The influence of feature vector on the classification of mechanical faults using neural networks
2016 IEEE 7th Latin American Symposium on Circuits & Systems (LASCAS)(2016)
摘要
This paper investigates the problem of automatic detection of rotating-machine faults based on vibration signals acquired during machine operation. In particular, two new signal features, namely the kurtosis and entropy, are considered along with main spectral peaks to discriminate between several machine conditions: normal operation, (vertical and horizontal) misalignment, unbalanced load and bearing faults. Moreover, the inclusion of one set of three accelerometers for each roller bearing associated to the system acquiring more vibration signals also affects the generation of feature vector and is part of our proposal. In order to evaluate the rotating machine fault classification, a database of 1951 fault scenarios with several different fault intensities and rotating frequencies was designed and recorded, taking into consideration the specificities of the proposed machine learning task. The artificial neural networks recognition system employed in this work reached 95.8% of overall accuracy, showing the efficiency of the proposed approach.
更多查看译文
关键词
Rotating machine,pattern classification,fault diagnosis,feature extraction
AI 理解论文
溯源树
样例
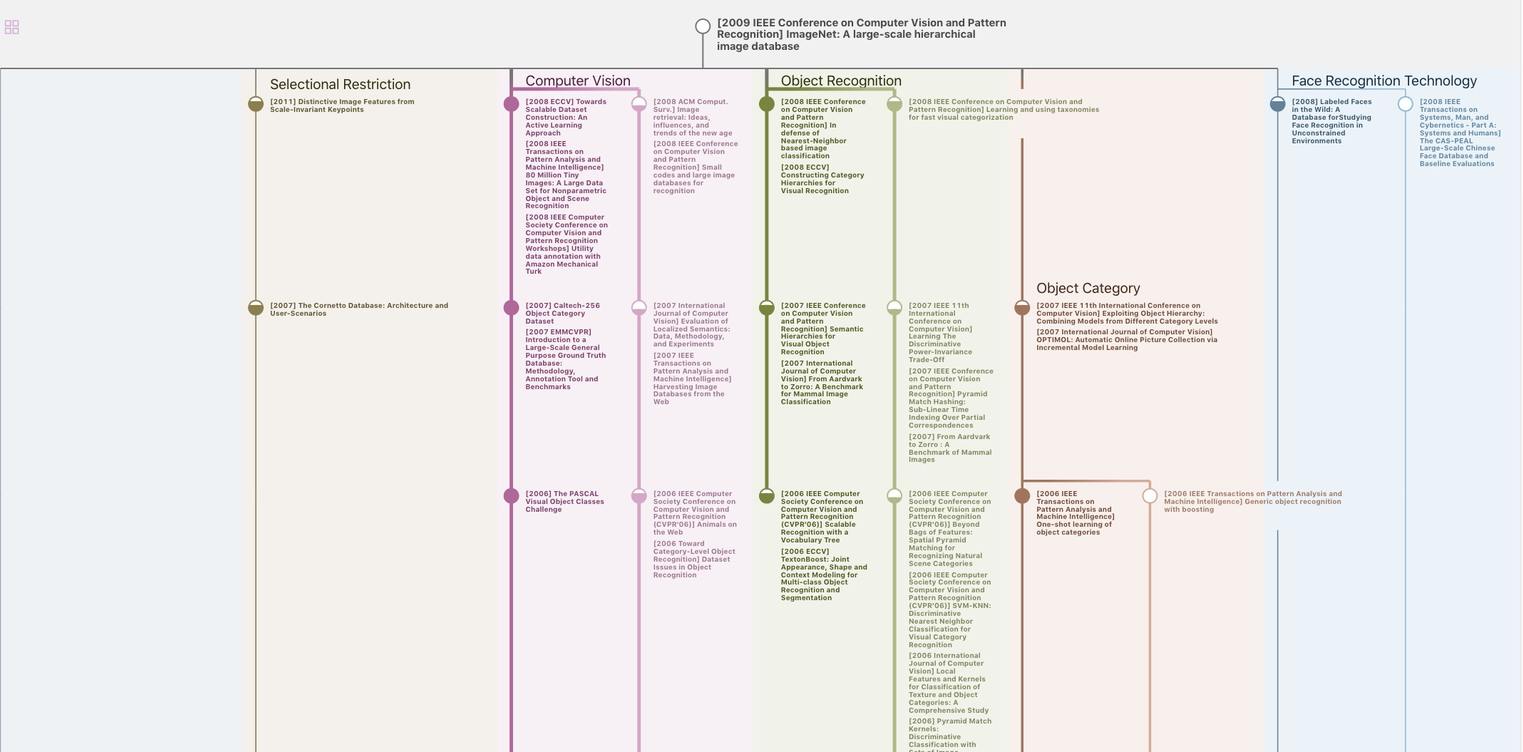
生成溯源树,研究论文发展脉络
Chat Paper
正在生成论文摘要