Sampling from Social Networks with Attributes
WWW '17: 26th International World Wide Web Conference Perth Australia April, 2017(2017)
摘要
Sampling from large networks represents a fundamental challenge for social network research. In this paper, we explore the sensitivity of different sampling techniques (node sampling, edge sampling, random walk sampling, and snowball sampling) on social networks with attributes. We consider the special case of networks (i) where we have one attribute with two values (e.g., male and female in the case of gender), (ii) where the size of the two groups is unequal (e.g., a male majority and a female minority), and (iii) where nodes with the same or different attribute value attract or repel each other (i.e., homophilic or heterophilic behavior). We evaluate the different sampling techniques with respect to conserving the position of nodes and the visibility of groups in such networks. Experiments are conducted both on synthetic and empirical social networks. Our results provide evidence that different network sampling techniques are highly sensitive with regard to capturing the expected centrality of nodes, and that their accuracy depends on relative group size differences and on the level of homophily that can be observed in the network. We conclude that uninformed sampling from social networks with attributes thus can significantly impair the ability of researchers to draw valid conclusions about the centrality of nodes and the visibility or invisibility of groups in social networks.
更多查看译文
关键词
social networks,sampling methods,sampling bias,homophily
AI 理解论文
溯源树
样例
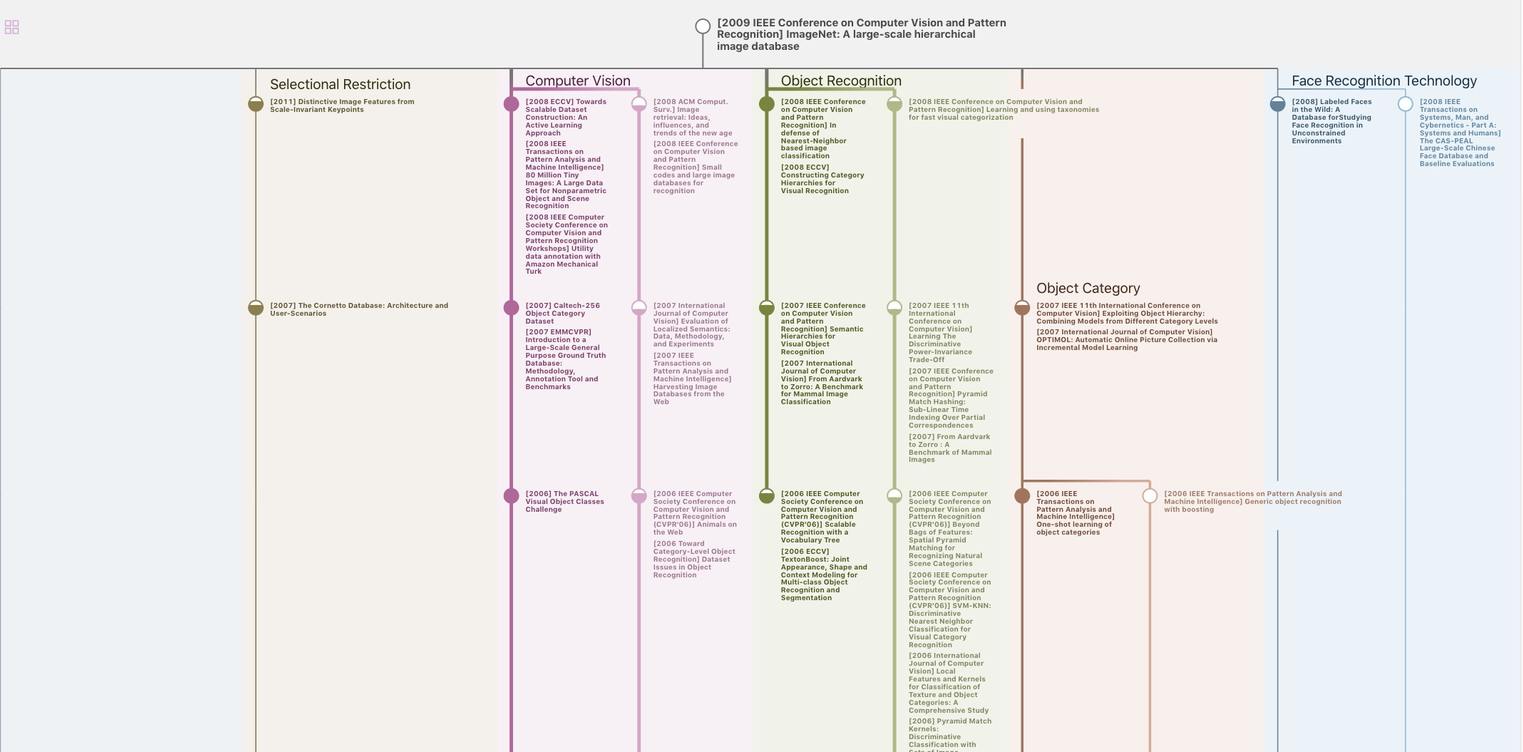
生成溯源树,研究论文发展脉络
Chat Paper
正在生成论文摘要