Event Classification in Microblogs via Social Tracking.
ACM TIST(2017)
摘要
Social media websites have become important information sharing platforms. The rapid development of social media platforms has led to increasingly large-scale social media data, which has shown remarkable societal and marketing values. There are needs to extract important events in live social media streams. However, microblogs event classification is challenging due to two facts, i.e., the short/conversational nature and the incompatible meanings between the text and the corresponding image in social posts, and the rapidly evolving contents. In this article, we propose to conduct event classification via deep learning and social tracking. First, we introduce a Multi-modal Multi-instance Deep Network (M2DN) for microblogs classification, which is able to handle the weakly labeled microblogs data oriented from the incompatible meanings inside microblogs. Besides predicting each microblogs as predefined events, we propose to employ social tracking to extract social-related auxiliary information to enrich the testing samples. We extract a set of candidate-relevant microblogs in a short time window by using social connections, such as related users and geographical locations. All these selected microblogs and the testing data are formulated in a Markov Random Field model. The inference on the Markov Random Field is conducted to update the classification results of the testing microblogs. This method is evaluated on the Brand-Social-Net dataset for classification of 20 events. Experimental results and comparison with the state of the arts show that the proposed method can achieve better performance for the event classification task.
更多查看译文
关键词
Event classification,multi-modal,multi-instance,social tracking,Markov Random Field (MRF)
AI 理解论文
溯源树
样例
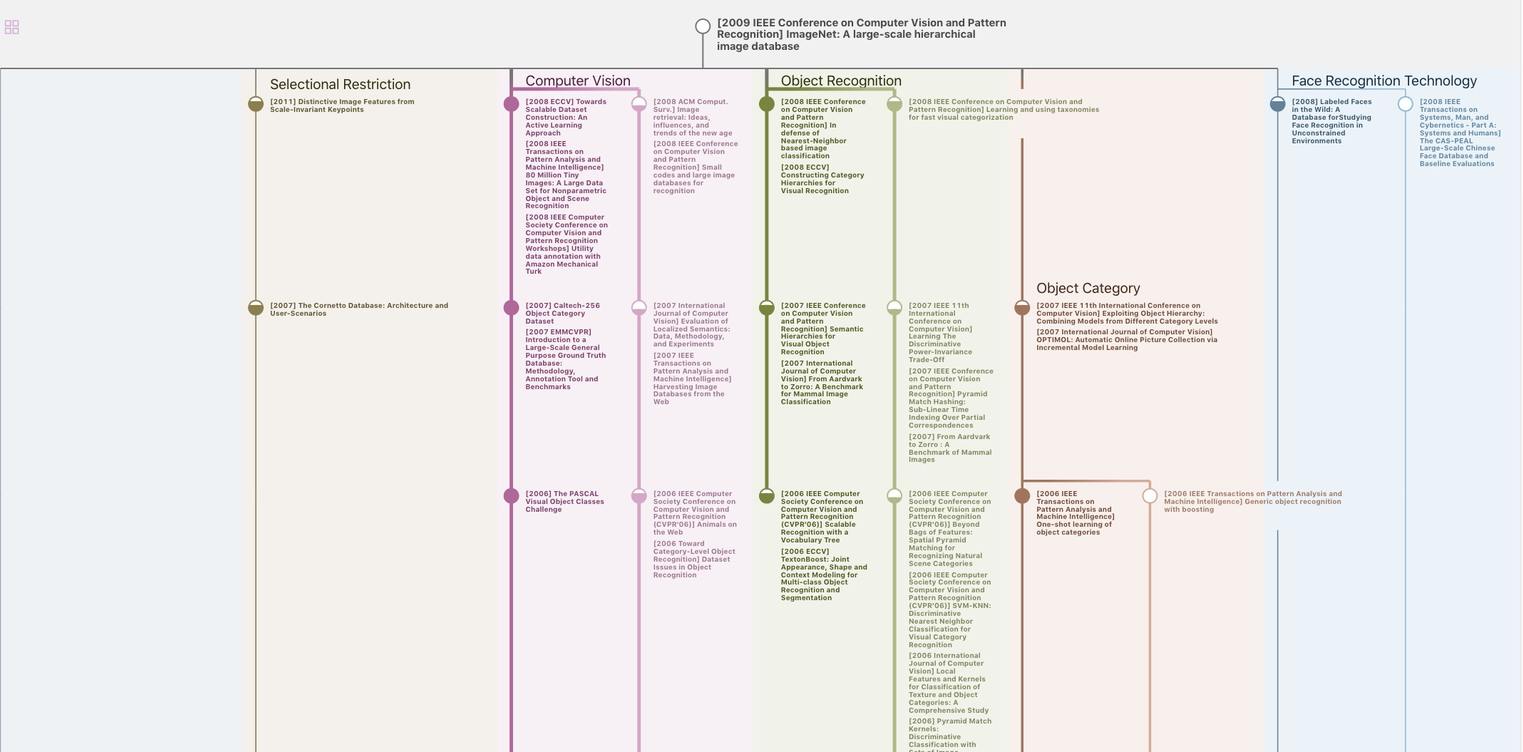
生成溯源树,研究论文发展脉络
Chat Paper
正在生成论文摘要