Data-driven techniques for smoothing histograms of local binary patterns.
Pattern Recognition(2016)
摘要
Local binary pattern histograms have proved very successful texture descriptors. Despite this success, the description procedure bears some drawbacks that are still lacking solutions in the literature. One of the problems arises when the number of extractable local patterns reduces while their dimension increases rendering the output histogram descriptions sparse and unstable, finally showing up as a reduced recognition rate. A smoothing method based on kernel density estimation was recently proposed as a means to tackle the aforementioned problem. A constituent part of the method is to determine how much to smooth a histogram. Previously, this was solved via trial-and-error in a problem-specific manner. In this paper, the goal is to present data-driven methods to determine this smoothing automatically. In the end, we present unsupervised and supervised methods for the given task and validate their performance with a representative set of local binary pattern variants in texture analysis problems covering material categorization and face recognition. HighlightsThis paper proposes data-driven techniques for smoothing LBP histograms.The proposed smoothing techniques cover unsupervised and supervised variants.The techniques are evaluated on material categorization and face recognition.Histogram smoothing is beneficial especially in small-sample-size scenarios.
更多查看译文
关键词
Local binary patterns,Local binarized descriptors,Histogram,Soft-assignment,Kernel density estimation,Histogram smoothing
AI 理解论文
溯源树
样例
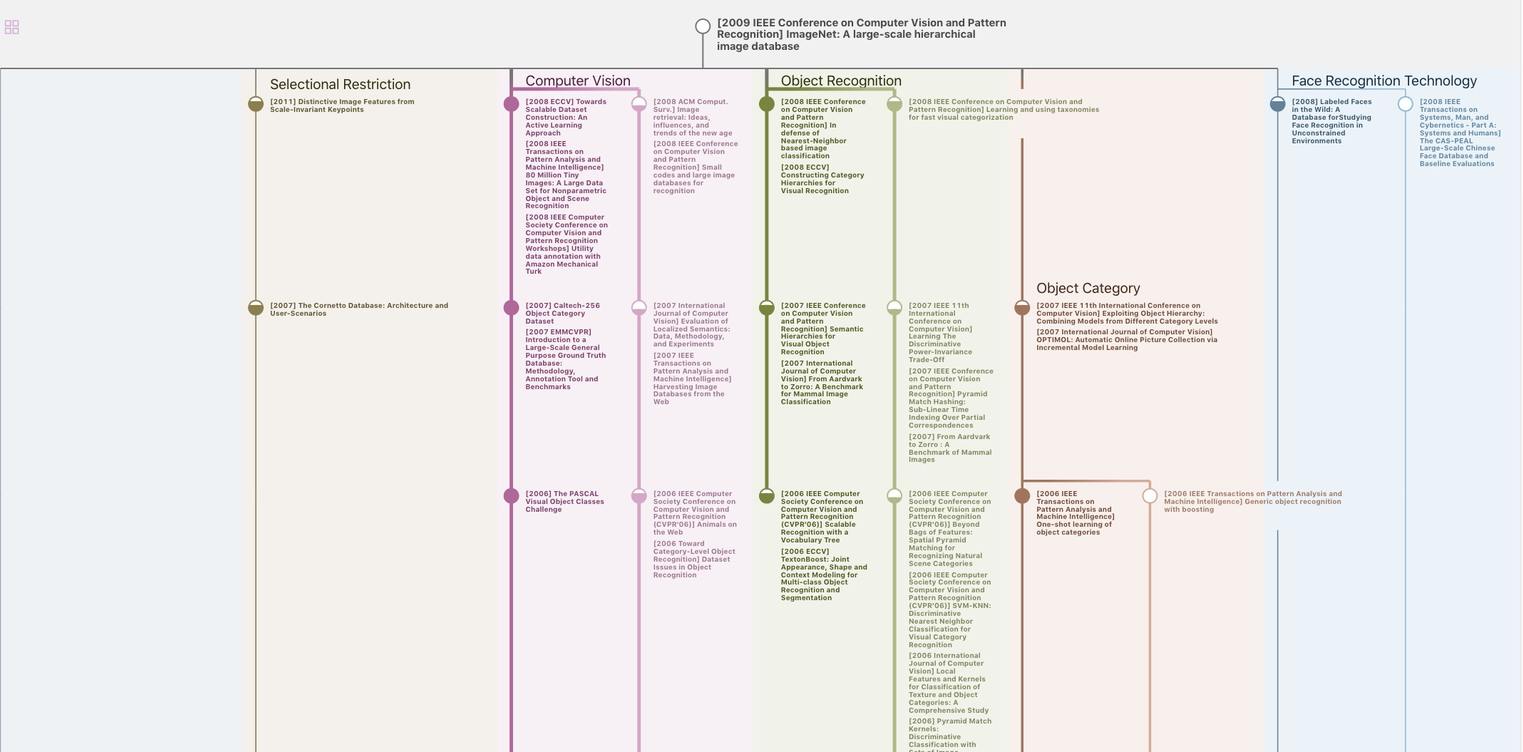
生成溯源树,研究论文发展脉络
Chat Paper
正在生成论文摘要