Joint Image-Text Representation by Gaussian Visual-Semantic Embedding.
MM(2016)
摘要
ABSTRACTHow to jointly represent images and texts is important for tasks involving both modalities. Visual-semantic embedding models have been recently proposed and shown to be effective. The key idea is that by learning a mapping from images into a semantic text space, the algorithm is able to learn a compact and effective joint representation. However, existing approaches simply map each text concept to a single point in the semantic space. Mapping instead to a density distribution provides many interesting advantages, including better capturing uncertainty about each text concept, and enabling better geometric interpretation of concepts such as inclusion, intersection, etc. In this work, we present a novel Gaussian Visual-Semantic Embedding (GVSE) model, which leverages the visual information to model text concepts as Gaussian distributions in semantic space. Experiments in two tasks, image classification and text-based image retrieval on the large scale MIT Places205 dataset, have demonstrated the superiority of our method over existing approaches, with higher accuracy and better robustness.
更多查看译文
AI 理解论文
溯源树
样例
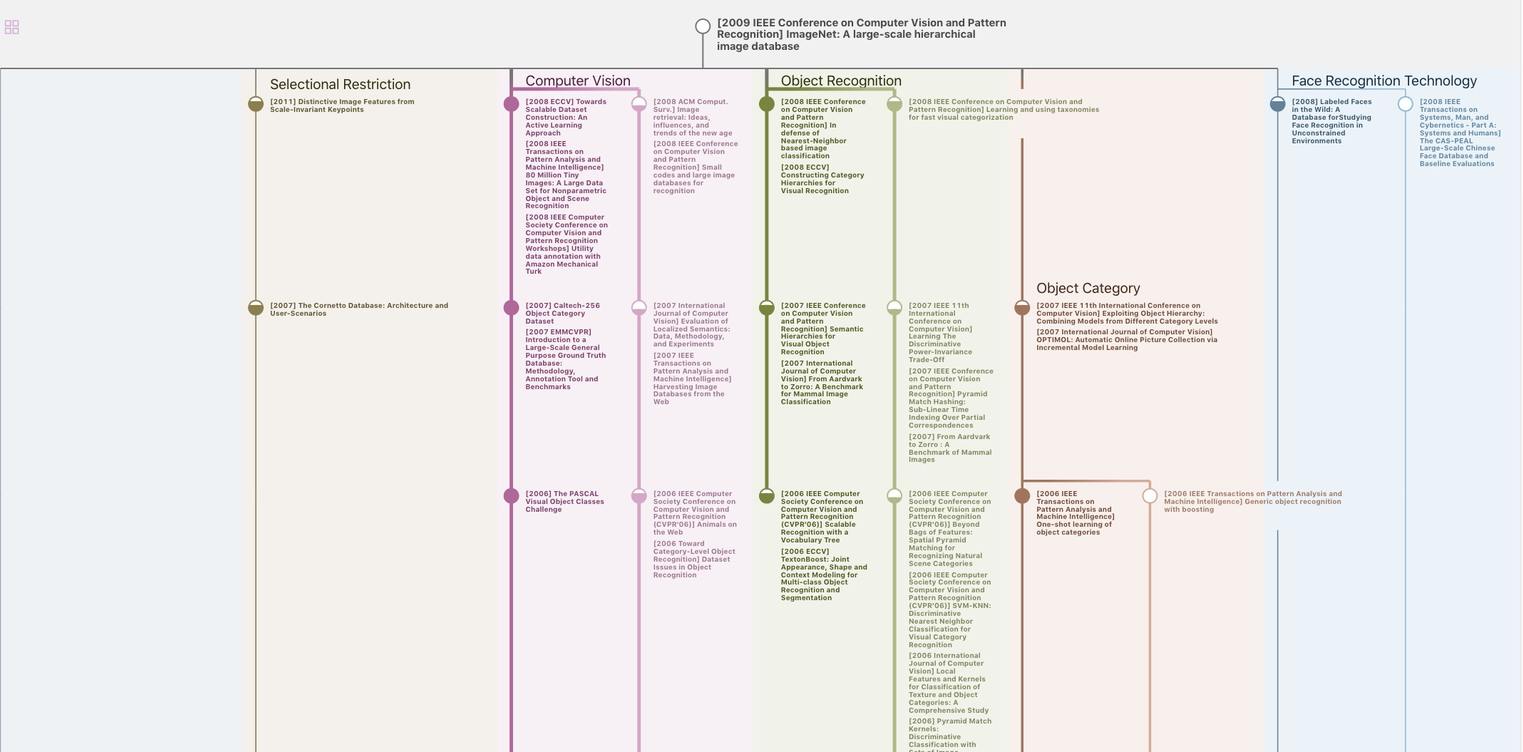
生成溯源树,研究论文发展脉络
Chat Paper
正在生成论文摘要