Enhancing and Combining Sequential and Tree LSTM for Natural Language Inference.
arXiv: Computation and Language(2016)
摘要
Reasoning and inference are central to human and artificial intelligence. Modeling inference in human language is notoriously challenging but is fundamental to natural language understanding and many applications. With the availability of large annotated data, neural network models have recently advanced the field significantly. In this paper, we present a new state-of-the-art result, achieving the accuracy of 88.3% on the standard benchmark, the Stanford Natural Language Inference dataset. This result is achieved first through our enhanced sequential encoding model, which outperforms the previous best model that employs more complicated network architectures, suggesting that the potential of sequential LSTM-based models have not been fully explored yet in previous work. We further show that by explicitly considering recursive architectures, we achieve additional improvement. Particularly, incorporating syntactic parse information contributes to our best result; it improves the performance even when the parse information is added to an already very strong system.
更多查看译文
AI 理解论文
溯源树
样例
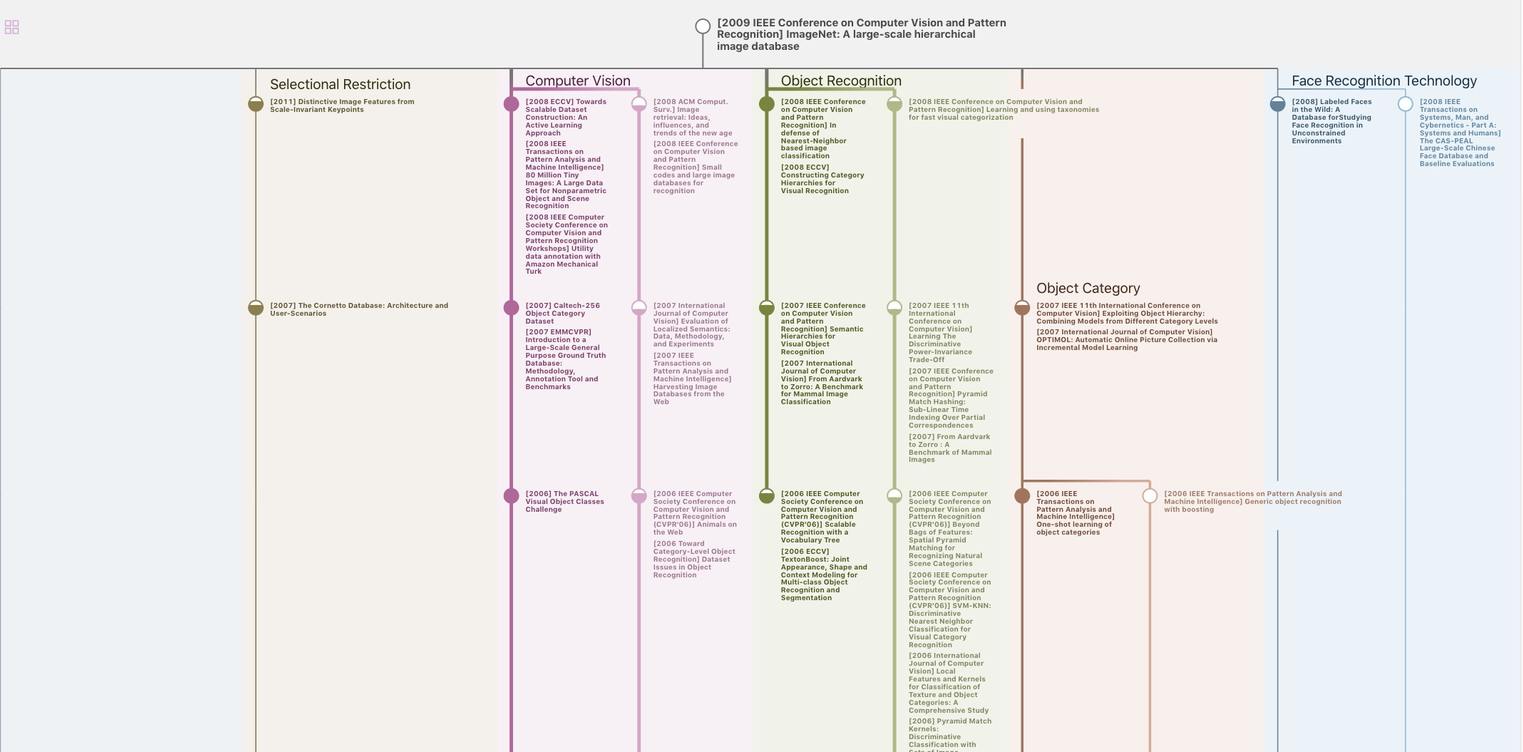
生成溯源树,研究论文发展脉络
Chat Paper
正在生成论文摘要