Sensitivity And Reliability In Incomplete Networks: Centrality Metrics To Community Scoring Functions
ASONAM '16: Advances in Social Networks Analysis and Mining 2016 Davis California August, 2016(2016)
摘要
In this paper we evaluate the effect of noise on community scoring and centrality-based parameters with respect to two different aspects of network analysis: (i) sensitivity, that is how the parameter value changes as edges are removed and (ii) reliability in the context of message spreading, that is how the time taken to broadcast a message changes as edges are removed.Our experiments on synthetic and real-world networks and three different noise models demonstrate that for both the aspects over all networks and all noise models, permanence qualifies as the most effective metric. For the sensitivity experiments closeness centrality is a close second. For the message spreading experiments, closeness and betweenness centrality based initiator selection closely competes with permanence. This is because permanence has a dual characteristic where the cumulative permanence over all vertices is sensitive to noise but the ids of the top-rank vertices, which are used to find seeds during message spreading remain relatively stable under noise.
更多查看译文
关键词
sensitivity,reliability,incomplete networks,centrality metrics,community scoring functions,centrality-based parameters,network analysis,real-world networks,noise models,top-rank vertices,cumulative permanence,dual characteristic,betweenness centrality based initiator selection,message spreading experiments,closeness centrality
AI 理解论文
溯源树
样例
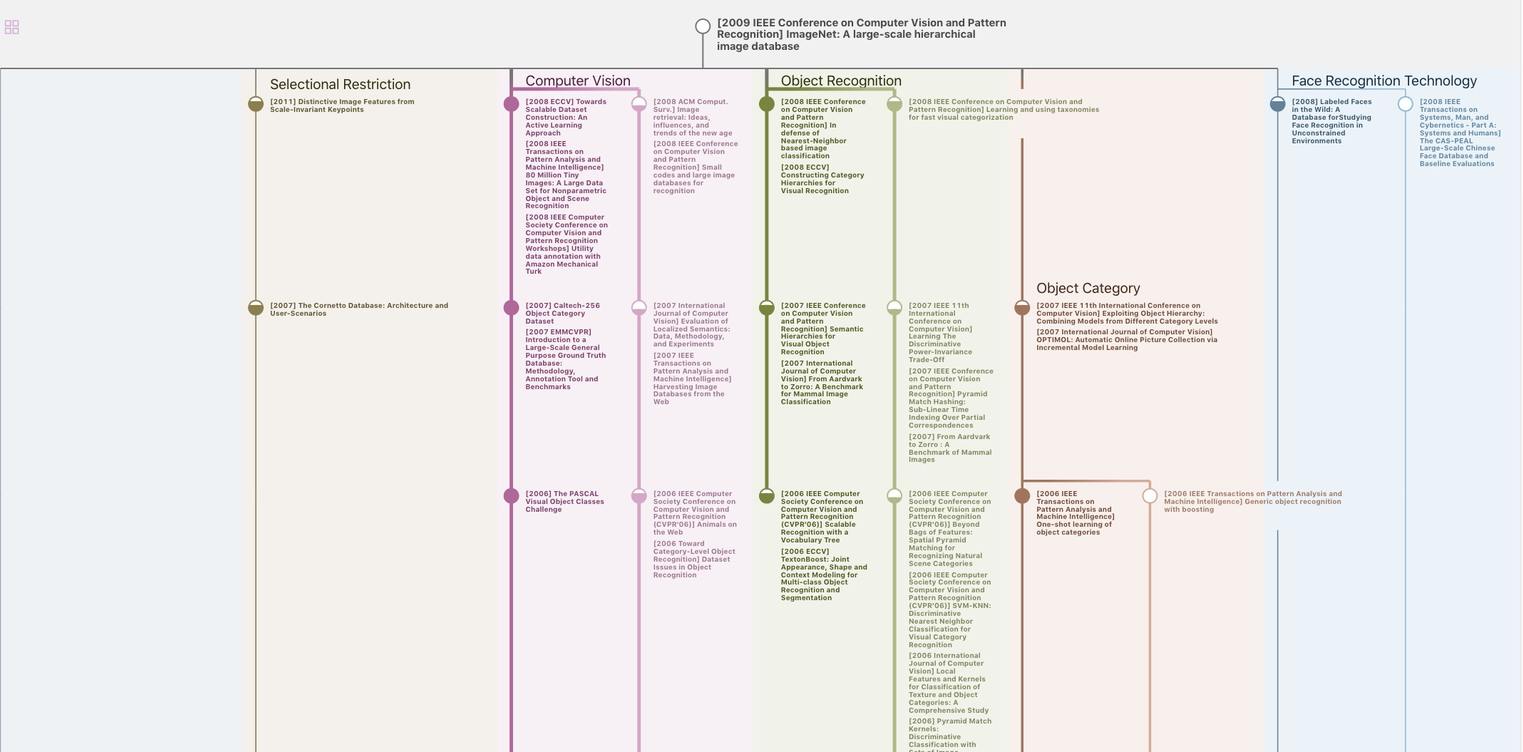
生成溯源树,研究论文发展脉络
Chat Paper
正在生成论文摘要