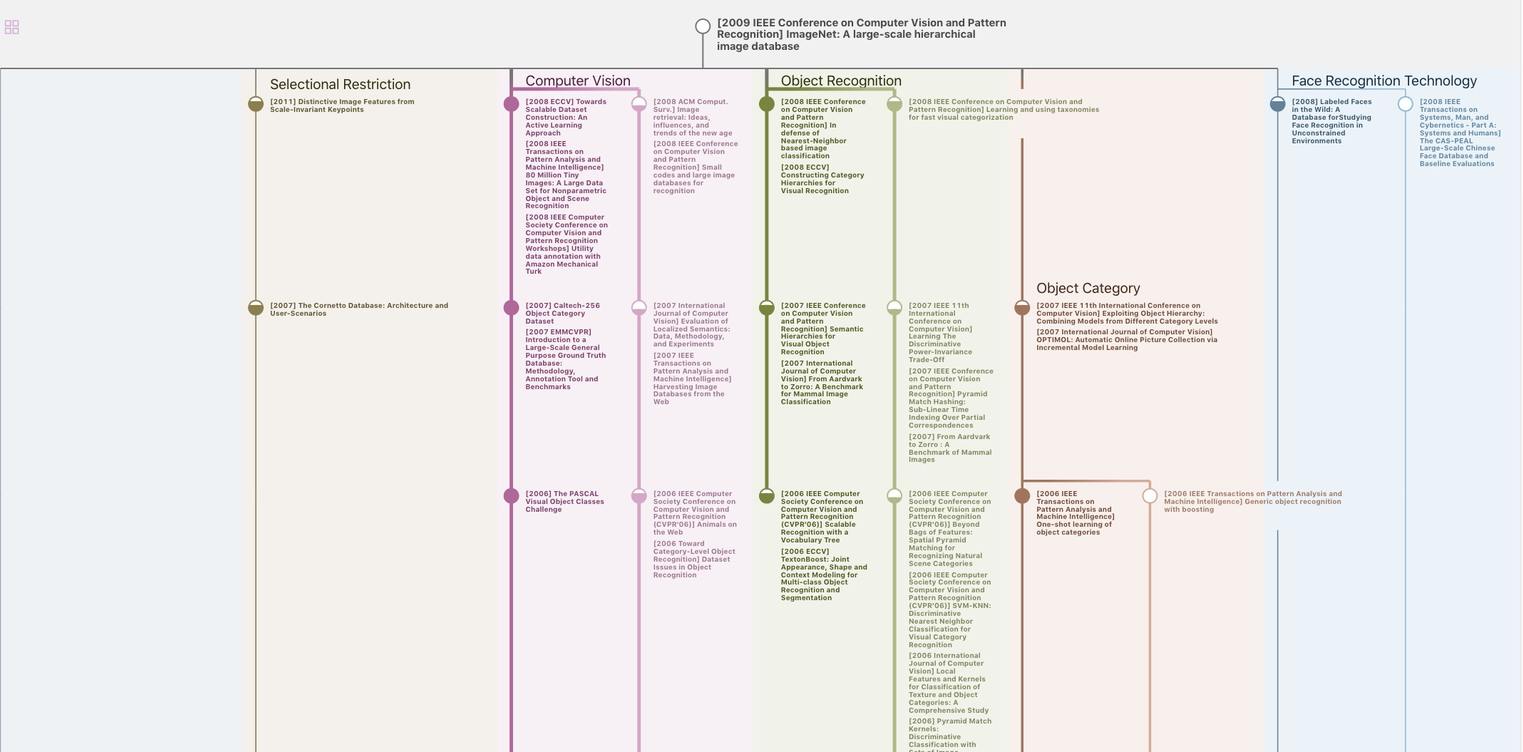
生成溯源树,研究论文发展脉络
Chat Paper
正在生成论文摘要