Markov Cross-Validation For Time Series Model Evaluations
Information Sciences(2017)
摘要
Cross-validation (CV) is a simple and universal tool to estimate generalization ability, however, existing CVs do not work well for periodicity, overlapping or correlation of series. The corresponding three criteria aimed at describing these properties are presented. Based on them, we put forward a novel Markov cross-validation (M-CV), whose data partition can be seen as a Markov process. The partition ensures that samples in each subset are neither too close nor too far. In doing so, overfitting model or information loss of series, which may result in underestimation or overestimation of the error, can be avoided. Furthermore, subsets from M-CV partition could well represent the original series, and it may be extended to time series or stream data sampling. Theoretical analysis shows that M-CV is the unique one which meets all of above criteria among current CVs. In addition, the error estimation on subsets is proved to have less variance than that on original series, therefore it ensures the stability of M-CV. Experimental results demonstrate that the proposed M-CV has lower bias, variance and time consumption than other CVs. (C) 2016 Elsevier Inc. All rights reserved.
更多查看译文
关键词
Model evaluation,Markov cross-validation,Time series
AI 理解论文
溯源树
样例
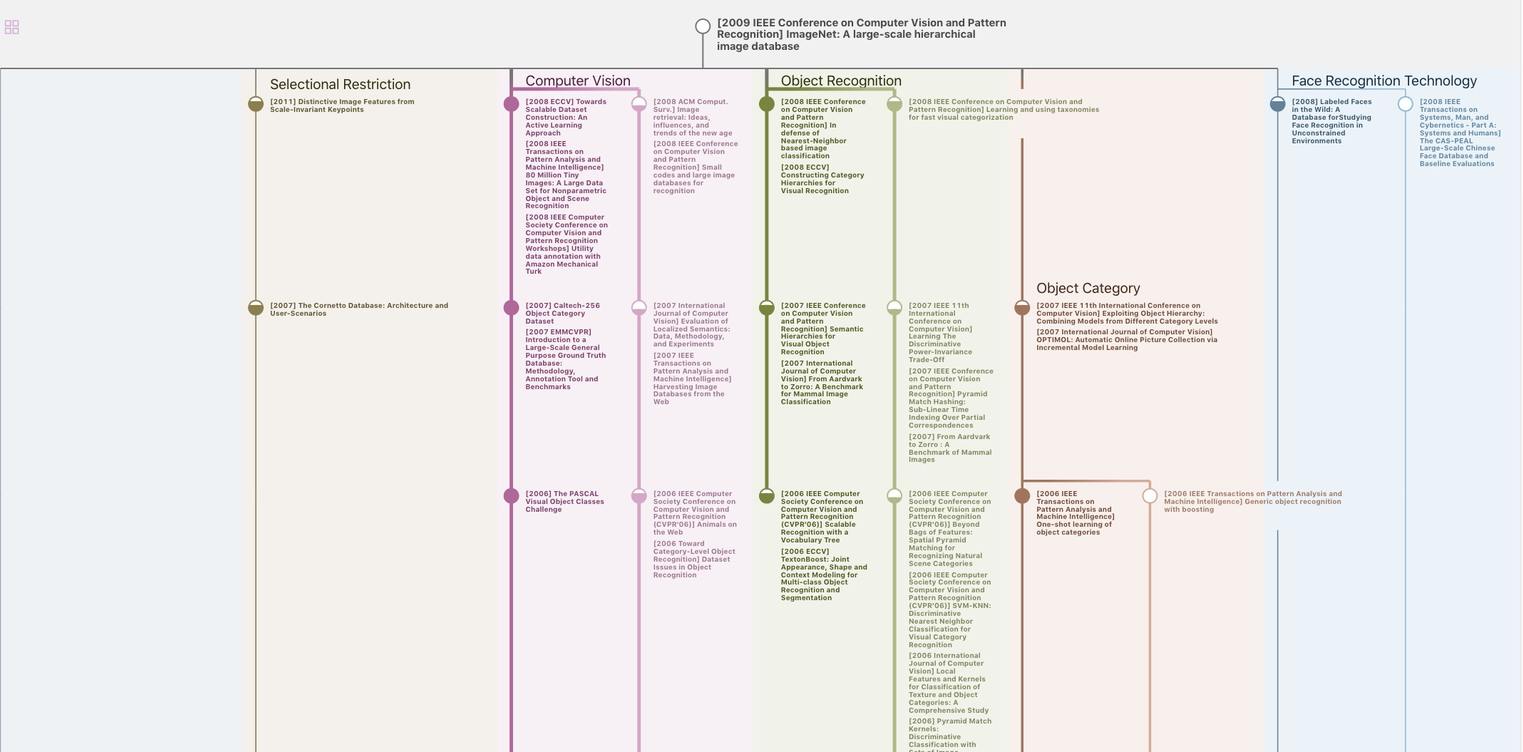
生成溯源树,研究论文发展脉络
Chat Paper
正在生成论文摘要