A Novel Approach for Relation Extraction with Few Labeled Data.
Communications in Computer and Information Science(2016)
摘要
Lack of large scale training data is a challenge for conventional supervised relation extraction approach. Although distant supervision has been proposed to address this issue, it suffers from massive noise and the trained model cannot be applied to unseen relations. We present a novel approach for relation extraction which uses the relation definition as a guide and only needs a hundred of high-quality mention examples for training model. In detail, we classify the candidate mention of a specific relation by judging whether the mention is in conformity with the relation's definition through measuring the semantic relevance between the definition and the mention. Our approach is insensitive to class-imbalance problem. And the trained model can be directly applied to classify mentions of newly defined relation without labeling new training data. Experimental results demonstrate that our approach achieves competitive performance and can be incorporated with existing approaches to boost performance.
更多查看译文
关键词
Parse Tree, Positive Instance, Negative Instance, Relation Extraction, Relation Definition
AI 理解论文
溯源树
样例
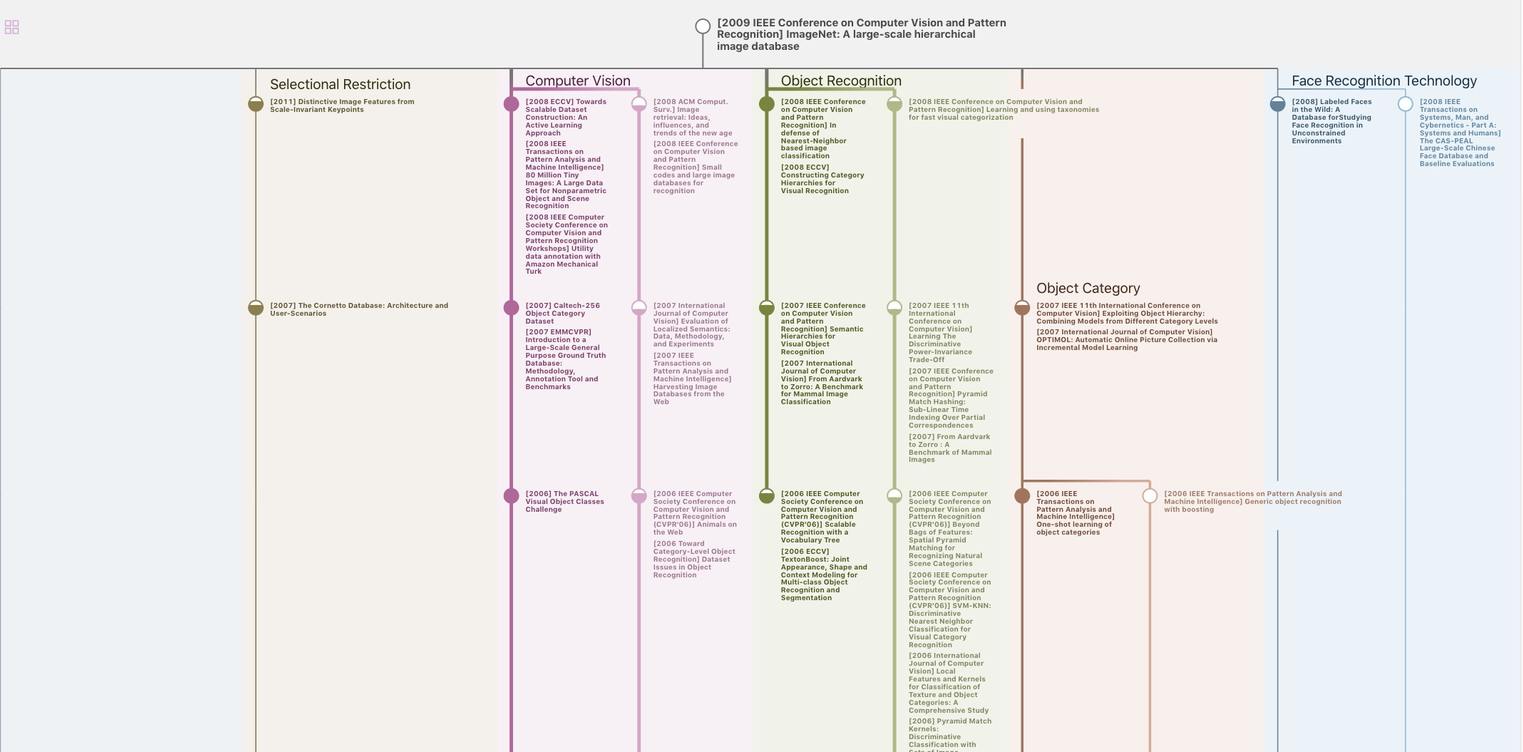
生成溯源树,研究论文发展脉络
Chat Paper
正在生成论文摘要