On Horizontal and Vertical Separation in Hierarchical Text Classification
ICTIR(2016)
摘要
Hierarchy is an effective and common way of organizing data and representing their relationships at different levels of abstraction. However, hierarchical data dependencies cause difficulties in the estimation of \"separable\" models that can distinguish between the entities in the hierarchy. Extracting separable models of hierarchical entities requires us to take their relative position into account and to consider the different types of dependencies in the hierarchy. In this paper, we present an investigation of the effect of separability in text-based entity classification and argue that in hierarchical classification, a separation property should be established between entities not only in the same layer, but also in different layers. Our main findings are the followings. First, we analyse the importance of separability on the data representation in the task of classification and based on that, we introduce \"Strong Separation Principle\" for optimizing expected effectiveness of classifiers decision based on separation property. Second, we present Significant Words Language Models (SWLM) which capture all, and only, the essential features of hierarchical entities according to their relative position in the hierarchy resulting in horizontally and vertically separable models. Third, we validate our claims on real world data and demonstrate that how SWLM improves the accuracy of classification and how it provides transferable models over time. Although discussions in this paper focus on the classification problem, the models are applicable to any information access tasks on data that has, or can be mapped to, a hierarchical structure.
更多查看译文
AI 理解论文
溯源树
样例
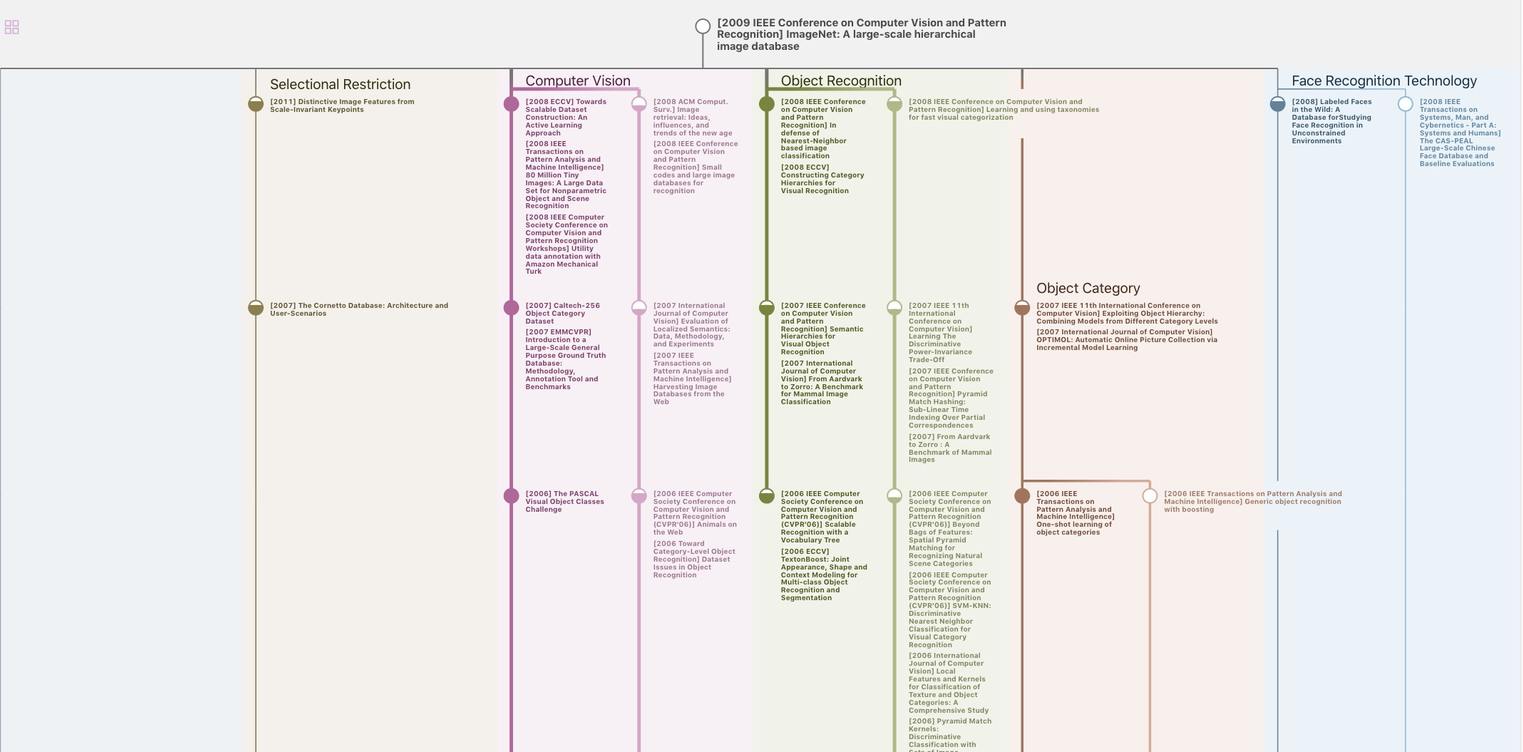
生成溯源树,研究论文发展脉络
Chat Paper
正在生成论文摘要