Action Recognition Using Convolutional Restricted Boltzmann Machines.
ICMR'16: International Conference on Multimedia Retrieval New York New York USA June, 2016(2016)
摘要
In this work we study deep learning architectures for the problem of action recognition in image sequences focusing on generative neural networks, namely the convolutional extension of restricted Boltzmann machines (RBMs). We first use a stack of convolutional restricted Boltzmann machines to learn and extract features from sequences of images in an unsupervised way, and then use them for the task of action classification. We modify the energy function of the convolutional RBM in such a way that the training updates reported in the literature follow directly from the differentiation of the objective function, which we define in terms of the free energy function. This is in contrast to other works on convolutional RBMs in the literature whose update equations do not directly follow from a well defined energy function or optimization framework without any ad hoc normalizations. We show that the representations that are derived from unsupervised training of the RBMs have very similar or better descriptive power than hand-designed image descriptors and give competitive performance in the problem of action recognition.
更多查看译文
AI 理解论文
溯源树
样例
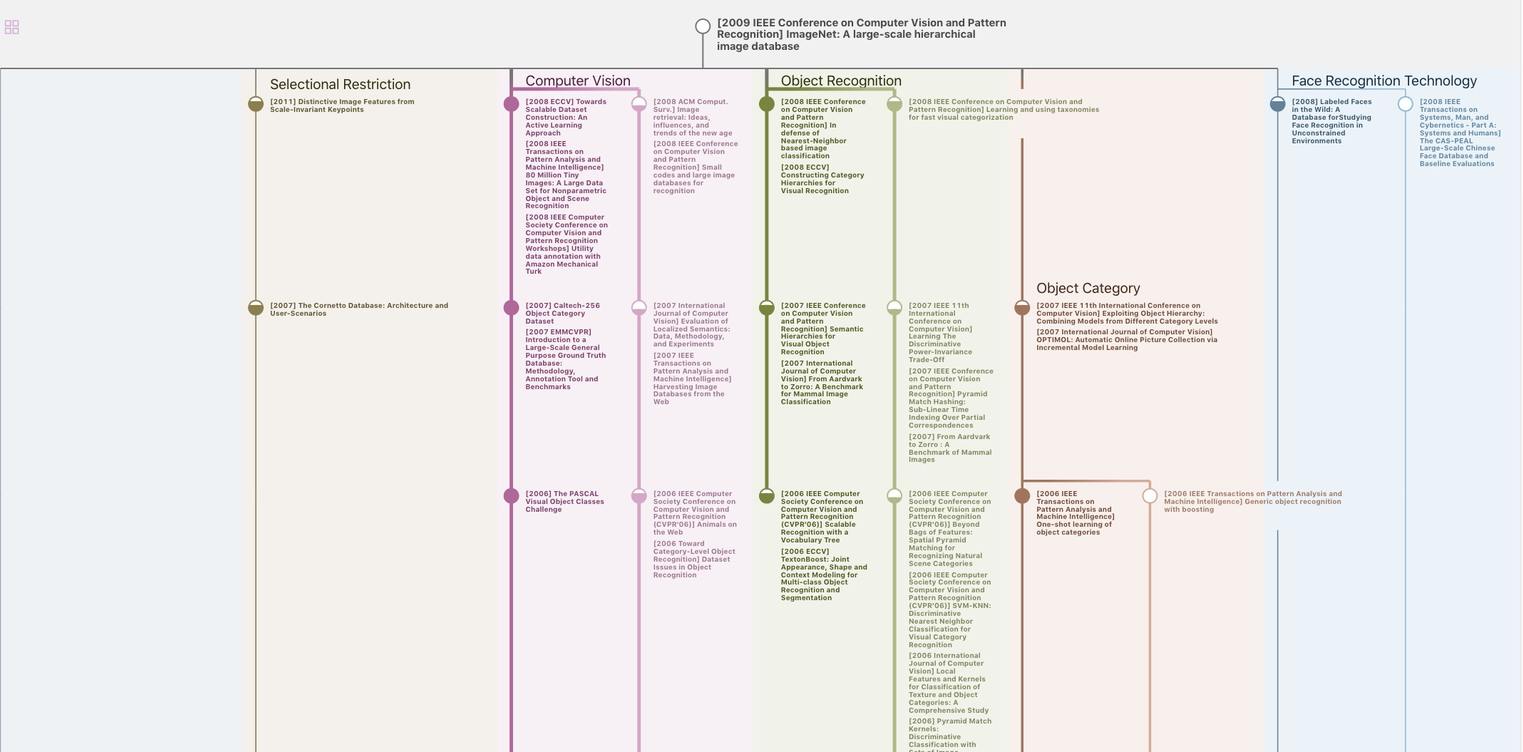
生成溯源树,研究论文发展脉络
Chat Paper
正在生成论文摘要