Exploiting Visual-Spatial First-Person Co-Occurrence for Action-Object Detection without Labels.
arXiv: Computer Vision and Pattern Recognition(2016)
摘要
Many first-person vision tasks such as activity recognition or video summarization requires knowing, which objects the camera wearer is interacting with (i.e. action-objects). The standard way to obtain this information is via a manual annotation, which is costly and time consuming. Also, whereas for the third-person tasks such as object detection, the annotator can be anybody, action-object detection task requires the camera wearer to annotate the data because a third-person may not know what the camera wearer was thinking. Such a constraint makes it even more difficult to obtain first-person annotations. To address this problem, we propose a Visual-Spatial Network (VSN) that detects action-objects without using any first-person labels. We do so (1) by exploiting the visual-spatial co-occurrence in the first-person data and (2) by employing an alternating cross-pathway supervision between the visual and spatial pathways of our VSN. During training, we use a selected action-object prior location to initialize the pseudo action-object ground truth, which is then used to optimize both pathways in an alternating fashion. The predictions from the spatial pathway are used to update the pseudo ground truth for the visual pathway and vice versa, which allows both pathways to improve each other. We show our methodu0027s success on two different action-object datasets, where our method achieves similar or better results than the supervised methods. We also show that our method can be successfully used as pretraining for a supervised action-object detection task.
更多查看译文
AI 理解论文
溯源树
样例
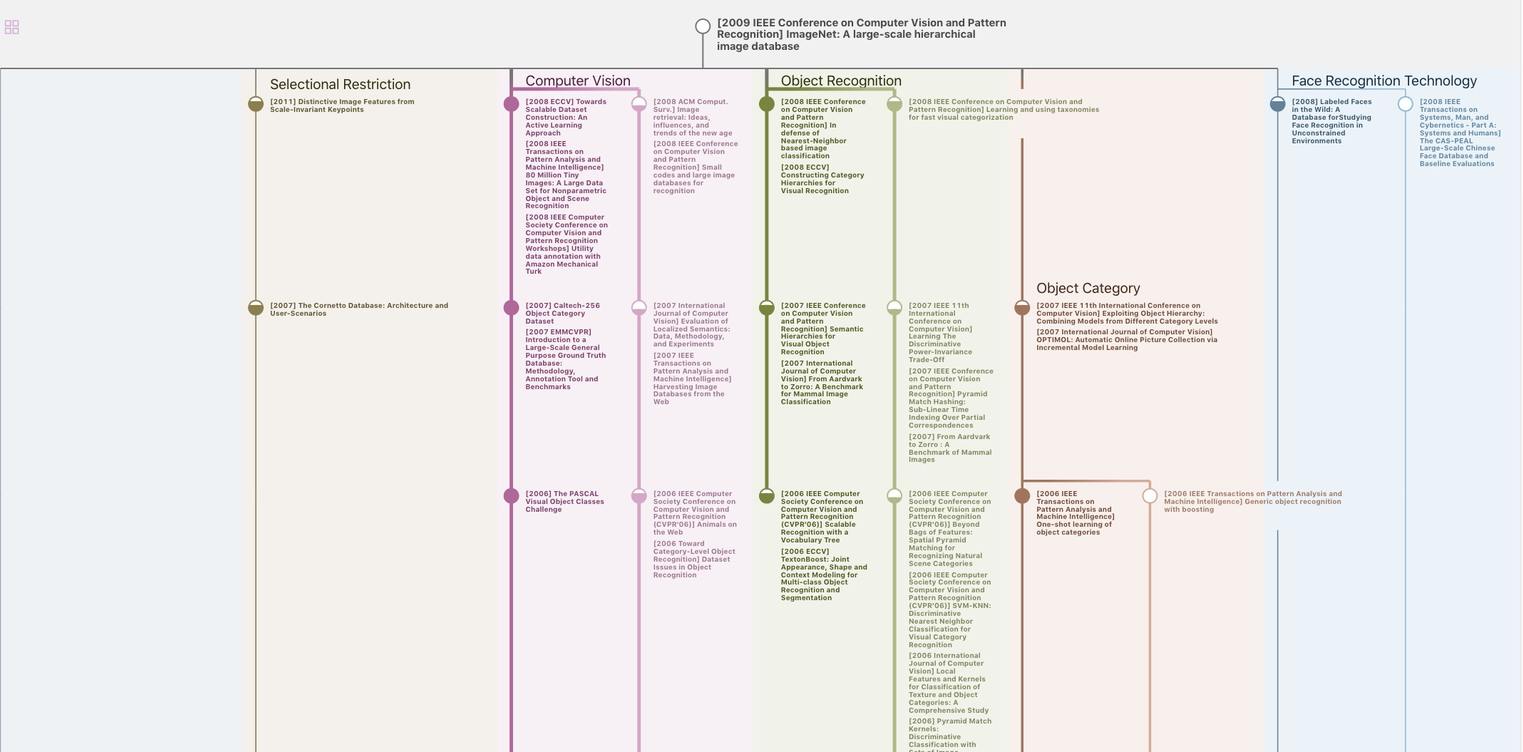
生成溯源树,研究论文发展脉络
Chat Paper
正在生成论文摘要