Characterization of coronary artery disease using flexible analytic wavelet transform applied on ECG signals.
Biomedical Signal Processing and Control(2017)
摘要
In the present work, an automated diagnosis of Coronary Artery Disease (CAD) using Electrocardiogram (ECG) signals is proposed. First, the ECG signals of 40 normal subjects and 7 CAD subjects are segmented into beats. 137,587 ECG beats of normal subjects and 44,426 ECG beats of CAD subjects are used in this work. Flexible Analytic Wavelet Transform (FAWT) technique is. used to decompose the ECG beats. Cross Information Potential (CIP) parameter is computed from the real values of detail coefficients of FAWT based decomposition. For CAD subjects mean value of CIP parameter is found higher in comparison to normal subjects. Thereafter, Student's t-test method and Kruskal-Wallis statistical test are applied to check the discrimination ability of the extracted features. Further, the features are fed to Least Squares Support Vector Machine (LS-SVM) for performing the classification. Classification accuracy is computed at every decomposition level starting from the first level of decomposition. We have observed significant improvement in the classification accuracy up to fourth level of decomposition. At fifth level of decomposition classification accuracy is not improved significantly as compared to the fourth level of decomposition. Hence, we analysed the ECG beats up to fifth level of decomposition. Accuracy of classification is higher for Morlet wavelet kernel (99.60%) in comparison to Radial Basis Function (RBF) kernel (99.56%). The developed methodology can be used in mass cardiac screening and can aid cardiologists in performing diagnosis. (C) 2016 Elsevier Ltd. All rights reserved.
更多查看译文
关键词
Coronary artery disease,Flexible analytic wavelet transform,ECG beats,Cross information potential,Student's t-test
AI 理解论文
溯源树
样例
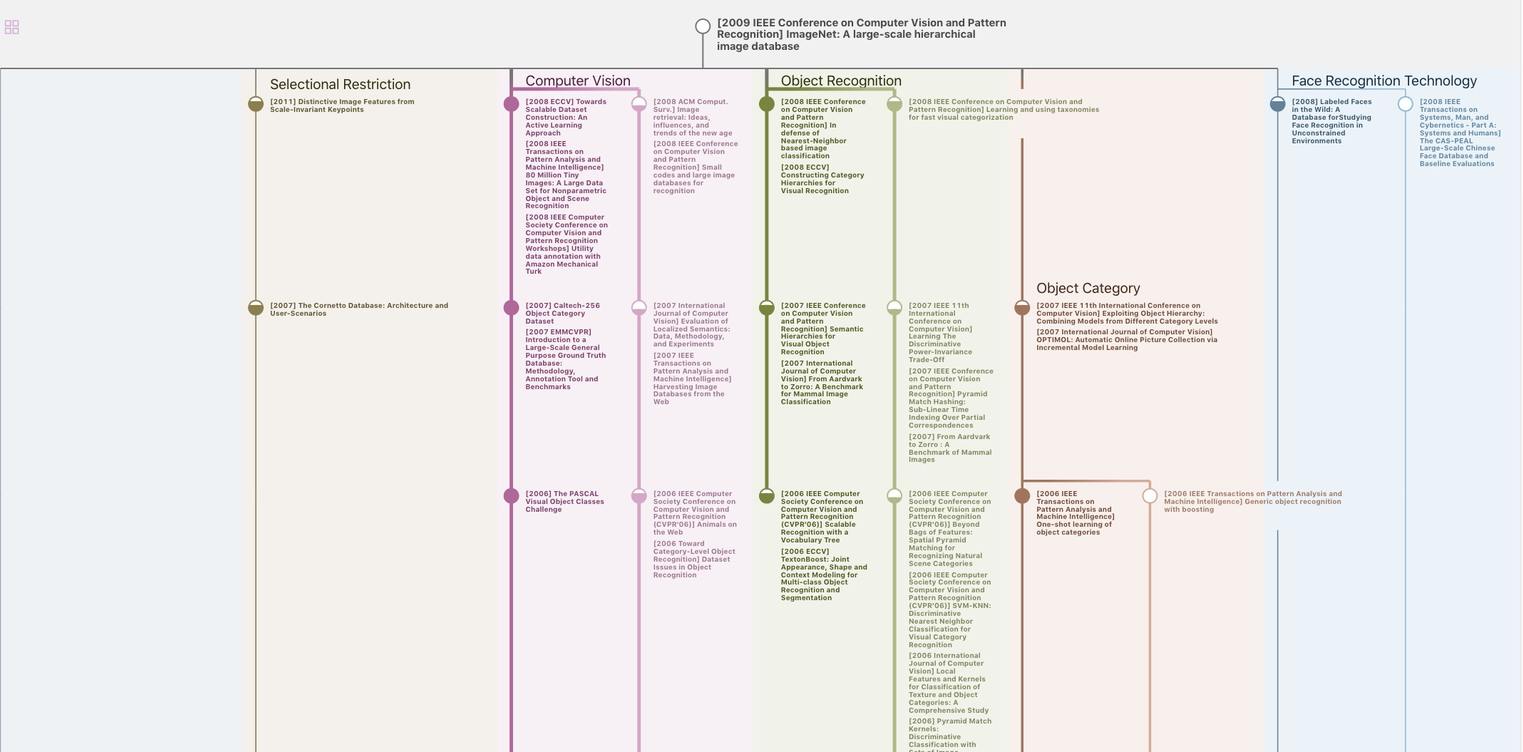
生成溯源树,研究论文发展脉络
Chat Paper
正在生成论文摘要