Replicating and Scaling up Qualitative Analysis using Crowdsourcing: A Github-based Case Study
arXiv (Cornell University)(2017)
摘要
Due to the difficulties in replicating and scaling up qualitative studies, such studies are rarely verified. Accordingly, in this paper, we leverage the advantages of crowdsourcing (low costs, fast speed, scalable workforce) to replicate and scale-up one state-of-the-art qualitative study. That qualitative study explored 20 GitHub pull requests to learn factors that influence the fate of pull requests with respect to approval and merging. As a secondary study, using crowdsourcing at a cost of 200, we studied 250 pull requests from 142 GitHub projects. The prior qualitative findings are mapped into questions for crowds workers. Their answers were converted into binary features to build a predictor which predicts whether code would be merged with median F1 scores of 68 the median F1 scores could achieve 90 features defined by prior quantitative results. Based on this case study, we conclude that there is much benefit in combining different kinds of research methods. While qualitative insights are very useful for finding novel insights, they can be hard to scale or replicate. That said, they can guide and define the goals of scalable secondary studies that use (e.g.) crowdsourcing+data mining. On the other hand, while data mining methods are reproducible and scalable to large data sets, their results may be spectacularly wrong since they lack contextual information. That said, they can be used to test the stability and external validity, of the insights gained from a qualitative analysis.
更多查看译文
关键词
crowdsourcing,qualitative analysis,scaling,case study,github-based
AI 理解论文
溯源树
样例
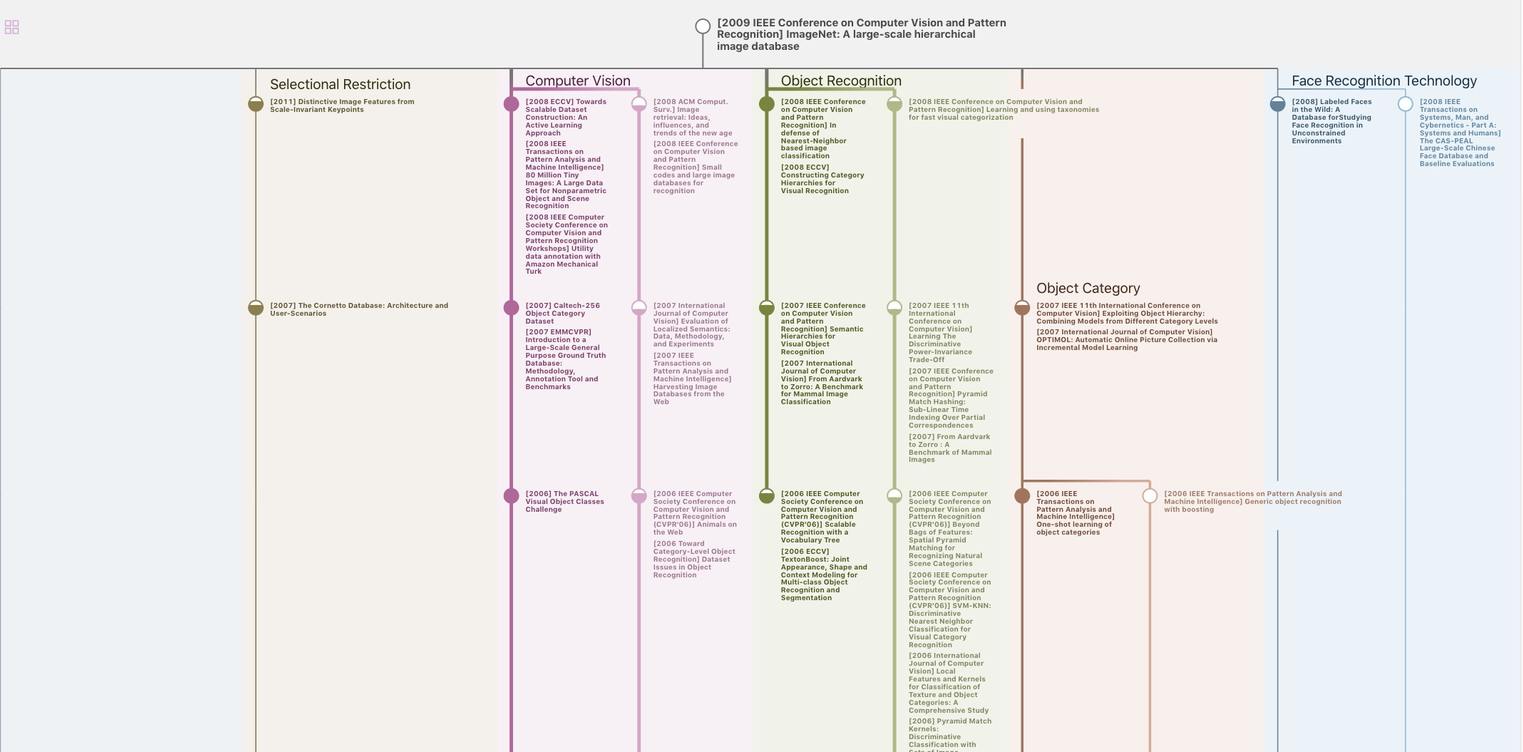
生成溯源树,研究论文发展脉络
Chat Paper
正在生成论文摘要