Human-inspired modulation frequency features for noise-robust ASR.
Speech Communication(2016)
摘要
We investigate whether the configuration of an auditory model that is optimal for predicting the intelligibility of speech under several adverse conditions is also optimal as a frontend for an automatic speech recognition system. We found that the answer is negative. The frequency resolution in the modulation filterbank that is optimal for predicting global intelligibility is too coarse for effective automatic speech recognition.We also found that detailed resolution of the modulation frequencies at the low end of the spectrum becomes more important as the signal-to-noise ratio decreases. Quite unexpectedly, the modulation frequency spectrum of car noise and train station noise appeared to be different from the spectra of the other noise type in AURORA-2.To handle the very high-dimensional and redundant feature vectors, we used a sparse coding approach for estimating the posterior probabilities of the subword units. As in a previous system that used sparse coding, we found that noise robustness in the lowest SNR conditions is improved relative to systems based on GMMs, but at the cost of slightly lower performance in the highest SNR conditions. We discuss the impact of the distance measures used in the sparse coding engine. We suggest several ways in which recognition accuracy can be improved, guided by knowledge about human speech processing.We briefly point out possible connections between combining an auditory model as a frontend and an exemplar-based procedure for estimating posterior probabilities with recent findings in brain research.The eventual aim of our research is building a model of speech recognition that is as robust to noise as humans are, using as much as possible the same processing procedures as humans do, so that the remaining recognition errors are similar to the errors that humans make. This paper investigates a computational model that combines a frontend based on an auditory model with an exemplar-based sparse coding procedure for estimating the posterior probabilities of sub-word units when processing noisified speech. Envelope modulation spectrogram (EMS) features are extracted using an auditory model which decomposes the envelopes of the outputs of a bank of gammatone filters into one lowpass and multiple bandpass components. Through a systematic analysis of the configuration of the modulation filterbank, we investigate how and why different configurations affect the posterior probabilities of sub-word units by measuring the recognition accuracy on a semantics-free speech recognition task. Our main finding is that representing speech signal dynamics by means of multiple bandpass filters typically improves recognition accuracy. This effect is particularly noticeable in very noisy conditions. In addition we find that to have maximum noise robustness, the bandpass filters should focus on low modulation frequencies. This reenforces our intuition that noise robustness can be increased by exploiting redundancy in those frequency channels which have long enough integration time not to suffer from envelope modulations that are solely due to noise. The ASR system we design based on these findings behaves more similar to human recognition of noisified digit strings than conventional ASR systems. Thanks to the relation between the modulation filterbank and procedures for computing dynamic acoustic features in conventional ASR systems, the finding can be used for improving the frontends in those systems.
更多查看译文
关键词
Modulation frequency,Auditory model,Noise-robust ASR
AI 理解论文
溯源树
样例
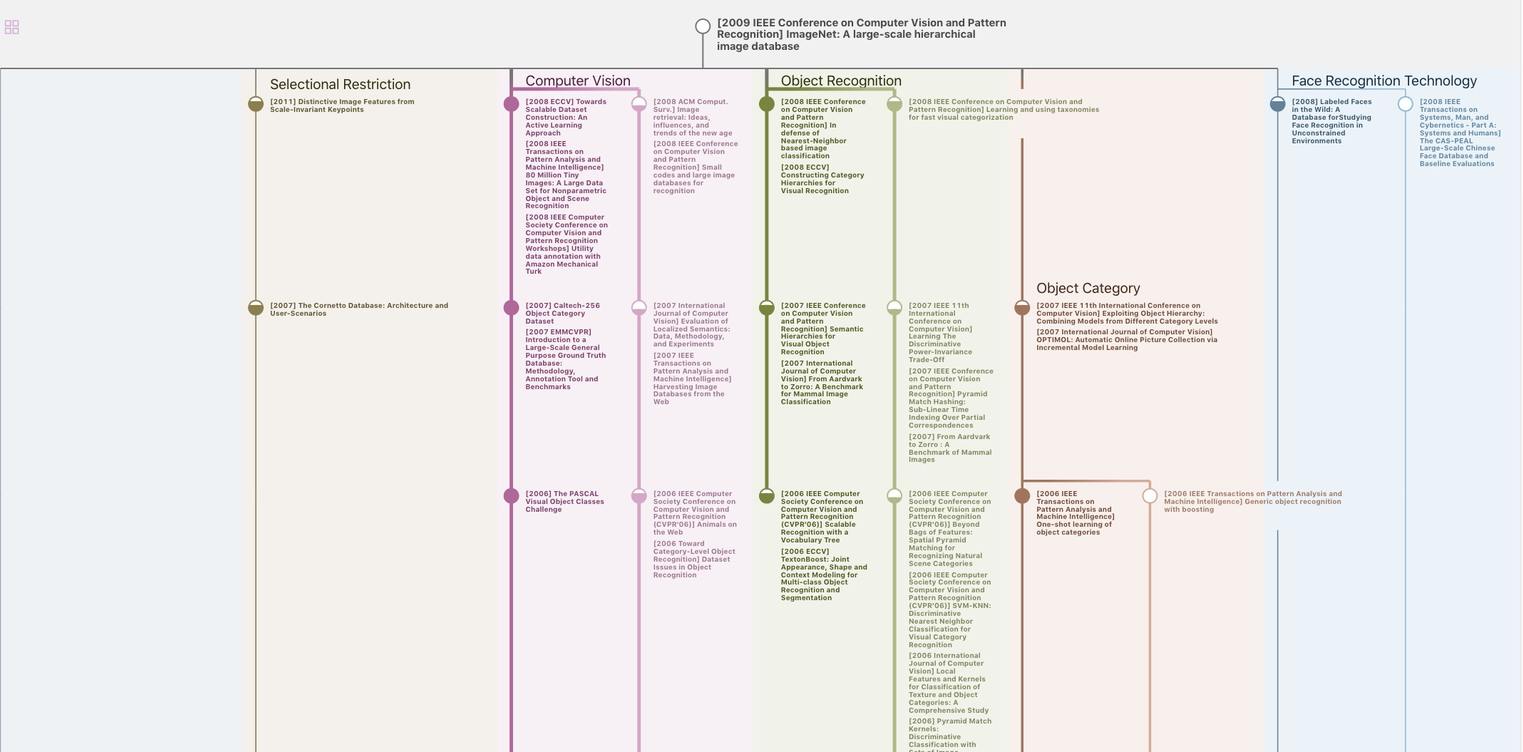
生成溯源树,研究论文发展脉络
Chat Paper
正在生成论文摘要