Sporadic Decision-Centric Data Scheduling with Normally-off Sensors
2016 IEEE Real-Time Systems Symposium (RTSS)(2016)
摘要
The Internet of Things heralds a new generation of data-centric applications, where controllers connect to large numbers of heterogeneous sensing devices. We consider a model, where the control loop does not execute periodically. Instead, controllers are prompted by contextual cues to make one-off decisions, resulting in sporadic activations. Since the need for data arises only sporadically, sensors do not sample data continuously. Rather, they are normally off (e.g., to save energy), but are activated by the controller on demand, when data is needed. Collected data has validity intervals, after which it must be re-sampled, since the measured value may change. Once a decision is made based on the data, sensors are turned off again. We call this model sporadic decision-centric data scheduling with normally-off sensors. It gives rise to novel scheduling problems because of the way the timing of activation of different sensors affects load attributed to data sampling; the shorter the interval between activation of a given sensor and the time a corresponding decision is made, the lower the number of samples taken by that sensor to support the decision, and thus decision cost. The paper defines the aforementioned decision-centric data scheduling problem and derives the optimal scheduling policy, called EDEF-LVF, for this task model. Simulation results confirm the superiority of EDEF-LVF compared to several baselines.
更多查看译文
关键词
Internet of Things,IoT,data,disaster response,smart city,decision,validity,freshness
AI 理解论文
溯源树
样例
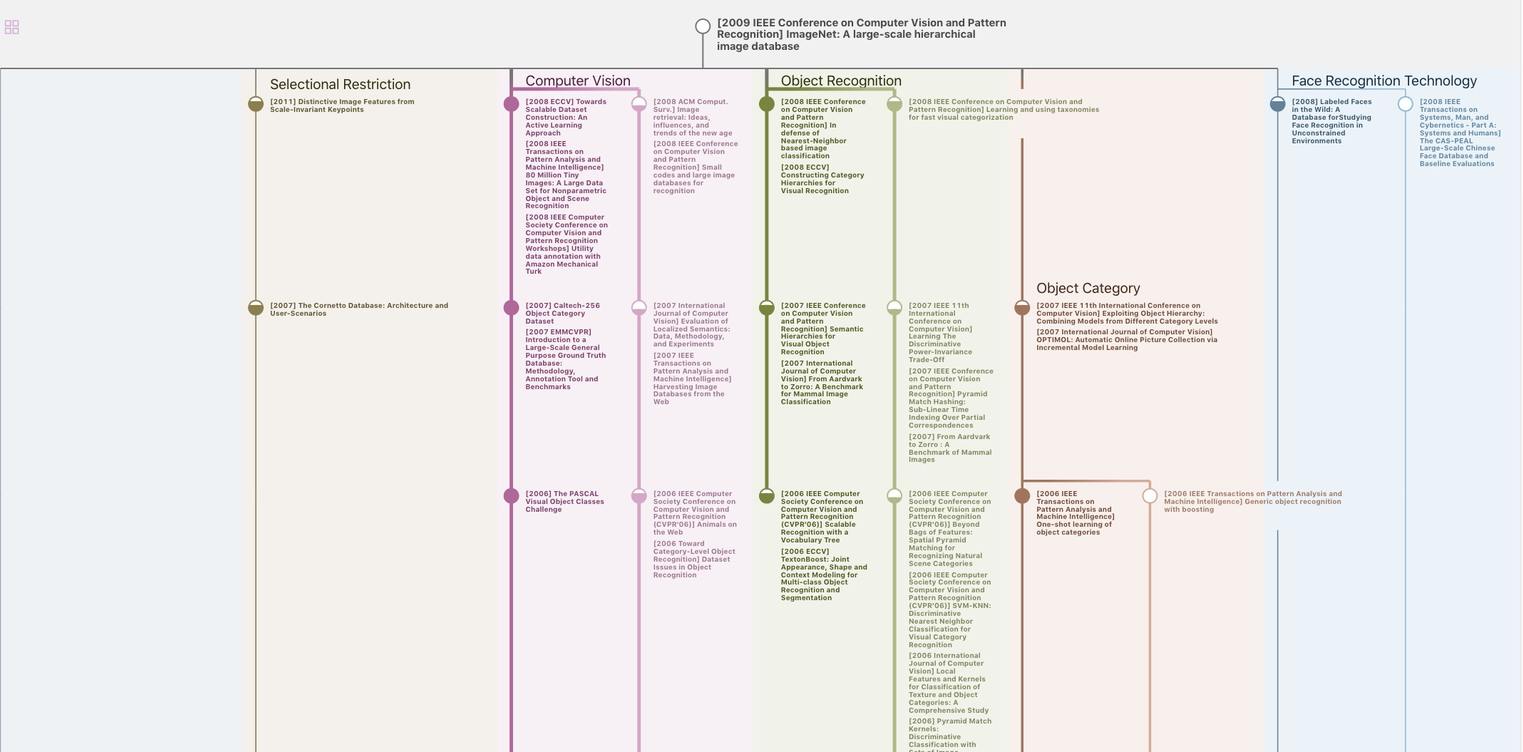
生成溯源树,研究论文发展脉络
Chat Paper
正在生成论文摘要