An Optimization Model for Clustering Categorical Data Streams with Drifting Concepts.
IEEE Trans. Knowl. Data Eng.(2016)
摘要
There is always a lack of a cluster validity function and optimization strategy to find out clusters and catch the evolution trend of cluster structures on a categorical data stream. Therefore, this paper presents an optimization model for clustering categorical data streams. In the model, a cluster validity function is proposed as the objective function to evaluate the effectiveness of the clustering model while each new input data subset is flowing. It simultaneously considers the certainty of the clustering model and the continuity with the last clustering model in the clustering process. An iterative optimization algorithm is proposed to solve an optimal solution of the objective function with some constraints. Furthermore, we strictly derive a detection index for drifting concepts from the optimization model. We propose a detection method that integrates the detection index and the optimization model to catch the evolution trend of cluster structures on a categorical data stream. The new method can effectively avoid ignoring the effect of the clustering validity on the detection result. Finally, using the experimental studies on several real data sets, we illustrate the effectiveness of the proposed algorithm in clustering categorical data streams, compared with existing data-streams clustering algorithms.
更多查看译文
关键词
Optimization,Data models,Clustering algorithms,Linear programming,Indexes,Algorithm design and analysis,Market research
AI 理解论文
溯源树
样例
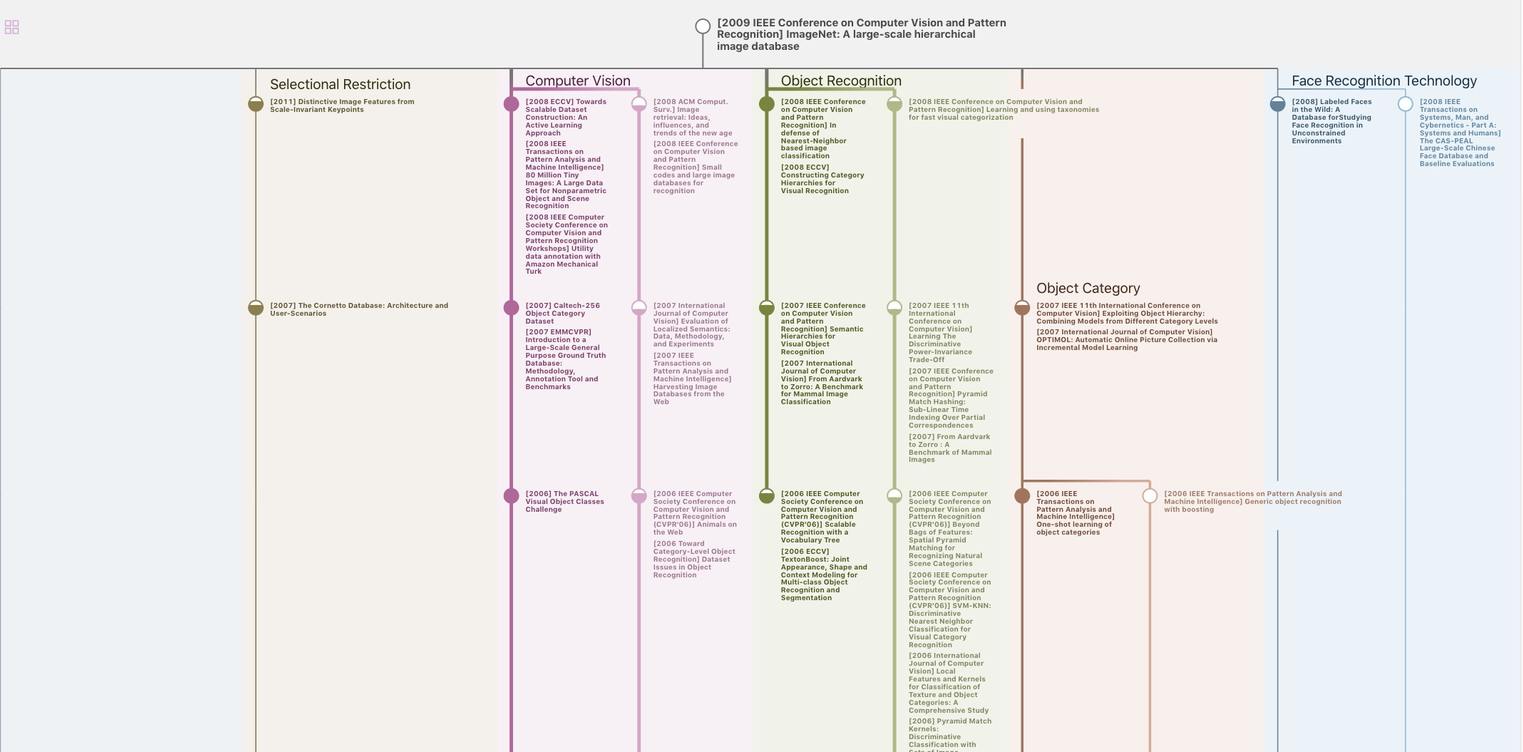
生成溯源树,研究论文发展脉络
Chat Paper
正在生成论文摘要