Aligned Matrix Completion: Integrating Consistency and Independency in Multiple Domains
2016 IEEE 16th International Conference on Data Mining (ICDM)(2016)
摘要
Matrix completion is the task of recovering a data matrix from a sample of entries, and has received significant attention in theory and practice. Normally, matrix completion considers a single matrix, which can be a noisy image or a rating matrix in recommendation. In practice however, data is often obtained from multiple domains rather than a single domain. For example, in recommendation, multiple matrices may exist as user x movie and user x book, while correlations among the multiple domains can be reasonably exploited to improve the quality of matrix completion. In this paper, we consider the problem of aligned matrix completion, where multiple matrices are recovered that correspond to different representations of the same group of objects. In the proposed model, we maintain consistency of multiple domains with a shared latent structure, while allowing independent patterns for each separate domain. In addition, we impose the low-rank structure of a matrix with a novel regularizer which provides better approximation than the standard nuclear norm relaxation.
更多查看译文
关键词
aligned matrix completion,data matrix,noisy image,rating matrix,low-rank structure,multidomain recommendation task
AI 理解论文
溯源树
样例
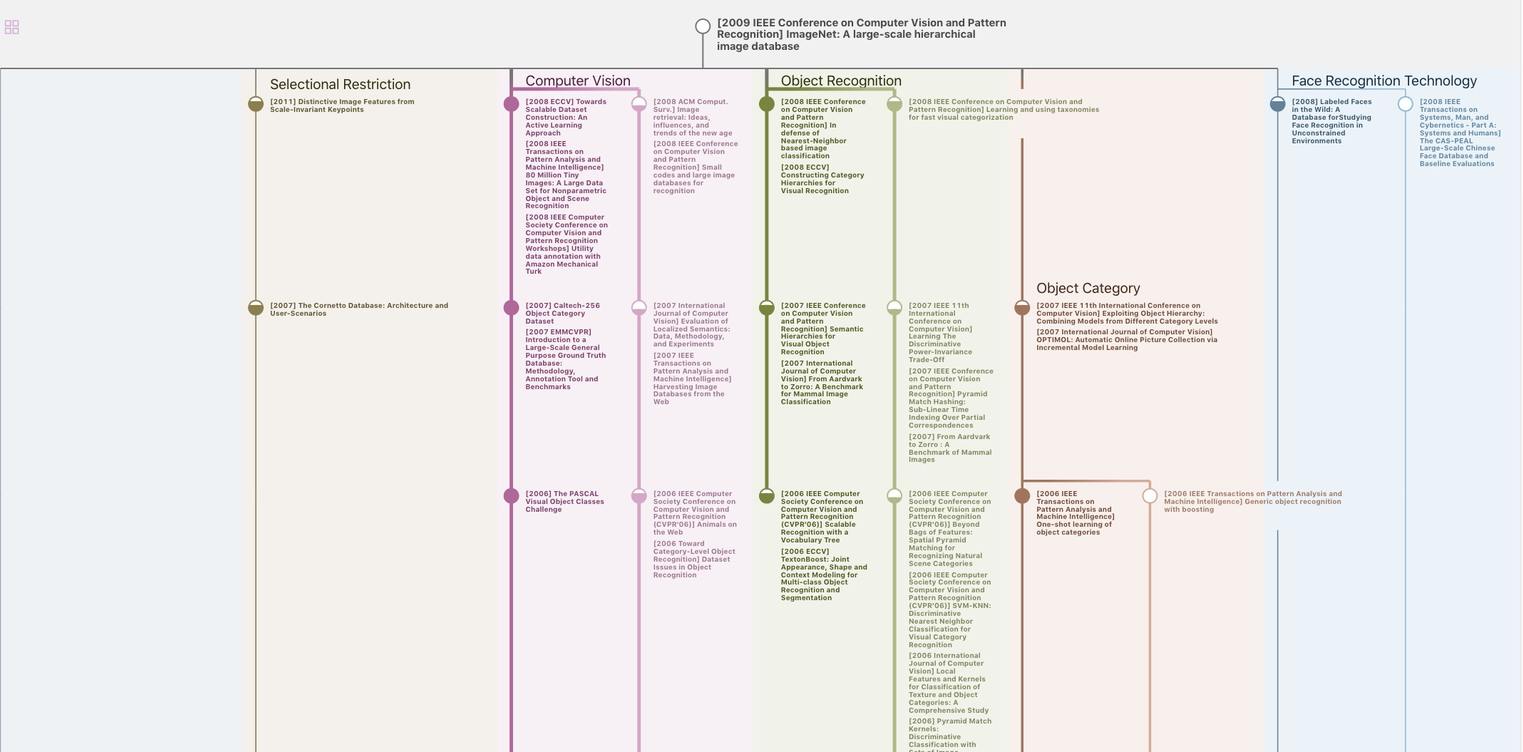
生成溯源树,研究论文发展脉络
Chat Paper
正在生成论文摘要